Machine learning for modeling the progression of Alzheimer disease dementia using clinical data: a systematic literature review
- PMID:34350389
- PMCID: PMC8327375
- DOI: 10.1093/jamiaopen/ooab052
Machine learning for modeling the progression of Alzheimer disease dementia using clinical data: a systematic literature review
Abstract
Objective: Alzheimer disease (AD) is the most common cause of dementia, a syndrome characterized by cognitive impairment severe enough to interfere with activities of daily life. We aimed to conduct a systematic literature review (SLR) of studies that applied machine learning (ML) methods to clinical data derived from electronic health records in order to model risk for progression of AD dementia.
Materials and methods: We searched for articles published between January 1, 2010, and May 31, 2020, in PubMed, Scopus, ScienceDirect, IEEE Explore Digital Library, Association for Computing Machinery Digital Library, and arXiv. We used predefined criteria to select relevant articles and summarized them according to key components of ML analysis such as data characteristics, computational algorithms, and research focus.
Results: There has been a considerable rise over the past 5 years in the number of research papers using ML-based analysis for AD dementia modeling. We reviewed 64 relevant articles in our SLR. The results suggest that majority of existing research has focused on predicting progression of AD dementia using publicly available datasets containing both neuroimaging and clinical data (neurobehavioral status exam scores, patient demographics, neuroimaging data, and laboratory test values).
Discussion: Identifying individuals at risk for progression of AD dementia could potentially help to personalize disease management to plan future care. Clinical data consisting of both structured data tables and clinical notes can be effectively used in ML-based approaches to model risk for AD dementia progression. Data sharing and reproducibility of results can enhance the impact, adaptation, and generalizability of this research.
Keywords: Alzheimer disease; clinical data; dementia; electronic health records; machine learning.
© The Author(s) 2021. Published by Oxford University Press on behalf of the American Medical Informatics Association.
Figures
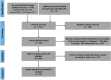
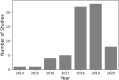
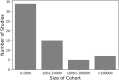
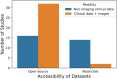
Similar articles
- Folic acid supplementation and malaria susceptibility and severity among people taking antifolate antimalarial drugs in endemic areas.Crider K, Williams J, Qi YP, Gutman J, Yeung L, Mai C, Finkelstain J, Mehta S, Pons-Duran C, Menéndez C, Moraleda C, Rogers L, Daniels K, Green P.Crider K, et al.Cochrane Database Syst Rev. 2022 Feb 1;2(2022):CD014217. doi: 10.1002/14651858.CD014217.Cochrane Database Syst Rev. 2022.PMID:36321557Free PMC article.
- Screening for Cognitive Impairment in Older Adults: An Evidence Update for the U.S. Preventive Services Task Force [Internet].Lin JS, O'Connor E, Rossom RC, Perdue LA, Burda BU, Thompson M, Eckstrom E.Lin JS, et al.Rockville (MD): Agency for Healthcare Research and Quality (US); 2013 Nov. Report No.: 14-05198-EF-1.Rockville (MD): Agency for Healthcare Research and Quality (US); 2013 Nov. Report No.: 14-05198-EF-1.PMID:24354019Free Books & Documents.Review.
- Neuroimaging and machine learning for studying the pathways from mild cognitive impairment to alzheimer's disease: a systematic review.Ahmadzadeh M, Christie GJ, Cosco TD, Arab A, Mansouri M, Wagner KR, DiPaola S, Moreno S.Ahmadzadeh M, et al.BMC Neurol. 2023 Aug 22;23(1):309. doi: 10.1186/s12883-023-03323-2.BMC Neurol. 2023.PMID:37608251Free PMC article.
- Mini-Mental State Examination (MMSE) for the detection of Alzheimer's disease and other dementias in people with mild cognitive impairment (MCI).Arevalo-Rodriguez I, Smailagic N, Roqué I Figuls M, Ciapponi A, Sanchez-Perez E, Giannakou A, Pedraza OL, Bonfill Cosp X, Cullum S.Arevalo-Rodriguez I, et al.Cochrane Database Syst Rev. 2015 Mar 5;2015(3):CD010783. doi: 10.1002/14651858.CD010783.pub2.Cochrane Database Syst Rev. 2015.Update in:Cochrane Database Syst Rev. 2021 Jul 27;7:CD010783. doi: 10.1002/14651858.CD010783.pub3.PMID:25740785Free PMC article.Updated.Review.
- Data-driven modeling and prediction of blood glucose dynamics: Machine learning applications in type 1 diabetes.Woldaregay AZ, Årsand E, Walderhaug S, Albers D, Mamykina L, Botsis T, Hartvigsen G.Woldaregay AZ, et al.Artif Intell Med. 2019 Jul;98:109-134. doi: 10.1016/j.artmed.2019.07.007. Epub 2019 Jul 26.Artif Intell Med. 2019.PMID:31383477Review.
Cited by
- Analyzing heterogeneity in Alzheimer Disease using multimodal normative modeling on imaging-based ATN biomarkers.Kumar S, Earnest T, Yang B, Kothapalli D, Aschenbrenner AJ, Hassenstab J, Xiong C, Ances B, Morris J, Benzinger TLS, Gordon BA, Payne P, Sotiras A; Alzheimer’s Disease Neuroimaging Initiative.Kumar S, et al.bioRxiv [Preprint]. 2024 Jun 30:2023.08.15.553412. doi: 10.1101/2023.08.15.553412.bioRxiv. 2024.PMID:37662280Free PMC article.Preprint.
- Machine learning models identify predictive features of patient mortality across dementia types.Zhang J, Song L, Miller Z, Chan KCG, Huang KL.Zhang J, et al.Commun Med (Lond). 2024 Feb 28;4(1):23. doi: 10.1038/s43856-024-00437-7.Commun Med (Lond). 2024.PMID:38418871Free PMC article.
- Digital cognitive stimulation in elderly breast cancer patients: the Cog-Tab-Age feasibility study.Binarelli G, Lange M, Santos MD, Duivon M, Capel A, Fernette M, Boué A, Grellard JM, Tron L, Ahmed-Lecheheb D, Clarisse B, Rigal O, Le Fel J, Joly F.Binarelli G, et al.BMC Complement Med Ther. 2024 May 31;24(1):209. doi: 10.1186/s12906-024-04507-0.BMC Complement Med Ther. 2024.PMID:38822324Free PMC article.
- Identifying Medicare beneficiaries with Alzheimer's disease and related dementia using home health OASIS assessments.Bélanger E, Rosendaal N, Gutman R, Lake D, Santostefano CM, Meyers DJ, Gozalo PL.Bélanger E, et al.J Am Geriatr Soc. 2023 Oct;71(10):3229-3236. doi: 10.1111/jgs.18487. Epub 2023 Jun 26.J Am Geriatr Soc. 2023.PMID:37358283Free PMC article.
- Predicting the Risk of Alzheimer's Disease and Related Dementia in Patients with Mild Cognitive Impairment Using a Semi-Competing Risk Approach.Chen Z, Yang Y, Zhang D, Guo J, Guo Y, Hu X, Chen Y, Bian J.Chen Z, et al.Informatics (MDPI). 2023 Jun;10(2):46. doi: 10.3390/informatics10020046. Epub 2023 May 30.Informatics (MDPI). 2023.PMID:38919750Free PMC article.
References
- Ballard C, Gauthier S, Corbett A, et al.Alzheimer's disease. Lancet 2011; 377 (9770): 1019–31. - PubMed
- Prince M, Bryce R, Albanese E, et al.The global prevalence of dementia: a systematic review and metaanalysis. Alzheimers Dement 2013; 9 (1): 63–75.e2. - PubMed
- Villemagne VL, Burnham S, Bourgeat P, et al.; Australian Imaging Biomarkers and Lifestyle (AIBL) Research Group. Amyloid beta deposition, neurodegeneration, and cognitive decline in sporadic Alzheimer's disease: a prospective cohort study. Lancet Neurol 2013; 12 (4): 357–67. - PubMed
Publication types
Related information
Grants and funding
LinkOut - more resources
Full Text Sources
Miscellaneous