Identification of diagnostic biomarkers in patients with gestational diabetes mellitus based on transcriptome gene expression and methylation correlation analysis
- PMID:31881887
- PMCID: PMC6933721
- DOI: 10.1186/s12958-019-0556-x
Identification of diagnostic biomarkers in patients with gestational diabetes mellitus based on transcriptome gene expression and methylation correlation analysis
Abstract
Background: Gestational diabetes mellitus (GDM) has a high prevalence in the period of pregnancy. However, the lack of gold standards in current screening and diagnostic methods posed the biggest limitation. Regulation of gene expression caused by DNA methylation plays an important role in metabolic diseases. In this study, we aimed to screen GDM diagnostic markers, and establish a diagnostic model for predicting GDM.
Methods: First, we acquired data of DNA methylation and gene expression in GDM samples (N = 41) and normal samples (N = 41) from the Gene Expression Omnibus (GEO) database. After pre-processing the data, linear models were used to identify differentially expressed genes (DEGs). Then we performed pathway enrichment analysis to extract relationships among genes from pathways, construct pathway networks, and further analyzed the relationship between gene expression and methylation of promoter regions. We screened for genes which are significantly negatively correlated with methylation and established mRNA-mRNA-CpGs network. The network topology was further analyzed to screen hub genes which were recognized as robust GDM biomarkers. Finally, the samples were randomly divided into training set (N = 28) and internal verification set (N = 27), and the support vector machine (SVM) ten-fold cross-validation method was used to establish a diagnostic classifier, which verified on internal and external data sets.
Results: In this study, we identified 465 significant DEGs. Functional enrichment analysis revealed that these genes were associated with Type I diabetes mellitus and immunization. And we constructed an interactional network including 1091 genes by using the regulatory relationships of all 30 enriched pathways. 184 epigenetics regulated genes were screened by analyzing the relationship between gene expression and promoter regions' methylation in the network. Moreover, the accuracy rate in the training data set was increased up to 96.3, and 82.1% in the internal validation set, and 97.3% in external validation data sets after establishing diagnostic classifiers which were performed by analyzing the gene expression profiles of obtained 10 hub genes from this network, combined with SVM.
Conclusions: This study provided new features for the diagnosis of GDM and may contribute to the diagnosis and personalized treatment of GDM.
Keywords: Diagnostic markers; GDM; Methylation; Pathway; SVM.
Conflict of interest statement
The authors declare no conflicts of interest in the paper.
Figures
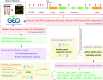
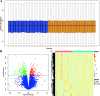
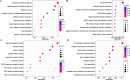
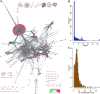
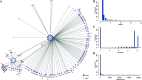
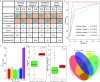
Similar articles
- Identification of Diagnostic CpG Signatures in Patients with Gestational Diabetes Mellitusvia Epigenome-Wide Association Study Integrated with Machine Learning.Liu Y, Geng H, Duan B, Yang X, Ma A, Ding X.Liu Y, et al.Biomed Res Int. 2021 May 19;2021:1984690. doi: 10.1155/2021/1984690. eCollection 2021.Biomed Res Int. 2021.PMID:34104645Free PMC article.
- Identification of hub-methylated differentially expressed genes in patients with gestational diabetes mellitus by multi-omic WGCNA basing epigenome-wide and transcriptome-wide profiling.Chen M, Yan J, Han Q, Luo J, Zhang Q.Chen M, et al.J Cell Biochem. 2020 Jun;121(5-6):3173-3184. doi: 10.1002/jcb.29584. Epub 2019 Dec 30.J Cell Biochem. 2020.PMID:31886571
- Comprehensive analysis of DNA methylation and gene expression profiles in gestational diabetes mellitus.He J, Liu K, Hou X, Lu J.He J, et al.Medicine (Baltimore). 2021 Jul 2;100(26):e26497. doi: 10.1097/MD.0000000000026497.Medicine (Baltimore). 2021.PMID:34190178Free PMC article.
- Molecular Biomarkers for Gestational Diabetes Mellitus.Dias S, Pheiffer C, Abrahams Y, Rheeder P, Adam S.Dias S, et al.Int J Mol Sci. 2018 Sep 26;19(10):2926. doi: 10.3390/ijms19102926.Int J Mol Sci. 2018.PMID:30261627Free PMC article.Review.
- Deciphering DNA Methylation in Gestational Diabetes Mellitus: Epigenetic Regulation and Potential Clinical Applications.Li N, Liu HY, Liu SM.Li N, et al.Int J Mol Sci. 2024 Aug 29;25(17):9361. doi: 10.3390/ijms25179361.Int J Mol Sci. 2024.PMID:39273309Free PMC article.Review.
Cited by
- Chordin-like 1 is a novel prognostic biomarker and correlative with immune cell infiltration in lung adenocarcinoma.Deng B, Chen X, Xu L, Zheng L, Zhu X, Shi J, Yang L, Wang D, Jiang D.Deng B, et al.Aging (Albany NY). 2022 Jan 12;14(1):389-409. doi: 10.18632/aging.203814. Epub 2022 Jan 12.Aging (Albany NY). 2022.PMID:35021154Free PMC article.
- Identification of Diagnostic CpG Signatures in Patients with Gestational Diabetes Mellitusvia Epigenome-Wide Association Study Integrated with Machine Learning.Liu Y, Geng H, Duan B, Yang X, Ma A, Ding X.Liu Y, et al.Biomed Res Int. 2021 May 19;2021:1984690. doi: 10.1155/2021/1984690. eCollection 2021.Biomed Res Int. 2021.PMID:34104645Free PMC article.
- UHPLC-MS/MS-Based Metabolomics and Clinical Phenotypes Analysis Reveal Broad-Scale Perturbations in Early Pregnancy Related to Gestational Diabetes Mellitus.Hu T, An Z, Li H, Liu Y, Xia L, Qiu L, Yao A, Ma L, Liu L.Hu T, et al.Dis Markers. 2022 Aug 24;2022:4231031. doi: 10.1155/2022/4231031. eCollection 2022.Dis Markers. 2022.PMID:36061360Free PMC article.
- Epigenetic marks associated with gestational diabetes mellitus across two time points during pregnancy.Linares-Pineda T, Peña-Montero N, Fragoso-Bargas N, Gutiérrez-Repiso C, Lima-Rubio F, Suarez-Arana M, Sánchez-Pozo A, Tinahones FJ, Molina-Vega M, Picón-César MJ, Sommer C, Morcillo S.Linares-Pineda T, et al.Clin Epigenetics. 2023 Jul 6;15(1):110. doi: 10.1186/s13148-023-01523-8.Clin Epigenetics. 2023.PMID:37415231Free PMC article.
- Study on the relationship between DNA methylation of target CpG sites in peripheral blood and gestational diabetes during early pregnancy.Wang X, Huang J, Zheng Y, Long S, Lin H, Zhang N, Tian M, Wu X, An R, Ma S, Tan H.Wang X, et al.Sci Rep. 2021 Oct 14;11(1):20455. doi: 10.1038/s41598-021-99836-2.Sci Rep. 2021.PMID:34650136Free PMC article.
References
- Weinert LS. International Association of Diabetes and Pregnancy Study Groups recommendations on the diagnosis and classification of hyperglycemia in pregnancy: comment to the International Association of Diabetes and Pregnancy Study Groups Consensus Panel. Diabetes Care. 2010;33(7):e97–ee8. doi: 10.2337/dc10-0544. - DOI - PubMed
MeSH terms
Substances
Related information
Grants and funding
LinkOut - more resources
Full Text Sources