Salivary metabolomic biomarkers for non-invasive lung cancer detection
- PMID:38417449
- PMCID: PMC11093188
- DOI: 10.1111/cas.16112
Salivary metabolomic biomarkers for non-invasive lung cancer detection
Abstract
Identifying novel biomarkers for early detection of lung cancer is crucial. Non-invasively available saliva is an ideal biofluid for biomarker exploration; however, the rationale underlying biomarker detection from organs distal to the oral cavity in saliva requires clarification. Therefore, we analyzed metabolomic profiles of cancer tissues compared with those of adjacent non-cancerous tissues, as well as plasma and saliva samples collected from patients with lung cancer (n = 109 pairs). Additionally, we analyzed plasma and saliva samples collected from control participants (n = 83 and 71, respectively). Capillary electrophoresis-mass spectrometry and liquid chromatography-mass spectrometry were performed to comprehensively quantify hydrophilic metabolites. Paired tissues were compared, revealing 53 significantly different metabolites. Plasma and saliva showed 44 and 40 significantly different metabolites, respectively, between patients and controls. Of these, 12 metabolites exhibited significant differences in all three comparisons and primarily belonged to the polyamine and amino acid pathways; N1-acetylspermidine exhibited the highest discrimination ability. A combination of 12 salivary metabolites was evaluated using a machine learning method to differentiate patients with lung cancer from controls. Salivary data were randomly split into training and validation datasets. Areas under the receiver operating characteristic curve were 0.744 for cross-validation using training data and 0.792 for validation data. This model exhibited a higher discrimination ability for N1-acetylspermidine than that for other metabolites. The probability of lung cancer calculated using this model was independent of most patient characteristics. These results suggest that consistently different salivary biomarkers in both plasma and lung tissues might facilitate non-invasive lung cancer screening.
Keywords: biomarker; lung cancer; metabolomics; plasma; saliva.
© 2024 The Authors. Cancer Science published by John Wiley & Sons Australia, Ltd on behalf of Japanese Cancer Association.
Conflict of interest statement
Masahiro Sugimoto is a board member of SalivaTech and serves as a consultant to Human Metabolome Technologies. The other authors have no conflict of interest. All authors had full access to all the data in the study and had final responsibility for the decision to submit for publication.
Figures
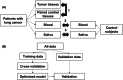
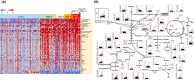
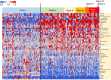
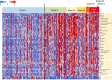
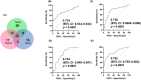
Similar articles
- Salivary metabolomic biomarkers for esophageal and gastric cancers by liquid chromatography-mass spectrometry.Nakane K, Yagi K, Yajima S, Nomura S, Sugimoto M, Seto Y.Nakane K, et al.Cancer Sci. 2024 Sep;115(9):3089-3098. doi: 10.1111/cas.16256. Epub 2024 Jul 14.Cancer Sci. 2024.PMID:39004809Free PMC article.
- Metabolomic analysis of the saliva of Japanese patients with oral squamous cell carcinoma.Ohshima M, Sugahara K, Kasahara K, Katakura A.Ohshima M, et al.Oncol Rep. 2017 May;37(5):2727-2734. doi: 10.3892/or.2017.5561. Epub 2017 Apr 6.Oncol Rep. 2017.PMID:28393236
- Salivary metabolomics with alternative decision tree-based machine learning methods for breast cancer discrimination.Murata T, Yanagisawa T, Kurihara T, Kaneko M, Ota S, Enomoto A, Tomita M, Sugimoto M, Sunamura M, Hayashida T, Kitagawa Y, Jinno H.Murata T, et al.Breast Cancer Res Treat. 2019 Oct;177(3):591-601. doi: 10.1007/s10549-019-05330-9. Epub 2019 Jul 8.Breast Cancer Res Treat. 2019.PMID:31286302
- Salivary metabolomics for cancer detection.Sugimoto M.Sugimoto M.Expert Rev Proteomics. 2020 Sep;17(9):639-648. doi: 10.1080/14789450.2020.1846524. Epub 2020 Nov 12.Expert Rev Proteomics. 2020.PMID:33140667Review.
- Role of Salivary Biomarkers in Oral Cancer Detection.Khurshid Z, Zafar MS, Khan RS, Najeeb S, Slowey PD, Rehman IU.Khurshid Z, et al.Adv Clin Chem. 2018;86:23-70. doi: 10.1016/bs.acc.2018.05.002. Epub 2018 Jul 23.Adv Clin Chem. 2018.PMID:30144841Review.
Cited by
- Metabolomics reveals early pregnancy biomarkers in sows: a non-invasive diagnostic approach.Ren Y, Zhang Q, He F, Qi M, Fu B, Zhang H, Huang T.Ren Y, et al.Front Vet Sci. 2024 Apr 25;11:1396492. doi: 10.3389/fvets.2024.1396492. eCollection 2024.Front Vet Sci. 2024.PMID:38725582Free PMC article.
- Stage-Specific Plasma Metabolomic Profiles in Colorectal Cancer.Ishizaki T, Sugimoto M, Kuboyama Y, Mazaki J, Kasahara K, Tago T, Udo R, Iwasaki K, Hayashi Y, Nagakawa Y.Ishizaki T, et al.J Clin Med. 2024 Sep 2;13(17):5202. doi: 10.3390/jcm13175202.J Clin Med. 2024.PMID:39274416Free PMC article.
References
MeSH terms
Related information
Grants and funding
LinkOut - more resources
Full Text Sources
Medical