Computational Intelligence-Based Disease Severity Identification: A Review of Multidisciplinary Domains
- PMID:37046431
- PMCID: PMC10093052
- DOI: 10.3390/diagnostics13071212
Computational Intelligence-Based Disease Severity Identification: A Review of Multidisciplinary Domains
Abstract
Disease severity identification using computational intelligence-based approaches is gaining popularity nowadays. Artificial intelligence and deep-learning-assisted approaches are proving to be significant in the rapid and accurate diagnosis of several diseases. In addition to disease identification, these approaches have the potential to identify the severity of a disease. The problem of disease severity identification can be considered multi-class classification, where the class labels are the severity levels of the disease. Plenty of computational intelligence-based solutions have been presented by researchers for severity identification. This paper presents a comprehensive review of recent approaches for identifying disease severity levels using computational intelligence-based approaches. We followed the PRISMA guidelines and compiled several works related to the severity identification of multidisciplinary diseases of the last decade from well-known publishers, such as MDPI, Springer, IEEE, Elsevier, etc. This article is devoted toward the severity identification of two main diseases, viz. Parkinson's Disease and Diabetic Retinopathy. However, severity identification of a few other diseases, such as COVID-19, autonomic nervous system dysfunction, tuberculosis, sepsis, sleep apnea, psychosis, traumatic brain injury, breast cancer, knee osteoarthritis, and Alzheimer's disease, was also briefly covered. Each work has been carefully examined against its methodology, dataset used, and the type of disease on several performance metrics, accuracy, specificity, etc. In addition to this, we also presented a few public repositories that can be utilized to conduct research on disease severity identification. We hope that this review not only acts as a compendium but also provides insights to the researchers working on disease severity identification using computational intelligence-based approaches.
Keywords: Alzheimer’s disease; CNN; MSC; Parkinson’s disease; deep learning; diabetic retinopathy; disease severity; machine learning.
Conflict of interest statement
The authors declare no conflict of interest.
Figures
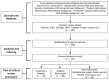

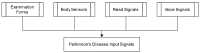
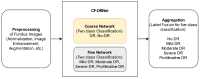

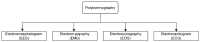
Similar articles
- Artificial Intelligence and Deep Learning Assisted Rapid Diagnosis of COVID-19 from Chest Radiographical Images: A Survey.Sinwar D, Dhaka VS, Tesfaye BA, Raghuwanshi G, Kumar A, Maakar SK, Agrawal S.Sinwar D, et al.Contrast Media Mol Imaging. 2022 Oct 12;2022:1306664. doi: 10.1155/2022/1306664. eCollection 2022.Contrast Media Mol Imaging. 2022.PMID:36304775Free PMC article.Review.
- A Systematic Review of Artificial Intelligence Applications Used for Inherited Retinal Disease Management.Esengönül M, Marta A, Beirão J, Pires IM, Cunha A.Esengönül M, et al.Medicina (Kaunas). 2022 Mar 31;58(4):504. doi: 10.3390/medicina58040504.Medicina (Kaunas). 2022.PMID:35454342Free PMC article.Review.
- Review on COVID-19 diagnosis models based on machine learning and deep learning approaches.Alyasseri ZAA, Al-Betar MA, Doush IA, Awadallah MA, Abasi AK, Makhadmeh SN, Alomari OA, Abdulkareem KH, Adam A, Damasevicius R, Mohammed MA, Zitar RA.Alyasseri ZAA, et al.Expert Syst. 2022 Mar;39(3):e12759. doi: 10.1111/exsy.12759. Epub 2021 Jul 28.Expert Syst. 2022.PMID:34511689Free PMC article.
- The mathematics of erythema: Development of machine learning models for artificial intelligence assisted measurement and severity scoring of radiation induced dermatitis.Ranjan R, Partl R, Erhart R, Kurup N, Schnidar H.Ranjan R, et al.Comput Biol Med. 2021 Dec;139:104952. doi: 10.1016/j.compbiomed.2021.104952. Epub 2021 Oct 27.Comput Biol Med. 2021.PMID:34739967
- Folic acid supplementation and malaria susceptibility and severity among people taking antifolate antimalarial drugs in endemic areas.Crider K, Williams J, Qi YP, Gutman J, Yeung L, Mai C, Finkelstain J, Mehta S, Pons-Duran C, Menéndez C, Moraleda C, Rogers L, Daniels K, Green P.Crider K, et al.Cochrane Database Syst Rev. 2022 Feb 1;2(2022):CD014217. doi: 10.1002/14651858.CD014217.Cochrane Database Syst Rev. 2022.PMID:36321557Free PMC article.
Cited by
- Liability of Health Professionals Using Sensors, Telemedicine and Artificial Intelligence for Remote Healthcare.Geny M, Andres E, Talha S, Geny B.Geny M, et al.Sensors (Basel). 2024 May 28;24(11):3491. doi: 10.3390/s24113491.Sensors (Basel). 2024.PMID:38894282Free PMC article.Review.
- Advancements in Artificial Intelligence for Medical Computer-Aided Diagnosis.Al-Antari MA.Al-Antari MA.Diagnostics (Basel). 2024 Jun 15;14(12):1265. doi: 10.3390/diagnostics14121265.Diagnostics (Basel). 2024.PMID:38928680Free PMC article.
References
- Wu D., Gong K., Arru C.D., Homayounieh F., Bizzo B., Buch V., Ren H., Kim K., Neumark N., Xu P., et al. Severity and consolidation quantification of COVID-19 from CT images using deep learning based on hybrid weak labels. IEEE J. Biomed. Health Inform. 2020;24:3529–3538. doi: 10.1109/JBHI.2020.3030224. - DOI - PMC - PubMed
- Mithra K., Emmanuel W.S. Gaussian model based hybrid technique for infection level identification in TB diagnosis. J. King Saud Univ.-Comput. Inf. Sci. 2021;33:988–998. doi: 10.1016/j.jksuci.2018.07.008. - DOI
Publication types
Related information
Grants and funding
LinkOut - more resources
Full Text Sources