Multi-schema computational prediction of the comprehensive SARS-CoV-2 vs. human interactome
- PMID:33868814
- PMCID: PMC8029698
- DOI: 10.7717/peerj.11117
Multi-schema computational prediction of the comprehensive SARS-CoV-2 vs. human interactome
Abstract
Background: Understanding the disease pathogenesis of the novel coronavirus, denoted SARS-CoV-2, is critical to the development of anti-SARS-CoV-2 therapeutics. The global propagation of the viral disease, denoted COVID-19 ("coronavirus disease 2019"), has unified the scientific community in searching for possible inhibitory small molecules or polypeptides. A holistic understanding of the SARS-CoV-2 vs. human inter-species interactome promises to identify putative protein-protein interactions (PPI) that may be considered targets for the development of inhibitory therapeutics.
Methods: We leverage two state-of-the-art, sequence-based PPI predictors (PIPE4 & SPRINT) capable of generating the comprehensive SARS-CoV-2 vs. human interactome, comprising approximately 285,000 pairwise predictions. Three prediction schemas (all,proximal,RP-PPI) are leveraged to obtain our highest-confidence subset of PPIs and human proteins predicted to interact with each of the 14 SARS-CoV-2 proteins considered in this study. Notably, the use of the Reciprocal Perspective (RP) framework demonstrates improved predictive performance in multiple cross-validation experiments.
Results: Theall schema identified 279 high-confidence putative interactions involving 225 human proteins, theproximal schema identified 129 high-confidence putative interactions involving 126 human proteins, and theRP-PPI schema identified 539 high-confidence putative interactions involving 494 human proteins. The intersection of the three sets of predictions comprise the seven highest-confidence PPIs. Notably, the Spike-ACE2 interaction was the highest ranked for both the PIPE4 and SPRINT predictors with theall andproximal schemas, corroborating existing evidence for this PPI. Several other predicted PPIs are biologically relevant within the context of the original SARS-CoV virus. Furthermore, the PIPE-Sites algorithm was used to identify the putative subsequence that might mediate each interaction and thereby inform the design of inhibitory polypeptides intended to disrupt the corresponding host-pathogen interactions.
Conclusion: We publicly released the comprehensive sets of PPI predictions and their corresponding PIPE-Sites landscapes in the following DataVerse repository: https://www.doi.org/10.5683/SP2/JZ77XA. The information provided represents theoretical modeling only and caution should be exercised in its use. It is intended as a resource for the scientific community at large in furthering our understanding of SARS-CoV-2.
Keywords: Comprehensive interactome; Inter-species interaction prediction; Machine learning; Protein–protein interaction; SARS-CoV-2.
©2021 Dick et al.
Conflict of interest statement
The authors declare there are no competing interests.
Figures
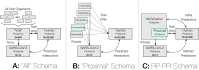
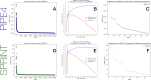
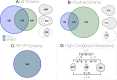
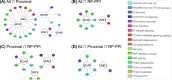

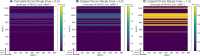
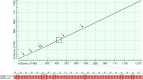
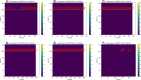
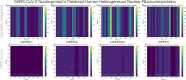
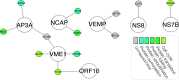
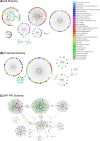
Similar articles
- PIPE4: Fast PPI Predictor for Comprehensive Inter- and Cross-Species Interactomes.Dick K, Samanfar B, Barnes B, Cober ER, Mimee B, Tan LH, Molnar SJ, Biggar KK, Golshani A, Dehne F, Green JR.Dick K, et al.Sci Rep. 2020 Jan 29;10(1):1390. doi: 10.1038/s41598-019-56895-w.Sci Rep. 2020.PMID:31996697Free PMC article.
- COVID-19: viral-host interactome analyzed by network based-approach model to study pathogenesis of SARS-CoV-2 infection.Messina F, Giombini E, Agrati C, Vairo F, Ascoli Bartoli T, Al Moghazi S, Piacentini M, Locatelli F, Kobinger G, Maeurer M, Zumla A, Capobianchi MR, Lauria FN, Ippolito G; COVID 19 INMI Network Medicine for IDs Study Group.Messina F, et al.J Transl Med. 2020 Jun 10;18(1):233. doi: 10.1186/s12967-020-02405-w.J Transl Med. 2020.PMID:32522207Free PMC article.
- Computational Identification of Human Biological Processes and Protein Sequence Motifs Putatively Targeted by SARS-CoV-2 Proteins Using Protein-Protein Interaction Networks.Nadeau R, Shahryari Fard S, Scheer A, Hashimoto-Roth E, Nygard D, Abramchuk I, Chung YE, Bennett SAL, Lavallée-Adam M.Nadeau R, et al.J Proteome Res. 2020 Nov 6;19(11):4553-4566. doi: 10.1021/acs.jproteome.0c00422. Epub 2020 Oct 26.J Proteome Res. 2020.PMID:33103435
- Mapping the SARS-CoV-2-Host Protein-Protein Interactome by Affinity Purification Mass Spectrometry and Proximity-Dependent Biotin Labeling: A Rational and Straightforward Route to Discover Host-Directed Anti-SARS-CoV-2 Therapeutics.Terracciano R, Preianò M, Fregola A, Pelaia C, Montalcini T, Savino R.Terracciano R, et al.Int J Mol Sci. 2021 Jan 7;22(2):532. doi: 10.3390/ijms22020532.Int J Mol Sci. 2021.PMID:33430309Free PMC article.Review.
- Contributions of human ACE2 and TMPRSS2 in determining host-pathogen interaction of COVID-19.Senapati S, Banerjee P, Bhagavatula S, Kushwaha PP, Kumar S.Senapati S, et al.J Genet. 2021;100(1):12. doi: 10.1007/s12041-021-01262-w.J Genet. 2021.PMID:33707363Free PMC article.Review.
Cited by
- Large-scale data mining pipeline for identifying novel soybean genes involved in resistance against the soybean cyst nematode.Nissan N, Hooker J, Arezza E, Dick K, Golshani A, Mimee B, Cober E, Green J, Samanfar B.Nissan N, et al.Front Bioinform. 2023 Jun 20;3:1199675. doi: 10.3389/fbinf.2023.1199675. eCollection 2023.Front Bioinform. 2023.PMID:37409347Free PMC article.
- Graph embedding on mass spectrometry- and sequencing-based biomedical data.Alvarez-Mamani E, Dechant R, Beltran-Castañón CA, Ibáñez AJ.Alvarez-Mamani E, et al.BMC Bioinformatics. 2024 Jan 2;25(1):1. doi: 10.1186/s12859-023-05612-6.BMC Bioinformatics. 2024.PMID:38166530Free PMC article.Review.
- Reciprocal perspective as a super learner improves drug-target interaction prediction (MUSDTI).Dick K, Kyrollos DG, Cosoreanu ED, Dooley J, Fryer JS, Gordon SM, Kharbanda N, Klamrowski M, LaCasse PNL, Leung TF, Nasir MA, Qiu C, Robinson AS, Shao D, Siromahov BR, Starlight E, Tran C, Wang C, Yang YK, Green JR.Dick K, et al.Sci Rep. 2022 Aug 2;12(1):13237. doi: 10.1038/s41598-022-16493-9.Sci Rep. 2022.PMID:35918366Free PMC article.
- Using informative features in machine learning based method for COVID-19 drug repurposing.Aghdam R, Habibi M, Taheri G.Aghdam R, et al.J Cheminform. 2021 Sep 20;13(1):70. doi: 10.1186/s13321-021-00553-9.J Cheminform. 2021.PMID:34544500Free PMC article.
- Bioinformatics resources facilitate understanding and harnessing clinical research of SARS-CoV-2.Ahsan MA, Liu Y, Feng C, Zhou Y, Ma G, Bai Y, Chen M.Ahsan MA, et al.Brief Bioinform. 2021 Mar 22;22(2):714-725. doi: 10.1093/bib/bbaa416.Brief Bioinform. 2021.PMID:33432321Free PMC article.Review.
References
- Chen T, Guestrin C. Xgboost: A scalable tree boosting system. Proceedings of the 22nd acm sigkdd international conference on knowledge discovery and data mining; 2016. pp. 785–794.
LinkOut - more resources
Full Text Sources
Other Literature Sources
Miscellaneous