Classification of low-density EEG for epileptic seizures by energy and fractal features based on EMD
- PMID:32561698
- PMCID: PMC7324275
- DOI: 10.7555/JBR.33.20190009
Classification of low-density EEG for epileptic seizures by energy and fractal features based on EMD
Abstract
We are here to present a new method for the classification of epileptic seizures from electroencephalogram (EEG) signals. It consists of applying empirical mode decomposition (EMD) to extract the most relevant intrinsic mode functions (IMFs) and subsequent computation of the Teager and instantaneous energy, Higuchi and Petrosian fractal dimension, and detrended fluctuation analysis (DFA) for each IMF. We validated the method using a public dataset of 24 subjects with EEG signals from 22 channels and showed that it is possible to classify the epileptic seizures, even with segments of six seconds and a smaller number of channels (e.g., an accuracy of 0.93 using five channels). We were able to create a general machine-learning-based model to detect epileptic seizures of new subjects using epileptic-seizure data from various subjects, after reducing the number of instances, based on the k-means algorithm.
Keywords: detrended fluctuation analysis; electroencephalograms; empirical mode decomposition; energy distribution; epileptic seizure; fractal dimension.
Figures
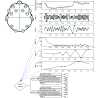
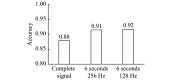
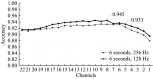
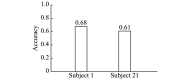
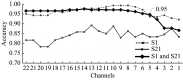
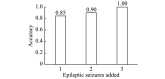
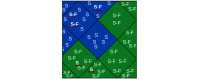
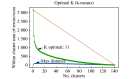
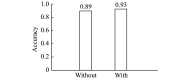
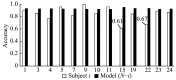
Similar articles
- EEG Channel-Selection Method for Epileptic-Seizure Classification Based on Multi-Objective Optimization.Moctezuma LA, Molinas M.Moctezuma LA, et al.Front Neurosci. 2020 Jun 17;14:593. doi: 10.3389/fnins.2020.00593. eCollection 2020.Front Neurosci. 2020.PMID:32625054Free PMC article.
- Nonlinear and chaos features over EMD/VMD decomposition methods for ictal EEG signals detection.Djemili R, Djemili I.Djemili R, et al.Comput Methods Biomech Biomed Engin. 2024 Nov;27(15):2091-2110. doi: 10.1080/10255842.2023.2271603. Epub 2023 Oct 20.Comput Methods Biomech Biomed Engin. 2024.PMID:37861376
- Epileptic seizure classifications using empirical mode decomposition and its derivative.Karabiber Cura O, Kocaaslan Atli S, Türe HS, Akan A.Karabiber Cura O, et al.Biomed Eng Online. 2020 Feb 14;19(1):10. doi: 10.1186/s12938-020-0754-y.Biomed Eng Online. 2020.PMID:32059668Free PMC article.
- Automatic identification of epileptic seizures using volume of phase space representation.Krishnaprasanna R, Vijaya Baskar V, Panneerselvam J.Krishnaprasanna R, et al.Phys Eng Sci Med. 2021 Jun;44(2):545-556. doi: 10.1007/s13246-021-01006-1. Epub 2021 May 6.Phys Eng Sci Med. 2021.PMID:33956327
- Machine Learning-Based Epileptic Seizure Detection Methods Using Wavelet and EMD-Based Decomposition Techniques: A Review.Thangarajoo RG, Reaz MBI, Srivastava G, Haque F, Ali SHM, Bakar AAA, Bhuiyan MAS.Thangarajoo RG, et al.Sensors (Basel). 2021 Dec 20;21(24):8485. doi: 10.3390/s21248485.Sensors (Basel). 2021.PMID:34960577Free PMC article.Review.
Cited by
- Editorial commentary on special issue of Advances in EEG Signal Processing and Machine Learning for Epileptic Seizure Detection and Prediction.Boubchir L.Boubchir L.J Biomed Res. 2020 May 28;34(3):149-150. doi: 10.7555/JBR.34.20200700.J Biomed Res. 2020.PMID:32561694Free PMC article.
- Two-dimensional CNN-based distinction of human emotions from EEG channels selected by multi-objective evolutionary algorithm.Moctezuma LA, Abe T, Molinas M.Moctezuma LA, et al.Sci Rep. 2022 Mar 3;12(1):3523. doi: 10.1038/s41598-022-07517-5.Sci Rep. 2022.PMID:35241745Free PMC article.
- Neural Decoding of EEG Signals with Machine Learning: A Systematic Review.Saeidi M, Karwowski W, Farahani FV, Fiok K, Taiar R, Hancock PA, Al-Juaid A.Saeidi M, et al.Brain Sci. 2021 Nov 18;11(11):1525. doi: 10.3390/brainsci11111525.Brain Sci. 2021.PMID:34827524Free PMC article.Review.
- EEG Channel-Selection Method for Epileptic-Seizure Classification Based on Multi-Objective Optimization.Moctezuma LA, Molinas M.Moctezuma LA, et al.Front Neurosci. 2020 Jun 17;14:593. doi: 10.3389/fnins.2020.00593. eCollection 2020.Front Neurosci. 2020.PMID:32625054Free PMC article.
References
Adeli H, Ghosh-Dastidar S. Automated EEG-based diagnosis of neurological disorders: inventing the future of neurology[M]. Boca Raton: CRC Press, 2010: 1–423.
- Devinsky O Diagnosis and treatment of temporal lobe epilepsy. Rev Neurol Dis. 2004;1(1):2–9. - PubMed
Related information
LinkOut - more resources
Full Text Sources
Other Literature Sources