Fundamental constraints to the logic of living systems
- PMID:39464646
- PMCID: PMC11503024
- DOI: 10.1098/rsfs.2024.0010
Fundamental constraints to the logic of living systems
Abstract
It has been argued that the historical nature of evolution makes it a highly path-dependent process. Under this view, the outcome of evolutionary dynamics could have resulted in organisms with different forms and functions. At the same time, there is ample evidence that convergence and constraints strongly limit the domain of the potential design principles that evolution can achieve. Are these limitations relevant in shaping the fabric of the possible? Here, we argue that fundamental constraints are associated with the logic of living matter. We illustrate this idea by considering the thermodynamic properties of living systems, the linear nature of molecular information, the cellular nature of the building blocks of life, multicellularity and development, the threshold nature of computations in cognitive systems and the discrete nature of the architecture of ecosystems. In all these examples, we present available evidence and suggest potential avenues towards a well-defined theoretical formulation.
Keywords: constraints; contingency; convergence; evolution; information; thermodynamics.
© 2024 The Authors.
Conflict of interest statement
We declare we have no competing interests.
Figures
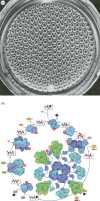
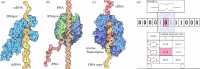
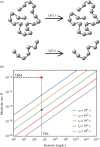
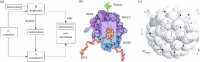
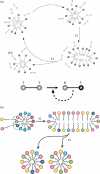
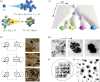
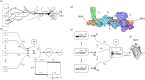
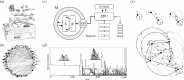
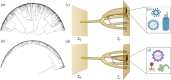
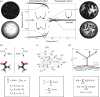
Similar articles
- Macromolecular crowding: chemistry and physics meet biology (Ascona, Switzerland, 10-14 June 2012).Foffi G, Pastore A, Piazza F, Temussi PA.Foffi G, et al.Phys Biol. 2013 Aug;10(4):040301. doi: 10.1088/1478-3975/10/4/040301. Epub 2013 Aug 2.Phys Biol. 2013.PMID:23912807
- Transformation of matter in living organisms during growth and evolution.Hansen LD, Tolley HD, Woodfield BF.Hansen LD, et al.Biophys Chem. 2021 Apr;271:106550. doi: 10.1016/j.bpc.2021.106550. Epub 2021 Jan 23.Biophys Chem. 2021.PMID:33517028Review.
- A universal model describing the structure and functions of living systems.Mistriotis A.Mistriotis A.Commun Integr Biol. 2021 Feb 24;14(1):27-36. doi: 10.1080/19420889.2021.1887549.Commun Integr Biol. 2021.PMID:33680281Free PMC article.
- Unravelling the thermodynamic properties of soil ecosystems in mature beech forests.Barros N, Popovic M, Molina-Valero J, Lestido-Cardama Y, Pérez-Cruzado C.Barros N, et al.Sci Rep. 2024 Jul 18;14(1):16644. doi: 10.1038/s41598-024-67590-w.Sci Rep. 2024.PMID:39025918Free PMC article.
- Thermodynamics and evolution.Demetrius L.Demetrius L.J Theor Biol. 2000 Sep 7;206(1):1-16. doi: 10.1006/jtbi.2000.2106.J Theor Biol. 2000.PMID:10968933Review.
References
- Summons RE, Albrecht P, McDonald G, Moldowan JM. 2008. Molecular biosignatures. In Strategies of life detection (ed. Botta O), pp. 133–159. New York, NY: Springer. (10.1007/978-0-387-77516-6_11) - DOI
Publication types
Related information
LinkOut - more resources
Full Text Sources