Bayesian models for spatial count data with informative finite populations with application to the American community survey
- PMID:37720247
- PMCID: PMC10503459
- DOI: 10.1080/02664763.2022.2078289
Bayesian models for spatial count data with informative finite populations with application to the American community survey
Abstract
The American Community Survey (ACS) is an ongoing program conducted by the US Census Bureau that publishes estimates of important demographic statistics over pre-specified administrative areas. ACS provides spatially referenced count-valued outcomes that are paired with finite populations. For example, the number of people below the poverty line and the total population for each county are estimated by ACS. One common assumption is that the spatially referenced count-valued outcome given the finite population is binomial distributed. This conditionally specified (CS) model does not define the joint relationship between the count-valued outcome and the finite population. Thus, we consider a joint model for the count-valued outcome and the finite population. When cross-dependence in our joint model can be leveraged to 'improve spatial prediction' we say that the finite population is 'informative.' We model the count given the finite population as binomial and the finite population as negative binomial and use multivariate logit-beta prior distributions. This leads to closed-form expressions of the full-conditional distributions for an efficient Gibbs sampler. We illustrate our model through simulations and our motivating application of ACS poverty estimates. These empirical analyses show the benefits of using our proposed model over the more traditional CS binomial model.
Keywords: Bayesian statistics; conjugate; generalized linear models; official statistics; spatial statistics.
© 2022 Informa UK Limited, trading as Taylor & Francis Group.
Conflict of interest statement
No potential conflict of interest was reported by the author(s).
Figures
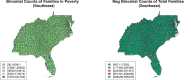
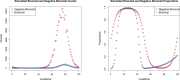
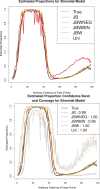
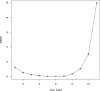
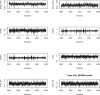
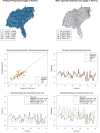
Similar articles
- A BAYESIAN HIERARCHICAL SMALL AREA POPULATION MODEL ACCOUNTING FOR DATA SOURCE SPECIFIC METHODOLOGIES FROM AMERICAN COMMUNITY SURVEY, POPULATION ESTIMATES PROGRAM, AND DECENNIAL CENSUS DATA.Peterson EN, Nethery RC, Padellini T, Chen JT, Coull BA, Piel FB, Wakefield J, Blangiardo M, Waller LA.Peterson EN, et al.Ann Appl Stat. 2024 Jun;18(2):1565-1595. doi: 10.1214/23-aoas1849. Epub 2024 Apr 5.Ann Appl Stat. 2024.PMID:39323985Free PMC article.
- Folic acid supplementation and malaria susceptibility and severity among people taking antifolate antimalarial drugs in endemic areas.Crider K, Williams J, Qi YP, Gutman J, Yeung L, Mai C, Finkelstain J, Mehta S, Pons-Duran C, Menéndez C, Moraleda C, Rogers L, Daniels K, Green P.Crider K, et al.Cochrane Database Syst Rev. 2022 Feb 1;2(2022):CD014217. doi: 10.1002/14651858.CD014217.Cochrane Database Syst Rev. 2022.PMID:36321557Free PMC article.
- [Meta-analysis of the Italian studies on short-term effects of air pollution].Biggeri A, Bellini P, Terracini B; Italian MISA Group.Biggeri A, et al.Epidemiol Prev. 2001 Mar-Apr;25(2 Suppl):1-71.Epidemiol Prev. 2001.PMID:11515188Italian.
- Bayesian negative binomial regression with spatially varying dispersion: Modeling COVID-19 incidence in Georgia.Mutiso F, Pearce JL, Benjamin-Neelon SE, Mueller NT, Li H, Neelon B.Mutiso F, et al.Spat Stat. 2022 Dec;52:100703. doi: 10.1016/j.spasta.2022.100703. Epub 2022 Sep 23.Spat Stat. 2022.PMID:36168515Free PMC article.Review.
- Sutureless Aortic Valve Replacement for Treatment of Severe Aortic Stenosis: A Single Technology Assessment of Perceval Sutureless Aortic Valve [Internet].Desser AS, Arentz-Hansen H, Fagerlund BF, Harboe I, Lauvrak V.Desser AS, et al.Oslo, Norway: Knowledge Centre for the Health Services at The Norwegian Institute of Public Health (NIPH); 2017 Aug 25. Report from the Norwegian Institute of Public Health No. 2017-01.Oslo, Norway: Knowledge Centre for the Health Services at The Norwegian Institute of Public Health (NIPH); 2017 Aug 25. Report from the Norwegian Institute of Public Health No. 2017-01.PMID:29553663Free Books & Documents.Review.
References
- Ahlburg D.A., Population growth and poverty, in The Impact of Population Growth on Well-Being in Developing Countries, D.A. Ahlburg, A. C. Kelley, and K. O. Mason eds., Berlin-Heidelberg: Springer, 1996, pp. 219–258.
- Bradley J., Wikle C., and Holan S., Spatio-temporal change of support with application to American community survey multi-year period estimates, Statistics 4 (2015), pp. 255–270.
- Bradley J.R., Wikle C.K., and Holan S.H., Bayesian spatial change of support for count-valued survey data with application to the American community survey, J. Am. Stat. Assoc. 111 (2016), pp. 472–487.
- Bradley J.R., Wikle C.K., and Holan S.H., Spatio-temporal models for big multinomial data using the conditional multivariate logit-beta distribution, J. Time Ser. Anal. 40 (2019), pp. 363–382.
- Bradley J.R., Holan S.H., and Wikle C.K., Bayesian hierarchical models with conjugate full-conditional distributions for dependent data from the natural exponential family, J. Am. Stat. Assoc. 115 (2020), pp. 2037–2052.
Grants and funding
LinkOut - more resources
Full Text Sources
Other Literature Sources