Effects of GC bias in next-generation-sequencing data on de novo genome assembly
- PMID:23638157
- PMCID: PMC3639258
- DOI: 10.1371/journal.pone.0062856
Effects of GC bias in next-generation-sequencing data on de novo genome assembly
Abstract
Next-generation-sequencing (NGS) has revolutionized the field of genome assembly because of its much higher data throughput and much lower cost compared with traditional Sanger sequencing. However, NGS poses new computational challenges to de novo genome assembly. Among the challenges, GC bias in NGS data is known to aggravate genome assembly. However, it is not clear to what extent GC bias affects genome assembly in general. In this work, we conduct a systematic analysis on the effects of GC bias on genome assembly. Our analyses reveal that GC bias only lowers assembly completeness when the degree of GC bias is above a threshold. At a strong GC bias, the assembly fragmentation due to GC bias can be explained by the low coverage of reads in the GC-poor or GC-rich regions of a genome. This effect is observed for all the assemblers under study. Increasing the total amount of NGS data thus rescues the assembly fragmentation because of GC bias. However, the amount of data needed for a full rescue depends on the distribution of GC contents. Both low and high coverage depths due to GC bias lower the accuracy of assembly. These pieces of information provide guidance toward a better de novo genome assembly in the presence of GC bias.
Conflict of interest statement
Figures
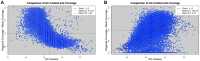
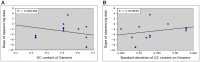
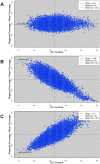
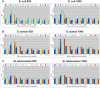
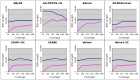
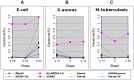
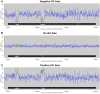
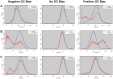
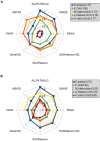
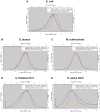
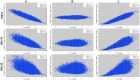
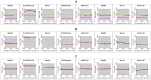
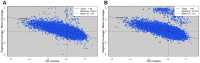
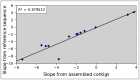
Similar articles
- Optimizing information in Next-Generation-Sequencing (NGS) reads for improving de novo genome assembly.Liu T, Tsai CH, Lee WB, Chiang JH.Liu T, et al.PLoS One. 2013 Jul 29;8(7):e69503. doi: 10.1371/journal.pone.0069503. Print 2013.PLoS One. 2013.PMID:23922726Free PMC article.
- Optimizing hybrid assembly of next-generation sequence data from Enterococcus faecium: a microbe with highly divergent genome.Wang Y, Yu Y, Pan B, Hao P, Li Y, Shao Z, Xu X, Li X.Wang Y, et al.BMC Syst Biol. 2012;6 Suppl 3(Suppl 3):S21. doi: 10.1186/1752-0509-6-S3-S21. Epub 2012 Dec 17.BMC Syst Biol. 2012.PMID:23282199Free PMC article.
- SMRT sequencing only de novo assembly of the sugar beet (Beta vulgaris) chloroplast genome.Stadermann KB, Weisshaar B, Holtgräwe D.Stadermann KB, et al.BMC Bioinformatics. 2015 Sep 16;16(1):295. doi: 10.1186/s12859-015-0726-6.BMC Bioinformatics. 2015.PMID:26377912Free PMC article.
- The present and future of de novo whole-genome assembly.Sohn JI, Nam JW.Sohn JI, et al.Brief Bioinform. 2018 Jan 1;19(1):23-40. doi: 10.1093/bib/bbw096.Brief Bioinform. 2018.PMID:27742661Review.
- Next-generation sequencing technologies and their impact on microbial genomics.Forde BM, O'Toole PW.Forde BM, et al.Brief Funct Genomics. 2013 Sep;12(5):440-53. doi: 10.1093/bfgp/els062. Epub 2013 Jan 11.Brief Funct Genomics. 2013.PMID:23314033Review.
Cited by
- An overlooked phenomenon: complex interactions of potential error sources on the quality of bacterial de novo genome assemblies.Rádai Z, Váradi A, Takács P, Nagy NA, Schmitt N, Prépost E, Kardos G, Laczkó L.Rádai Z, et al.BMC Genomics. 2024 Jan 9;25(1):45. doi: 10.1186/s12864-023-09910-4.BMC Genomics. 2024.PMID:38195441Free PMC article.
- Population structure of mitochondrial genomes in Saccharomyces cerevisiae.Wolters JF, Chiu K, Fiumera HL.Wolters JF, et al.BMC Genomics. 2015 Jun 11;16(1):451. doi: 10.1186/s12864-015-1664-4.BMC Genomics. 2015.PMID:26062918Free PMC article.
- Modeling of shotgun sequencing of DNA plasmids using experimental and theoretical approaches.Shityakov S, Bencurova E, Förster C, Dandekar T.Shityakov S, et al.BMC Bioinformatics. 2020 Apr 3;21(1):132. doi: 10.1186/s12859-020-3461-6.BMC Bioinformatics. 2020.PMID:32245400Free PMC article.
- Unveiling lignocellulolytic trait of a goat omasum inhabitant Klebsiella variicola strain HSTU-AAM51 in light of biochemical and genome analyses.Md Abdullah-Al-Mamun, Hossain MS, Debnath GC, Sultana S, Rahman A, Hasan Z, Das SR, Ashik MA, Prodhan MY, Aktar S, Cho KM, Haque MA.Md Abdullah-Al-Mamun, et al.Braz J Microbiol. 2022 Mar;53(1):99-130. doi: 10.1007/s42770-021-00660-7. Epub 2022 Jan 28.Braz J Microbiol. 2022.PMID:35088248Free PMC article.
- The large genome size variation in the Hesperis clade was shaped by the prevalent proliferation of DNA repeats and rarer genome downsizing.Hloušková P, Mandáková T, Pouch M, Trávníček P, Lysak MA.Hloušková P, et al.Ann Bot. 2019 Aug 2;124(1):103-120. doi: 10.1093/aob/mcz036.Ann Bot. 2019.PMID:31220201Free PMC article.
References
- Schuster SC (2008) Next-generation sequencing transforms today's biology. Nat Methods 5: 16–18. - PubMed
- Paszkiewicz K, Studholme DJ (2010) De novo assembly of short sequence reads. Brief Bioinform 11: 457–472. - PubMed
- Metzker ML (2010) Sequencing technologies - the next generation. Nat Rev Genet 11: 31–46. - PubMed
Publication types
MeSH terms
Related information
Grants and funding
LinkOut - more resources
Full Text Sources
Other Literature Sources
Miscellaneous