Computer Vision for Brain Disorders Based Primarily on Ocular Responses
- PMID:33967931
- PMCID: PMC8096911
- DOI: 10.3389/fneur.2021.584270
Computer Vision for Brain Disorders Based Primarily on Ocular Responses
Abstract
Real-time ocular responses are tightly associated with emotional and cognitive processing within the central nervous system. Patterns seen in saccades, pupillary responses, and spontaneous blinking, as well as retinal microvasculature and morphology visualized via office-based ophthalmic imaging, are potential biomarkers for the screening and evaluation of cognitive and psychiatric disorders. In this review, we outline multiple techniques in which ocular assessments may serve as a non-invasive approach for the early detections of various brain disorders, such as autism spectrum disorder (ASD), Alzheimer's disease (AD), schizophrenia (SZ), and major depressive disorder (MDD). In addition, rapid advances in artificial intelligence (AI) present a growing opportunity to use machine learning-based AI, especially computer vision (CV) with deep-learning neural networks, to shed new light on the field of cognitive neuroscience, which is most likely to lead to novel evaluations and interventions for brain disorders. Hence, we highlight the potential of using AI to evaluate brain disorders based primarily on ocular features.
Keywords: brain disorders; cognitive neuroscience; computer vision; eye-brain engineering; ocular assessment; retina.
Copyright © 2021 Li, Fan, Chen, Li, Ning, Lin, Chen, Qin, Yeung, Li, Wang and So.
Conflict of interest statement
XL and JL were both core members at the start-up company of BIAI INC, USA/BIAI LLC., China. The remaining authors declare that the research was conducted in the absence of any commercial or financial relationships that could be construed as a potential conflict of interest.
Figures
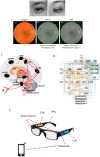
Similar articles
- Artificial intelligence in retina.Schmidt-Erfurth U, Sadeghipour A, Gerendas BS, Waldstein SM, Bogunović H.Schmidt-Erfurth U, et al.Prog Retin Eye Res. 2018 Nov;67:1-29. doi: 10.1016/j.preteyeres.2018.07.004. Epub 2018 Aug 1.Prog Retin Eye Res. 2018.PMID:30076935Review.
- Ocular biomarkers for early detection of Alzheimer's disease.Frost S, Martins RN, Kanagasingam Y.Frost S, et al.J Alzheimers Dis. 2010;22(1):1-16. doi: 10.3233/JAD-2010-100819.J Alzheimers Dis. 2010.PMID:20847434Review.
- Plasma neuronal exosomes serve as biomarkers of cognitive impairment in HIV infection and Alzheimer's disease.Pulliam L, Sun B, Mustapic M, Chawla S, Kapogiannis D.Pulliam L, et al.J Neurovirol. 2019 Oct;25(5):702-709. doi: 10.1007/s13365-018-0695-4. Epub 2019 Jan 4.J Neurovirol. 2019.PMID:30610738Free PMC article.Review.
- [Social cognition in schizophrenia and autism spectrum disorder: Points of convergence and functional differences].Le Gall E, Iakimova G.Le Gall E, et al.Encephale. 2018 Dec;44(6):523-537. doi: 10.1016/j.encep.2018.03.004. Epub 2018 Aug 16.Encephale. 2018.PMID:30122298Review.French.
- A Novel Transfer Learning Approach to Enhance Deep Neural Network Classification of Brain Functional Connectomes.Li H, Parikh NA, He L.Li H, et al.Front Neurosci. 2018 Jul 24;12:491. doi: 10.3389/fnins.2018.00491. eCollection 2018.Front Neurosci. 2018.PMID:30087587Free PMC article.
Cited by
- Retina as a potential biomarker in schizophrenia spectrum disorders: a systematic review and meta-analysis of optical coherence tomography and electroretinography.Komatsu H, Onoguchi G, Silverstein SM, Jerotic S, Sakuma A, Kanahara N, Kakuto Y, Ono T, Yabana T, Nakazawa T, Tomita H.Komatsu H, et al.Mol Psychiatry. 2024 Feb;29(2):464-482. doi: 10.1038/s41380-023-02340-4. Epub 2023 Dec 11.Mol Psychiatry. 2024.PMID:38081943Free PMC article.
- Autosomal recessive cerebellar ataxias: a diagnostic classification approach according to ocular features.Lopergolo D, Rosini F, Pretegiani E, Bargagli A, Serchi V, Rufa A.Lopergolo D, et al.Front Integr Neurosci. 2024 Feb 7;17:1275794. doi: 10.3389/fnint.2023.1275794. eCollection 2023.Front Integr Neurosci. 2024.PMID:38390227Free PMC article.
References
- Dowling JE. The Retina: An Approachable Part of the Brain. Cambridge: Harvard University Press; (1987).
Related information
LinkOut - more resources
Full Text Sources
Other Literature Sources