This is a preprint.
Comprehensive and accurate genome analysis at scale using DRAGEN accelerated algorithms
- PMID:38260545
- PMCID: PMC10802302
- DOI: 10.1101/2024.01.02.573821
Comprehensive and accurate genome analysis at scale using DRAGEN accelerated algorithms
Abstract
Research and medical genomics require comprehensive and scalable solutions to drive the discovery of novel disease targets, evolutionary drivers, and genetic markers with clinical significance. This necessitates a framework to identify all types of variants independent of their size (e.g., SNV/SV) or location (e.g., repeats). Here we present DRAGEN that utilizes novel methods based on multigenomes, hardware acceleration, and machine learning based variant detection to provide novel insights into individual genomes with ~30min computation time (from raw reads to variant detection). DRAGEN outperforms all other state-of-the-art methods in speed and accuracy across all variant types (SNV, indel, STR, SV, CNV) and further incorporates specialized methods to obtain key insights in medically relevant genes (e.g., HLA, SMN, GBA). We showcase DRAGEN across 3,202 genomes and demonstrate its scalability, accuracy, and innovations to further advance the integration of comprehensive genomics for research and medical applications.
Conflict of interest statement
Competing interests FJS receives research support from Genentech, Illumina, PacBio and Oxford Nanopore. SC, MR, ST, ZH, MR, AV, GP, CR, VO, SM, JH and RM are employees of Illumina.
Figures
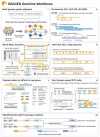
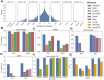
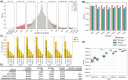
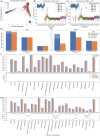
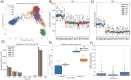
Similar articles
- Comprehensive genome analysis and variant detection at scale using DRAGEN.Behera S, Catreux S, Rossi M, Truong S, Huang Z, Ruehle M, Visvanath A, Parnaby G, Roddey C, Onuchic V, Finocchio A, Cameron DL, English A, Mehtalia S, Han J, Mehio R, Sedlazeck FJ.Behera S, et al.Nat Biotechnol. 2024 Oct 25. doi: 10.1038/s41587-024-02382-1. Online ahead of print.Nat Biotechnol. 2024.PMID:39455800
- VISTA: an integrated framework for structural variant discovery.Sarwal V, Lee S, Yang J, Sankararaman S, Chaisson M, Eskin E, Mangul S.Sarwal V, et al.Brief Bioinform. 2024 Jul 25;25(5):bbae462. doi: 10.1093/bib/bbae462.Brief Bioinform. 2024.PMID:39297879Free PMC article.
- Accuracy and efficiency of germline variant calling pipelines for human genome data.Zhao S, Agafonov O, Azab A, Stokowy T, Hovig E.Zhao S, et al.Sci Rep. 2020 Nov 19;10(1):20222. doi: 10.1038/s41598-020-77218-4.Sci Rep. 2020.PMID:33214604Free PMC article.
- From molecules to genomic variations: Accelerating genome analysis via intelligent algorithms and architectures.Alser M, Lindegger J, Firtina C, Almadhoun N, Mao H, Singh G, Gomez-Luna J, Mutlu O.Alser M, et al.Comput Struct Biotechnol J. 2022 Aug 18;20:4579-4599. doi: 10.1016/j.csbj.2022.08.019. eCollection 2022.Comput Struct Biotechnol J. 2022.PMID:36090814Free PMC article.Review.
- The Global Genome Question: Microbes as the Key to Understanding Evolution and Ecology: This report is based on a colloquium, “The Global Genome Question: Microbes as the Key to Understanding Evolution and Ecology,” sponsored by the American Academy of Microbiology and held October 11-13, 2002, in Longboat Key, Florida.[No authors listed][No authors listed]Washington (DC): American Society for Microbiology; 2004.Washington (DC): American Society for Microbiology; 2004.PMID:33119236Free Books & Documents.Review.
References
- Tarailo-Graovac M., Wasserman W. W. & Van Karnebeek C. D. M. Impact of next-generation sequencing on diagnosis and management of neurometabolic disorders: current advances and future perspectives. Expert Rev. Mol. Diagn. 17, 307–309 (2017). - PubMed
Publication types
Related information
Grants and funding
LinkOut - more resources
Full Text Sources
Research Materials