Ten quick tips for machine learning in computational biology
- PMID:29234465
- PMCID: PMC5721660
- DOI: 10.1186/s13040-017-0155-3
Ten quick tips for machine learning in computational biology
Abstract
Machine learning has become a pivotal tool for many projects in computational biology, bioinformatics, and health informatics. Nevertheless, beginners and biomedical researchers often do not have enough experience to run a data mining project effectively, and therefore can follow incorrect practices, that may lead to common mistakes or over-optimistic results. With this review, we present ten quick tips to take advantage of machine learning in any computational biology context, by avoiding some common errors that we observed hundreds of times in multiple bioinformatics projects. We believe our ten suggestions can strongly help any machine learning practitioner to carry on a successful project in computational biology and related sciences.
Keywords: Bioinformatics; Biomedical informatics; Computational biology; Computational intelligence; Data mining; Health informatics; Machine learning; Tips.
Conflict of interest statement
Ethics approval and consent to participate
Not applicable.
Consent for publication
Not applicable.
Competing interests
The author declares that he has no competing interests.
Publisher’s Note
Springer Nature remains neutral with regard to jurisdictional claims in published maps and institutional affiliations.
Figures
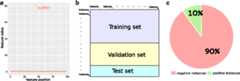
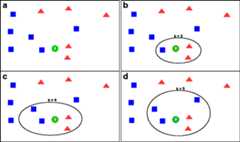
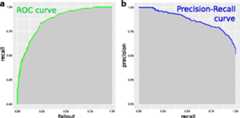
Similar articles
- Eleven quick tips for data cleaning and feature engineering.Chicco D, Oneto L, Tavazzi E.Chicco D, et al.PLoS Comput Biol. 2022 Dec 15;18(12):e1010718. doi: 10.1371/journal.pcbi.1010718. eCollection 2022 Dec.PLoS Comput Biol. 2022.PMID:36520712Free PMC article.
- Ten quick tips for bioinformatics analyses using an Apache Spark distributed computing environment.Chicco D, Ferraro Petrillo U, Cattaneo G.Chicco D, et al.PLoS Comput Biol. 2023 Jul 20;19(7):e1011272. doi: 10.1371/journal.pcbi.1011272. eCollection 2023 Jul.PLoS Comput Biol. 2023.PMID:37471333Free PMC article.
- Ten quick tips for avoiding pitfalls in multi-omics data integration analyses.Chicco D, Cumbo F, Angione C.Chicco D, et al.PLoS Comput Biol. 2023 Jul 6;19(7):e1011224. doi: 10.1371/journal.pcbi.1011224. eCollection 2023 Jul.PLoS Comput Biol. 2023.PMID:37410704Free PMC article.
- Machine learning in bioinformatics: a brief survey and recommendations for practitioners.Bhaskar H, Hoyle DC, Singh S.Bhaskar H, et al.Comput Biol Med. 2006 Oct;36(10):1104-25. doi: 10.1016/j.compbiomed.2005.09.002. Epub 2005 Oct 13.Comput Biol Med. 2006.PMID:16226240Review.
- Explainable AI for Bioinformatics: Methods, Tools and Applications.Karim MR, Islam T, Shajalal M, Beyan O, Lange C, Cochez M, Rebholz-Schuhmann D, Decker S.Karim MR, et al.Brief Bioinform. 2023 Sep 20;24(5):bbad236. doi: 10.1093/bib/bbad236.Brief Bioinform. 2023.PMID:37478371Review.
Cited by
- Towards a potential pan-cancer prognostic signature for gene expression based on probesets and ensemble machine learning.Chicco D, Alameer A, Rahmati S, Jurman G.Chicco D, et al.BioData Min. 2022 Nov 3;15(1):28. doi: 10.1186/s13040-022-00312-y.BioData Min. 2022.PMID:36329531Free PMC article.
- A genome scale transcriptional regulatory model of the human placenta.Paquette A, Ahuna K, Hwang YM, Pearl J, Liao H, Shannon P, Kadam L, Lapehn S, Bucher M, Roper R, Funk C, MacDonald J, Bammler T, Baloni P, Brockway H, Mason WA, Bush N, Lewinn KZ, Karr CJ, Stamatoyannopoulos J, Muglia LJ, Jones H, Sadovsky Y, Myatt L, Sathyanarayana S, Price ND.Paquette A, et al.Sci Adv. 2024 Jun 28;10(26):eadf3411. doi: 10.1126/sciadv.adf3411. Epub 2024 Jun 28.Sci Adv. 2024.PMID:38941464Free PMC article.
- Evaluation of penalized and machine learning methods for asthma disease prediction in the Korean Genome and Epidemiology Study (KoGES).Choi Y, Cha J, Choi S.Choi Y, et al.BMC Bioinformatics. 2024 Feb 2;25(1):56. doi: 10.1186/s12859-024-05677-x.BMC Bioinformatics. 2024.PMID:38308205Free PMC article.
- Prediction of Left Ventricular Mechanics Using Machine Learning.Dabiri Y, Van der Velden A, Sack KL, Choy JS, Kassab GS, Guccione JM.Dabiri Y, et al.Front Phys. 2019 Sep;7:117. doi: 10.3389/fphy.2019.00117. Epub 2019 Sep 6.Front Phys. 2019.PMID:31903394Free PMC article.
- Predicting and explaining the impact of genetic disruptions and interactions on organismal viability.Al-Anzi BF, Khajah M, Fakhraldeen SA.Al-Anzi BF, et al.Bioinformatics. 2022 Sep 2;38(17):4088-4099. doi: 10.1093/bioinformatics/btac519.Bioinformatics. 2022.PMID:35861390Free PMC article.
References
- Baldi P, Brunak S. Bioinformatics: the machine learning approach. Cambridge: MIT press; 2001.
- Schölkopf B, Tsuda K, Vert J-P . Kernel methods in computational biology. Cambridge: MIT Press; 2004.
Publication types
Related information
LinkOut - more resources
Full Text Sources
Other Literature Sources