the Creative Commons Attribution 4.0 License.
the Creative Commons Attribution 4.0 License.
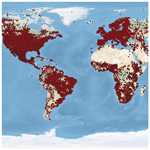
Standardised soil profile data to support global mapping and modelling (WoSIS snapshot 2019)
The World Soil Information Service (WoSIS) providesquality-assessed and standardised soil profile data to support digital soilmapping and environmental applications at broadscale levels. Since therelease of the first “WoSIS snapshot”, in July 2016, many new soil data wereshared with us, registered in the ISRIC data repository and subsequentlystandardised in accordance with the licences specified by the dataproviders. Soil profile data managed in WoSIS were contributed by a widerange of data providers; therefore, special attention was paid to measuresfor soil data quality and the standardisation of soil property definitions,soil property values (and units of measurement) and soil analytical methoddescriptions. We presently consider the following soil chemical properties:organic carbon, total carbon, total carbonate equivalent, total nitrogen,phosphorus (extractable P, total P and P retention), soil pH, cationexchange capacity and electrical conductivity. We also consider the following physical properties:soil texture (sand, silt, and clay), bulk density, coarse fragments andwater retention. Both of these sets of properties are grouped according to analytical procedures that areoperationally comparable. Further, for each profile we provide the originalsoil classification (FAO, WRB, USDA), version and horizon designations,insofar as these have been specified in the source databases. Measures forgeographical accuracy (i.e. location) of the point data, as well as a firstapproximation for the uncertainty associated with the operationally definedanalytical methods, are presented for possible consideration in digital soilmapping and subsequent earth system modelling. The latest (dynamic) set ofquality-assessed and standardised data, called “wosis_latest”, is freely accessible via an OGC-compliant WFS (web featureservice). For consistent referencing, we also provide time-specific static“snapshots”. The present snapshot (September 2019) is comprised of 196 498geo-referenced profiles originating from 173 countries. They represent over832 000 soil layers (or horizons) and over 5.8 million records. The actualnumber of observations for each property varies (greatly) between profilesand with depth, generally depending on the objectives of the initialsoil sampling programmes. In the coming years, we aim to fill gradually gapsin the geographic distribution and soil property data themselves, thissubject to the sharing of a wider selection of soil profile data for so farunder-represented areas and properties by our existing and prospectivepartners. Part of this work is foreseen in conjunction within the GlobalSoil Information System (GloSIS) being developed by the Global SoilPartnership (GSP). The “WoSIS snapshot – September 2019” is archived andfreely accessible athttps://doi.org/10.17027/isric-wdcsoils.20190901(Batjes et al., 2019).
According to a recent review, so far over 800 000 soil profiles have beenrescued and compiled into databases over the past few decades (Arrouays etal., 2017). However, only a fraction thereof is readily accessible (i.e.shared) in a consistent format for the greater benefit of the internationalcommunity. This paper describes procedures for preserving,quality-assessing, standardising and subsequently providing consistentworld soil data to the international community, as developed in the frameworkof the Data or WoSIS (World Soil Information Service) project sincethe release of the first snapshot in 2016 (Batjes et al.,2017); this collaborative project draws on an increasingly large complementof shared soil profile data. Ultimately, WoSIS aims to provide consistentharmonised soil data, derived from a wide range of legacy holdings as wellas from more recently developed soil datasets derived from proximal sensing(e.g. soil spectral libraries; see Terhoeven-Urselmans et al., 2010;Viscarra Rossel et al., 2016), in an interoperable mode and preferablywithin the setting of a federated, global soil information system(GLOSIS; see GSP-SDF, 2018).
We follow the definition of harmonisation used by the Global SoilPartnership (GSP, Baritz et al., 2014). It encompasses “providingmechanisms for the collation, analysis and exchange of consistent andcomparable global soil data and information”. The following domains need tobe considered according to GSP's definition: (a) soil description,classification, and mapping; (b) soil analyses; (c) exchange of digital soildata; and (d) interpretations. In view of the breadth and magnitude of thetask, as indicated earlier (Batjes et al., 2017), we haverestricted ourselves to the standardisation of soil property definitions,soil analytical method descriptions and soil property values (i.e.measurement units). We have expanded the number of soil propertiesconsidered in the preceding snapshot, i.e. those listed in the GlobalSoilMap (2015) specifications, gradually working towards the range of soilproperties commonly considered in other global soil data compilationprogrammes (Batjes, 2016; FAO et al., 2012; van Engelen and Dijkshoorn,2013).
Soil characterisation data, such as pH and bulk density, are collatedaccording to a wide range of analytical procedures. Such data can be moreappropriately used when the procedures for their collection, analysis andreporting are well understood. As indicated by USDA Soil Survey Staff (2011), results differ when different analyticalmethods are used, even though these methods may carry the same name (e.g.soil pH) or concept. This complicates, or sometimes precludes, comparison ofone set of data with another if it is not known how both sets werecollected and analysed. Hence, our use of “operational definitions” for soilproperties that are linked to specific methods. As an example, we mayconsider the “pH of a soil”. This requires information on samplepretreatment, soil ∕ solution ratio and description of solution (e.g.H2O, 1 M KCl, 0.02 MCaCl2, or 1 M NaF) to be fully understood. The pH levelmeasured in sodium fluoride (pH NaF), for example, provides a measure forthe phosphorus (P) retention of a soil, whereas pH measured in water (pHH2O) is an indicator for soil nutrient status. Consequently, in WoSIS,soil properties are defined by the analytical methods and the terminologyused, based on common practice in soil science.
This paper discusses methodological changes in the WoSIS workflow since therelease of the preceding snapshot (Batjes et al., 2017),describes the data screening procedure, provides a detailed overview of thedatabase content, explains how the new set of standardised data can beaccessed and outlines future developments. The data model for theunderpinning PostgreSQL database itself is described in a recently updatedprocedures manual (Ribeiro et al., 2018); these largelytechnical aspects are considered beyond the scope of this paper.
Quality-assessed data provided through WoSIS can be (and have been) used forvarious purposes. For example, as point data for making soil property mapsat various spatial-scale levels, using digital soil mapping techniques(Arrouays et al., 2017; Guevara et al., 2018; Hengl et al., 2017a, b; Moulatlet et al., 2017). Such property maps, for example, canbe used to study global effects of soil and climate on leaf photosynthetictraits and rates (Maire et al., 2015), generate maps ofroot zone plant-available water capacity (Leenaars et al.,2018) in support of yield gap analyses (van Ittersum et al.,2013), assess impacts of long-term human land use on world soil carbonstocks (Sanderman et al., 2017), or the effects of tillagepractices on soil gaseous emissions (Lutz et al., 2019). Inturn, this type of information can help to inform global conventionssuch as the UNCCD (United Nations Convention to Combat Desertification) andUNFCCC (United Nations Framework Convention on Climate Change) so thatpolicymakers and business leaders can make informed decisions aboutenvironmental and societal well-being.
The overall workflow for acquiring, ingesting and processing data in WoSIShas been described in an earlier paper (Batjes et al.,2017). To avoid repetition, we will only name the main steps here (Fig. 1).These are, successively, (a) store submitted datasets with their metadata(including the licence defining access rights) in the ISRIC Data Repository;(b) import all datasets “as is” into PostgreSQL; (c) ingest the data into theWoSIS data model, including basic data quality assessment and control; (d) standardise the descriptions for the soil analytical methods and the unitsof measurement; and (e) ultimately, upon final consistency checks,distribute the quality-assessed and standardised data via WFS (webfeature service) and other formats (e.g. TSV for snapshots).

Figure 1Schematic representation of the WoSIS workflow forsafeguarding and processing disparate soils datasets.
As indicated, datasets shared with our centre are first stored in the ISRICData Repository, together with their metadata (currently representing some452 000 profiles) and the licence and data-sharing agreement in particular,in line with the ISRIC Data Policy (ISRIC, 2016). For theWoSIS standardisation workflow proper, we only consider those datasets (orprofiles) that have a “non-restrictive” Creative Commons (CC) licence aswell as a defined complement of attributes (see Appendix A).Non-restrictive has been defined here as at least a CC-BY (attribution) orCC-BY-NC (attribution non-commercial) licence. Presently, this correspondswith data for some 196 498 profiles (i.e. profiles that have the rightlicence and data for at least one of the standard soil properties).Alternatively, some datasets may only be used for digital soil mapping usingSoilGrids™, corresponding with an additional 42 000 profiles,corresponding to some 18 % of the total amount of standardised profiles(∼238 000). Although the latter profiles are quality-assessedand standardised following the regular WoSIS workflow, they are not distributed to theinternational community in accordance with the underpinning licenceagreements; as such, their description is beyond the scope of the presentpaper. Finally, several datasets have licences indicating that they shouldonly be safeguarded in the repository; inherently, these are not being usedfor any data processing.
3.1 Consistency checks
Soil profile data submitted for consideration in WoSIS were collatedaccording to various national or international standards and presented invarious formats (from paper to digital). Further, they are of varying degreesof completeness, as discussed below. Proper documentation of the provenanceand identification of each dataset and, ideally, each observation ormeasurement is necessary to allow for efficient processing of the sourcedata. The following need to be specified: profiles and layers referenced by feature (x–y–z) and time (t), attribute (class, site, layer field andlayer lab), method, and value, including units of expression.
To be considered in the actual WoSIS standardisation workflow, each profilemust meet several criteria (Table 1). First, we assess if each profile isgeo-referenced, has (consistently) defined upper and lower depths for eachlayer (or horizon), and has data for at least some soil properties (e.g. sand,silt, clay and pH). Having a soil (taxonomic) classification is considereddesirable (case 1) but not mandatory (case 2). Georeferenced profilesfor which only the classification is specified can still be useful formapping of soil taxonomic classes (case 3). Alternatively, profiles withoutany geo-reference may still prove useful to develop pedotransfer functions(case 4 and 5); however, they cannot be served through WFS (because there isno geometry,x,y). The remaining cases (6 and 7) are automatically excludedfrom the WoSIS workflow. This first broad consistency check led to theexclusion of over 50 000 profiles from the initial complement of soilprofiles.
Table 1Basic requirements for considering soil profiles in theWoSIS standardisation workflow.
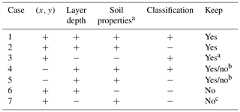
a Such profiles may be used to generate maps of soil taxonomic classesusing
SoilGrids™ (Hengl et al., 2017b).b Such profiles (geo-referenced solely according
to their country oforigin) may be useful for developing pedotransfer functions. Hence, they arestandardised, though they are not distributed with the snapshot, as they lack (x,y)coordinates.c Lacking information on the depth of sampling (i.e. layer), thedifferent soil properties cannot be meaningfully grouped to developpedotransfer functions.
Consistency in layer depth (i.e. sequential increase in the upper and lowerdepth reported for each layer down the profile) is checked using automatedprocedures (see Sect. 3.2). In accord with currentinternationally accepted conventions, such depth increments are given as“measured from the surface, including organic layers and mineral covers”(FAO, 2006; Schoeneberger et al., 2012). Priorto 1993, however, the beginning (zero datum) of the profile was set at the topof the mineral surface (the solum proper), except for “thick” organic layers as defined for peat soils (FAO-ISRIC, 1986; FAO, 1977). Organic horizonswere recorded as above and mineral horizons recorded as below, relative tothe mineral surface (Schoeneberger et al., 2012, pp. 2–6). Insofar as is possible, such “surficial litter” layers are flagged inWoSIS as an auxiliary variable (see Appendix B) so that they may befiltered out during auxiliary computations of soil organic carbon stocks,for example.
3.2 Flagging duplicate profiles
Several source materials, such as the harmonised WISE soil profile database(Batjes, 2009), the Africa Soil Profile Database(AfSP, Leenaars et al., 2014) and the dataset collatedby the International Soil Carbon Network (ISCN, Nave et al., 2017)are compilations of shared soil profile data. These three datasets, forexample, contain varying amounts of profiles derived from the NationalCooperative Soil Survey database (USDA-NCSS, 2018), an importantsource of freely shared, primary soil data. The original NCSS profileidentifiers, however, may not always have been preserved “as is” in thevarious data compilations.
To avoid duplication in the WoSIS database, soil profiles located within 100 m of each other are flagged as possible duplicates. Upon additional,semi-automated checks concerning the first three layers (upper and lowerdepth), i.e. sand, silt and clay content, the duplicates with theleast comprehensive component of attribute data are flagged and excludedfrom further processing. When still in doubt at this stage, additionalvisual checks are made with respect to other commonly reported soilproperties, such as pHwater and organic carbon content. This laborious,yet critical, screening process (see Ribeiro et al., 2018)led to the exclusion of some 50 000 additional profiles from the initialcomplement of soil profile data.
3.3 Ensuring naming consistency
The next key stage has been the standardisation of soil property names to theWoSIS conventions, as well as the standardisation of the soil analyticalmethods descriptions themselves (see Appendix A). Quality checks considerthe units of measurement, plausible ranges for defined soil properties (e.g.soil pH cannot exceed 14) using checks on minimum, average and maximumvalues for each source dataset. Data that do not fulfil the requirementsare flagged and not considered further in the workflow, unless the observed“inconsistencies” can easily be fixed (e.g. blatant typos in pH values). Thewhole procedure, with flowcharts and option tables, is documented in theWoSIS Procedures Manual (see Appendices D, E and F in Ribeiroet al., 2018).
Presently, we standardise the following set of soil properties in WoSIS.
Chemical. Organic carbon, total carbon (i.e. organic plus inorganic carbon),total nitrogen, total carbonate equivalent (inorganic carbon), soil pH,cation exchange capacity, electrical conductivity and phosphorus(extractable P, total P and P retention).
Physical. Soil texture (sand, silt and clay), coarse fragments, bulkdensity and water retention.
It should be noted that all measurement values are reported as recorded inthe source data, subsequent to the above consistency checks (andstandardisation of the units of measurement to the target units; seeAppendix A). As such, we neither apply “gap-filling” procedures in WoSIS, e.g. when only the sand and silt fractions are reported, nor do we applypedotransfer functions to derive soil hydrological properties. This nextstage of data processing is seen as the responsibility of the data users(modellers) themselves, as the required functions or means ofdepth-aggregating the layer data will vary with the projected use(s) of thestandardised data (see Finke, 2006; Hendriks et al., 2016; Van Looy etal., 2017).
3.4 Providing measures for geographic and attribute accuracy
It is well known that “soil observations used for calibration andinterpolation are themselves not error free” (Baroni et al., 2017;Cressie and Kornak, 2003; Folberth et al., 2016; Grimm and Behrens, 2010;Guevara et al., 2018; Hengl et al., 2017b; Heuvelink, 2014; Heuvelink andBrown, 2006). Hence, we provide measures for the geographic accuracy of thepoint locations as well as the accuracy of the laboratory measurements forpossible consideration in digital soil mapping and subsequent earth systemmodelling (Dai et al., 2019).
All profile coordinates in WoSIS are presented according to the WorldGeodetic System (i.e. WGS84, EPSG code 4326). These coordinates wereconverted from a diverse range of national projections. Further, the sourcereferencing may have been in decimal degrees (DD) or expressed in degrees,minutes, and seconds (DMS) for both latitude and longitude. The (approximate)accuracy of georeferencing in WoSIS is given in decimal degrees. If thesource only provided degrees, minutes, and seconds (DMS) then the geographicaccuracy is set at 0.01; if seconds (DM) are missing it is set at 0.1; and if secondsand minutes (D) are missing it is set at 1. For most profiles (86 %; see Table 2),the approximate accuracy of the point locations, as inferred from theoriginal coordinates given in the source datasets, is less than 10 m(total=196 498 profiles; see Sect. 4). Typically, the geo-referencingof soil profiles described and sampled before the advent of GPS (GlobalPositioning Systems) in the 1970s is less accurate; sometimes we just do notknow the “true” accuracy. Digital soil mappers should duly consider theinferred geometric accuracy of the profile locations in their applications(Grimm and Behrens, 2010), since the soil observations andcovariates may not actually correspond (Cressie and Kornak, 2003) in both space and time (see Sect. 4, second paragraph).
As indicated, soil data considered in WoSIS have been analysed according toa wide range of analytical procedures and in different laboratories. Anindication of the measurement uncertainty is thus desired; soil-laboratory-specific Quality Management Systems (van Reeuwijk,1998), as well as laboratory proficiency-testing (PT, Magnusson andÖrnemark, 2014; Munzert et al., 2007; WEPAL, 2019), can provide this typeof information. Yet, calculation of laboratory-specific measurementuncertainty for a single method or multiple analytical methodswill require several measurement rounds (years of observation) and solidstatistical analyses. Overall, such detailed information is not availablefor the datasets submitted to the ISRIC data repository. Therefore, out ofnecessity, we have distilled the desired information from the PT literature(Kalra and Maynard, 1991; Rayment and Lyons, 2011; Rossel and McBratney,1998; van Reeuwijk, 1983; WEPAL, 2019), in so far as technically feasible.For example, accuracy for bulk density measurements, both for the directcore and the clod method, has been termed “low” (though not quantified) in arecent review (Al-Shammary et al., 2018); using expert knowledge,we have assumed this corresponds with an uncertainty (or variability,expressed as coefficient of variation) of 35 %. Alternatively, fororganic carbon content the mean variability was 17 % (with a range of 12 %to 42 %) and for “CEC (cation exchange capacity) buffered at pH 7” it was 18 % (range 13 % to 25 %)when multiple laboratories analyse a standard set of reference materialsusing similar operational methods (WEPAL, 2019). For soil pHmeasurements (log scale), we have expressed the uncertainty in terms of“±pH units”.
Importantly, the figures for measurement accuracy presented in Appendix Arepresent first approximations. They are based on the inter-laboratorycomparison of well-homogenised reference samples for a still relativelysmall range of soil types. These indicative figures should be refined oncelaboratory-specific and method-related accuracy (i.e. systematic and randomerror) information is provided for the shared soil data, e.g. byusing the procedures described by Eurachem (Magnusson andÖrnemark, 2014). Alternatively, this type of information may be refinedin the context of international laboratory PT networks, such as GLOSOLAN andWEPAL. Meanwhile, the present “first” estimates may already be considered tocalculate the accuracy of digital soil maps and of any interpretationsderived from them (e.g. maps of soil organic carbon stocks in support of theUNCCD Land Degradation Neutrality, LDN, effort).
The present snapshot includes standardised data for 196 498 profiles (Fig. 2), about twice the amount represented in the “July 2016” snapshot. Theseare represented by some 832 000 soil layers (or horizons). In total, thiscorresponds with over 5.8 million records that include both numeric (e.g.sand content, soil pH and cation exchange capacity) and class (e.g.WRB soil classification and horizon designation) properties. The namingconventions and standard units of measurement are provided in Appendix A,and the file structure is provided in Appendix B.
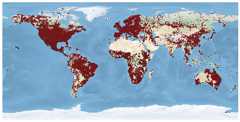
Figure 2Location of soil profiles provided in the “September 2019”snapshot of WoSIS; see Appendix C for the number and density of profiles by country.
Being a compilation of national soil data, the profiles were sampled over along period of time. The dates reported in the snapshot will reflect theyear the respective data were sampled and analysed: 1397 (0.7 %) profiles weresampled before 1920, 218 (0.1 %) between 1921 and 1940, 7,657 (3.9 %)between 1941 and 1960, 26,614 (13.5 %) between 1961 and 1980, 62 691 (31.9 %) between 1981 and 2000, and 31 084 (15.8 %) between 2001 and2020, while the date of sampling is unknown for 66 837 profiles (34.0 %).This information should be taken into consideration when linking the pointdata with environmental covariates, such as land use, in digital soilmapping.
The number of profiles per continent is highest for North America (73 604versus 63 066 in the “2016” snapshot), followed by Oceania (42 918 versus 235), Europe(35 311 versus 1,908), Africa (27 688 versus 17 153), South America (10 218versus 8790), Asia (6704 versus 3089) and Antarctica (9, no change). Theseprofiles come from 173 countries; the average density of observations is1.35 profiles per 1000 km2. The actual density of observations variesgreatly, both between countries (Appendix C) and within each country, withthe largest densities of “shared” profiles reported for Belgium (228 profiles per 1000 km2) and Switzerland (265 profiles per 1000 km2). There are still relatively few profiles for Central Asia, Southeast Asia, Central and Eastern Europe, Russia, and the northern circumpolarregion. The number of profiles by biome (R. J. Olson et al.,2001) or broad climatic region (Sayreet al., 2014), as derived from GIS overlays, is provided in Appendix D foradditional information.
There are more observations for the chemical data than the physical data(see Appendix A) and the number of observations generally decreases withdepth, largely depending on the objectives of the original soilsurveys. The interquartile range for maximum depth of soil sampled in thefield is 56–152 cm, with a median of 110 cm (mean=117 cm). In thisrespect, it should be noted that some specific purpose surveys onlyconsidered the topsoil (e.g. soil fertility surveys), while otherssystematically sampled soil layers up to depths exceeding 20 m.
Present gaps in the geographic distribution (Appendices C and D) and range ofsoil attribute data (Appendix A) will gradually be filled in the comingyears, though this largely depends on the willingness or ability of dataproviders to share (some of) their data for consideration in WoSIS. For thenorthern boreal and Arctic region, for example, ISRIC will regularly ingestnew profile data collated by the International Soil Carbon Network(ISCN, Malhotra et al., 2019). Alternatively, it should bereiterated that for some regions, such as Europe (e.g. EULUCAS topsoil database; see Tóth et al., 2013) and the state of Victoria(Australia), there are holdings in the ISRIC repository that may only beused and standardised for SoilGrids™ applications due to licencerestrictions. Consequently, the corresponding profiles (∼42 000) are neither shown in Fig. 2 nor are considered in the descriptivestatistics in Appendix C.
Upon their standardisation, the data are distributed through ISRIC's SDI(Spatial Data Infrastructure). This web platform is based on open-sourcetechnologies and open web-services (WFS, WMS, WCS, CSW) following OpenGeospatial Consortium (OGC) standards and is aimed specifically at handlingsoil data; our metadata are organised following standards of theInternational Organization for Standardization (ISO-28258, 2013)and are INSPIRE (2015) compliant. The three main components of theSDI are PostgreSQL+ PostGIS, GeoServer and GeoNetwork. Visualisation anddata download are done in GeoNetwork with resources from GeoServer(https://data.isric.org, last access: 12 September 2019). The third component is the PostgreSQLdatabase, with the spatial extension PostGIS, in which WoSIS resides; thedatabase is connected to GeoServer to permit data download from GeoNetwork.These processes are aimed at facilitating global data interoperability andciteability in compliance with FAIR principles: the data should be“findable, accessible, interoperable and reusable” (Wilkinson et al.,2016). With partners, steps are being taken towards the development ofa federated and ultimately interoperable spatial soil data infrastructure(GLOSIS) through which source data are served and updated by the respectivedata providers and made queryable according to a common SoilML standard(OGC, 2019).
The procedure for accessing the most current set of standardised soilprofile data (“wosis_latest”), either from R or QGIS usingWFS, is explained in a detailed tutorial (Rossiter, 2019). This dataset is dynamic; hence, it will grow when new point data are shared and processed,additional soil attributes are considered in the WoSIS workflow, and/or whenpossible corrections are required. Potential errors may be reported onlinevia a “Google group” so that they may be addressed in the dynamic version(register via:https://groups.google.com/forum/#!forum/isric-world-soil-informationlast access: 15 January 2020).
For consistent citation purposes, we provide static snapshots of the standardiseddata, in a tab-separated values format, with unique DOI's (digital objectidentifier); as indicated, this paper describes the second WoSIS snapshot.
The above procedures describe standardisation according to operationaldefinitions for soil properties. Importantly, it should be stressed herethat the ultimate, desired full harmonisation to an agreed reference methody, for example, “pHH2O,1:2.5 soil ∕ water solution” for all “pH1:xH2O” measurements, will first become feasible once the target method(y) for each property has been defined and subsequently accepted by theinternational soil community. A next step would be to collate and develop“comparative” datasets for each soil property, i.e. sets with samplesanalysed according to a given reference method (Yi) and thecorresponding national methods (Xj) for pedotransfer functiondevelopment. In practice, however, such relationships will often be soil type and region specific (see Appendix C in GlobalSoilMap, 2015).Alternatively, according to GLOSOLAN (Suvannang et al.,2018, p. 10) “comparable and useful soil information (at the global level)will only be attainable once laboratories agree to follow common standardsand norms”. In such a collaborative process, it will be essential toconsider the end user's requirements in terms of quality and applicabilityof the data for their specific purposes (i.e. fitness for intended use).Over the years, many organisations have individually developed and implementedanalytical methods and quality assurance systems that are well suited fortheir countries (e.g. Soil Survey Staff, 2014a) orregions (Orgiazzi et al., 2018) and thus, pragmatically, maynot be inclined to implement the anticipated GLOSOLAN standard analyticalmethods.
Snapshot “WoSIS_2019_September” is archivedfor long-term storage at ISRIC – World Soil Information, the World DataCentre for Soils (WDC-Soils) of the ISC (International Council for Science,formerly ICSU) World Data System (WDS). It is freely accessible athttps://doi.org/10.17027/isric-wdcsoils.20190901(Batjes et al., 2019). The zip file (154 Mb) includes a“readme first” file that describes key aspects of the dataset (see alsoAppendix B) with reference to the WoSIS Procedures Manual(Ribeiro et al., 2018), and the data itself in TSV format(1.8 Gb, decompressed) and GeoPackage format (2.2 Gb decompressed).
The second WoSIS snapshot provides consistent, standardised data for some196 000 profiles worldwide. However, as described, there are still importantgaps in terms of geographic distribution as well as the range of soil taxonomicunits and/or properties represented. These issues will be addressed infuture releases, depending largely on the success of our targeted requestsand searches for new data providers and/or partners worldwide.
We will increasingly consider data derived by soil spectroscopy and emerginginnovative methods. Further, long-term time series at defined locations willbe sought to support space–time modelling of soil properties, such aschanges in soil carbon stocks or soil salinity.
We provide measures for geographic accuracy of the point data, as well as afirst approximation for the uncertainty associated with theoperationally defined analytical methods. This information may be used toassess uncertainty in digital soil mapping and earth system modellingefforts that draw on the present set of point data.
Capacity building and cooperation among (inter)national soil institutes willbe necessary to create and share ownership of the soil information newlyderived from the shared data and to strengthen the necessary expertise andcapacity to further develop and test the world soil information serviceworldwide. Such activities may be envisaged within the broader framework ofthe Global Soil Partnership and emerging GLOSIS system.
Table A1Coding conventions and soil property names and theirdescription, units of measurement, inferred accuracy, and number of profilesand layers provided in the “WoSIS September 2019” snapshot. Soil propertiesare listed in alphabetical order using the property code.
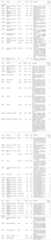
a Inferred accuracy (or uncertainty), rounded to the nearest 5 %,unless otherwise indicated (i.e. units for soil pH), as derived from thefollowing sources: Al-Shammary et al. (2018), Kalra and Maynard (1991),Rayment and Lyons (2011), Rossel and McBratney (1998), van Reeuwijk (1983),WEPAL (2019). These figures are first approximations that will be fine-tunedonce more specific results of laboratory proficiency tests, from nationalSoil Quality Management systems, become available.b Generally, the fine-earth fraction is defined as being<2 mm. Alternatively, an upper limit of 1 mm was used in the former SovietUnion and its satellite states (Katchynsky scheme). This has been indicatedin the file “wosis_201907_layers_chemical.tsv” and “wosis_201907_layer_physicals.tsv” for those soil properties where thisdifferentiation is important (see “sample pretreatment” in string“xxxx_method” in Appendix B).c Provided only when the sum of clay, silt and sand fraction is≥90 % and≤100 %.d Where available, the “cleaned” (original) layer and horizondesignation is provided for general information; these codes have not beenstandardised as they vary widely between different classification systems(Bridges, 1993; Gerasimova et al., 2013).When horizon designations are not provided in the source databases, we haveflagged all layers with an upper depth given as being negative (e.g.−10 to0 cm under pre-1993 conventions; see text and the WoSIS ProceduresManual 2018; Ribeiro et al., 2018, p. 24, footnote 9) in the source databases as likely being“litter” layers. n/a – not applicable
This Appendix describes the structure of the data files presented in the“September 2019” WoSIS snapshot:
wosis_201909_attributes.tsv,
wosis_201909_profiles.tsv,
wosis_201909_layers_chemical.tsv,
wosis_201909_layer_physicals.tsv.
wosis_201909_attributes.tsv. This file lists the four to six letter codes for each attribute, whetherthe attribute is a site or horizon property, the unit of measurement, thenumber of profiles and layers represented in the snapshot, and abrief description of each attribute, as well as the inferred uncertainty foreach property (Appendix A).
wosis_201909_profiles.tsv. This file contains the unique profile ID (i.e. primary key), the source ofthe data, country ISO code and name, accuracy of geographical coordinates,latitude and longitude (WGS 1984), point geometry of the location of theprofile, and the maximum depth of soil described and sampled, as well as informationon the soil classification system and edition (Table B1). Depending on the soilclassification system used, the number of fields will vary. For example, forthe World Soil Reference Base (WRB) system these are as follows:publication_year (i.e. version), reference_soil_group_code, reference_soil_group_name, and the name(s) of the prefix(primary) qualifier(s) and suffix (supplementary) qualifier(s). Theterms principal qualifier and supplementary qualifier are currently used(IUSS Working Group WRB, 2015); earlier WRB versions used prefixand suffix for this (e.g. IUSS Working Group WRB, 2006).Alternatively, for USDA Soil Taxonomy, the version (year), order, suborder,great group and subgroup can be accommodated (Soil Survey Staff,2014b). Inherently, the number of records filled will vary between (andwithin) the various source databases.
wosis_201909_layer_chemical.tsv andwosis_201909_layer_physical.tsv. Data for the various layers (or horizons) are presented in two separate files in view of their size (i.e. one for the chemical and one for the physical soil properties). The file structure is described in Table B1.
Table B1List of properties described in filewosis_201909_profiles.tsv,wosis_201909_layers_chemical.tsv andwosis_201909_layer_physicals.tsv.
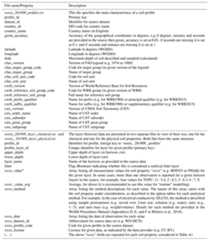
∗ Name of attribute (“xxxx”) as defined under “code” in filewosis_201909_attributes.tsv.
Format. All fields in the above files are delimited by tab, with double quotationmarks as text delimiters. File coding is according to the UTF-8 unicodetransformation format.
Using the data. The above TSV files can easily be imported into an SQL database orstatistical software such as R, after which they may be joined using theunique profile_id. Guidelines for handling and querying thedata are provided in the WoSIS Procedures Manual (Ribeiro et al., 2018, pp. 45–48); see also the detailed tutorial by Rossiter (2019).
Table C1Number of profiles by country and continent.

* Disputed territories. Country names and areas are based on the GlobalAdministrative Layers (GAUL) database; seehttp://www.fao.org/geonetwork/srv/en/metadata.show?id=12691 (last access: 8 January 2020).
NB led the DATA (WoSIS) project and wrote the body of the paper. ER provided special expertise on database management and AO on soil analytical methods. All co-authors contributed to the writing and revision of this paper.
The authors declare that they have no conflict of interest.
The development of WoSIS has been made possible thanks to the contributionsand shared knowledge of a steadily growing number of data providers,including soil survey organisations, research institutes and individualexperts, for which we are grateful; for an overview, please seehttps://www.isric.org/explore/wosis/wosis-contributing-institutions-and-experts (last access: 8 January 2020).We thank our colleagues Laura Poggio, Luis de Sousa and Bas Kempen for theirconstructive comments on a “pre-release” of the snapshot data. Further, themanuscript benefitted from the constructive comments provided by the tworeviewers.
ISRIC – World Soil Information, legally registered as the International Soil Reference and Information Centre, receives core funding from the Dutch Government.
This paper was edited by David Carlson and reviewed by Alessandro Samuel-Rosa and one anonymous referee.
Al-Shammary, A. A. G., Kouzani, A. Z., Kaynak, A., Khoo, S. Y., Norton, M.,and Gates, W.: Soil Bulk Density Estimation Methods: A Review, Pedosphere,28, 581–596,https://doi.org/10.1016/S1002-0160(18)60034-7,2018.
Arrouays, D., Leenaars, J. G. B., Richer-de-Forges, A. C., Adhikari, K.,Ballabio, C., Greve, M., Grundy, M., Guerrero, E., Hempel, J., Hengl, T.,Heuvelink, G., Batjes, N., Carvalho, E., Hartemink, A., Hewitt, A., Hong,S.-Y., Krasilnikov, P., Lagacherie, P., Lelyk, G., Libohova, Z., Lilly, A.,McBratney, A., McKenzie, N., Vasquez, G. M., Mulder, V. L., Minasny, B.,Montanarella, L., Odeh, I., Padarian, J., Poggio, L., Roudier, P., Saby, N.,Savin, I., Searle, R., Solbovoy, V., Thompson, J., Smith, S., Sulaeman, Y.,Vintila, R., Rossel, R. V., Wilson, P., Zhang, G.-L., Swerts, M., Oorts, K.,Karklins, A., Feng, L., Ibelles Navarro, A. R., Levin, A., Laktionova, T.,Dell'Acqua, M., Suvannang, N., Ruam, W., Prasad, J., Patil, N., Husnjak, S.,Pásztor, L., Okx, J., Hallet, S., Keay, C., Farewell, T., Lilja, H.,Juilleret, J., Marx, S., Takata, Y., Kazuyuki, Y., Mansuy, N., Panagos, P.,Van Liedekerke, M., Skalsky, R., Sobocka, J., Kobza, J., Eftekhari, K.,Alavipanah, S. K., Moussadek, R., Badraoui, M., Da Silva, M., Paterson, G.,Gonçalves, M. d. C., Theocharopoulos, S., Yemefack, M., Tedou, S.,Vrscaj, B., Grob, U., Kozák, J., Boruvka, L., Dobos, E., Taboada, M.,Moretti, L., and Rodriguez, D.: Soil legacy data rescue via GlobalSoilMapand other international and national initiatives, GeoResJ, 14, 1–19,https://doi.org/10.1016/j.grj.2017.06.001, 2017.
Baroni, G., Zink, M., Kumar, R., Samaniego, L., and Attinger, S.: Effects of uncertainty in soil properties on simulated hydrological states and fluxes at different spatio-temporal scales, Hydrol. Earth Syst. Sci., 21, 2301–2320,https://doi.org/10.5194/hess-21-2301-2017, 2017.
Baritz, R., Erdogan, H., Fujii, K., Takata, Y., Nocita, M., Bussian, B., Batjes, N. H., Hempel, J., Wilson, P., and Vargas, R.: Harmonization of methods, measurements and indicators for the sustainable management and protection of soil resources (Providing mechanisms for the collation, analysis and exchange of consistent and comparable global soil data and information), Global Soil Partnership, FAO, 44 pp., 2014.
Batjes, N. H.: Harmonized soil profile data for applications at global andcontinental scales: updates to the WISE database, Soil Use Manage.,25, 124–127https://doi.org/10.1111/j.1475-2743.2009.00202.x,(supplemental information:https://www.isric.org/sites/default/files/isric_report_2008_02.pdf, last access: 8 January 2020), 2009.
Batjes, N. H.: Harmonised soil property values for broad-scale modelling(WISE30sec) with estimates of global soil carbon stocks, Geoderma, 269,61–68,https://doi.org/10.1016/j.geoderma.2016.01.034, 2016.
Batjes, N. H., Ribeiro, E., van Oostrum, A., Leenaars, J., Hengl, T., and Mendes de Jesus, J.: WoSIS: providing standardised soil profile data for the world, Earth Syst. Sci. Data, 9, 1–14,https://doi.org/10.5194/essd-9-1-2017, 2017.
Batjes, N. H., Ribeiro, E., and van Oostrum, A. J. M.: Standardised soilprofile data for the world (WoSIS snapshot – September 2019), ISRICWDC-Soils,https://doi.org/10.17027/isric-wdcsoils.20190901,2019.
Bridges, E. M.: Soil horizon designations: past use and future prospects,Catena, 20, 363–373,https://doi.org/10.1016/S0341-8162(05)80002-5, 1993.
Cressie, N. and Kornak, J.: Spatial Statistics in the Presence of LocationError with an Application to Remote Sensing of the Environment, Stat.Sci., 18, 436–456, https://projecteuclid.org:443/euclid.ss/1081443228, 2003.
Dai, Y., Shangguan, W., Wei, N., Xin, Q., Yuan, H., Zhang, S., Liu, S., Lu, X., Wang, D., and Yan, F.: A review of the global soil property maps for Earth system models, SOIL, 5, 137–158,https://doi.org/10.5194/soil-5-137-2019, 2019.
FAO: Guidelines for the description of soils, FAO, Rome, 1977.
FAO: Guidelines for soil description (4th Edn.), FAO, Rome, 97 pp., 2006.
FAO, IIASA, ISRIC, ISSCAS, and JRC: Harmonized World Soil Database (version1.2), prepared by: Nachtergaele, F. O., van Velthuizen, H., Verelst, L., Wiberg, D.,Batjes, N. H., Dijkshoorn, J. A., van Engelen, V. W. P., Fischer, G., Jones, A., Montanarella,L., Petri, M., Prieler, S., Teixeira, E., and Shi, X., Food and AgricultureOrganization of the United Nations (FAO), International Institute forApplied Systems Analysis (IIASA), ISRIC – World Soil Information, Instituteof Soil Science – Chinese Academy of Sciences (ISSCAS), Joint ResearchCentre of the European Commission (JRC), Laxenburg, Austria, 2012.
FAO-ISRIC: Guidelines for soil description, 3rd Edn., Rev., FAO, Rome,70 pp., 1986.
Finke, P.: Quality assessment of digital soil maps: producers and usersperspectives, in: Digital soil mapping: An introductory perspective, editedby: Lagacherie, P., McBratney, A., and Voltz, M., Elsevier, Amsterdam,523–541, 2006.
Folberth, C., Skalsky, R., Moltchanova, E., Balkovic, J., Azevedo, L. B.,Obersteiner, M., and van der Velde, M.: Uncertainty in soil data canoutweigh climate impact signals in global crop yield simulations, Nat. Commun., 7, 11872,https://doi.org/10.1038/ncomms11872, 2016.
Gerasimova, M. I., Lebedeva, I. I., and Khitrov, N. B.: Soil horizondesignation: State of the art, problems, and proposals, Eurasian SoilSci., 46, 599–609,https://doi.org/10.1134/S1064229313050037, 2013.
GlobalSoilMap: Specifications Tiered GlobalSoilMap products (Release 2.4),52 pp., 2015.
Grimm, R. and Behrens, T.: Uncertainty analysis of sample locations withindigital soil mapping approaches, Geoderma, 155, 154–163,https://doi.org/10.1016/j.geoderma.2009.05.006, 2010.
GSP Pillar4 Working Group: Towards the implementation of GloSIS through a Country Soil InformationSystems (CountrySIS) Framework (Concept Note, draft), available at:http://www.fao.org/global-soil-partnership/pillars-action/4-information-data/glosis/en/,last access: 26 November 2018.
Guevara, M., Olmedo, G. F., Stell, E., Yigini, Y., Aguilar Duarte, Y., Arellano Hernández, C., Arévalo, G. E., Arroyo-Cruz, C. E., Bolivar, A., Bunning, S., Bustamante Cañas, N., Cruz-Gaistardo, C. O., Davila, F., Dell Acqua, M., Encina, A., Figueredo Tacona, H., Fontes, F., Hernández Herrera, J. A., Ibelles Navarro, A. R., Loayza, V., Manueles, A. M., Mendoza Jara, F., Olivera, C., Osorio Hermosilla, R., Pereira, G., Prieto, P., Ramos, I. A., Rey Brina, J. C., Rivera, R., Rodríguez-Rodríguez, J., Roopnarine, R., Rosales Ibarra, A., Rosales Riveiro, K. A., Schulz, G. A., Spence, A., Vasques, G. M., Vargas, R. R., and Vargas, R.: No silver bullet for digital soil mapping: country-specific soil organic carbon estimates across Latin America, SOIL, 4, 173–193,https://doi.org/10.5194/soil-4-173-2018, 2018.
Hendriks, C. M. J., Stoorvogel, J. J., and Claessens, L.: Exploring thechallenges with soil data in regional land use analysis, Agr.Syst., 144, 9–21,https://doi.org/10.1016/j.agsy.2016.01.007, 2016.
Hengl, T., Leenaars, J. G. B., Shepherd, K. D., Walsh, M. G., Heuvelink, G.B. M., Mamo, T., Tilahun, H., Berkhout, E., Cooper, M., Fegraus, E.,Wheeler, I., and Kwabena, N. A.: Soil nutrient maps of Sub-Saharan Africa:assessment of soil nutrient content at 250 m spatial resolution usingmachine learning, Nutr. Cycl. Agroecosys., 109, 77–102,https://doi.org/10.1007/s10705-017-9870-x, 2017a.
Hengl, T., Mendes de Jesus, J., Heuvelink, G. B. M., Ruiperez Gonzalez, M.,Kilibarda, M., Blagotić, A., Shangguan, W., Wright, M. N., Geng, X.,Bauer-Marschallinger, B., Guevara, M. A., Vargas, R., MacMillan, R. A.,Batjes, N. H., Leenaars, J. G. B., Ribeiro, E., Wheeler, I., Mantel, S., andKempen, B.: SoilGrids250m: Global gridded soil information based on machinelearning, PLoS ONE, 12, e0169748,https://doi.org/10.1371/journal.pone.0169748, 2017b.
Heuvelink, G. B. M.: Uncertainty quantification of GlobalSoilMap productsin: GlobalSoilMap. Basis of the Global Spatial Soil Information System,edited by: Arrouays, D., McKenzie, N., Hempel, J., Forges, A. R. D., andMcBratney, A., Taylor & Francis Group, London, UK, 335–240, 2014.
Heuvelink, G. B. M. and Brown, J. D.: Towards a soil information system foruncertain soil data in: Digital soil mapping: An introductory perspective,edited by: Lagacherie, P., McBratney, A., and Voltz, M., Elsevier,Amsterdam, 97–106, 2006.
INSPIRE: Data specifications – Infrastructure for spatial information in theEuropean Community, available at:http://inspire.ec.europa.eu/index.cfm/pageid/2 (last access: 25 April 2016),2015.
ISO-28258: Soil quality – Digital exchange of soil-related data, available at:https://www.iso.org/obp/ui#iso:std:iso:28258:ed-1:v1:en (last access: 31 January 2018), 2013.
ISRIC: Data and Software Policy: available at:http://www.isric.org/sites/default/files/ISRIC_Data_Policy_2016jun21.pdf(last accesss: 15 May 2019), 2016.
IUSS Working Group WRB: World Reference Base for Soil Resources (2nd Edn.),FAO, Rome, World Soil Resources Report 103, 145 pp., 2006.
IUSS Working Group WRB: World Reference Base for soil resources 2014 –International soil classification system for naming soils and creatinglegends for soil maps (update 2015), Global Soil Partnership, InternationalUnion of Soil Sciences, and Food and Agriculture Organization of the UnitedNations, Rome, World Soil Resources Reports 106, 182 pp., 2015.
Kalra, Y. P. and Maynard, D. G.: Methods manual for forest soil and plantanalysis, Forestry Canada, Edmonton (Alberta), 116 pp., 1991.
Leenaars, J. G. B., van Oostrum, A. J. M., and Ruiperez Gonzalez, M.: AfricaSoil Profiles Database: A compilation of georeferenced and standardisedlegacy soil profile data for Sub Saharan Africa (version 1.2), Africa SoilInformation Service (AfSIS) and ISRIC – World Soil Information, Wageningen,Report 2014/01, 160 pp., 2014.
Leenaars, J. G. B., Claessens, L., Heuvelink, G. B. M., Hengl, T., RuiperezGonzález, M., van Bussel, L. G. J., Guilpart, N., Yang, H., and Cassman,K. G.: Mapping rootable depth and root zone plant-available water holdingcapacity of the soil of sub-Saharan Africa, Geoderma, 324, 18–36,https://doi.org/10.1016/j.geoderma.2018.02.046, 2018.
Lutz, F., Stoorvogel, J. J., and Müller, C.: Options to model theeffects of tillage onN2O emissions at the global scale, Ecol.Model., 392, 212–225, 2019.
Magnusson, B. and Örnemark, U.: The Fitness for Purpose of AnalyticalMethods – A Laboratory Guide to Method Validation and Related Topics (2ndEdn.), available at:http://www.eurachem.org (last access: 8 September 2019), 2014.
Maire, V., Wright, I. J., Prentice, I. C., Batjes, N. H., Bhaskar, R., vanBodegom, P. M., Cornwell, W. K., Ellsworth, D., Niinemets, Ü., Ordonez,A., Reich, P. B., and Santiago, L. S.: Global effects of soil and climate onleaf photosynthetic traits and rates, Global Ecol. Biogeogr., 24,706–715,https://doi.org/10.1111/geb.12296, 2015.
Malhotra, A., Todd-Brown, K., Nave, L. E., Batjes, N. H., Holmquist, J. R.,Hoyt, A. M., Iversen, C. M., Jackson, R. B., Lajtha, K., Lawrence, C.,Vindušková, O., Wieder, W., Williams, M., Hugelius, G., and Harden,J.: The landscape of soil carbon data: emerging questions, synergies anddatabases, Prog. Phys. Geog., 43, 707–719,https://doi.org/10.1177/0309133319873309, 2019.
Moulatlet, G. M., Zuquim, G., Figueiredo, F. O. G., Lehtonen, S., Emilio,T., Ruokolainen, K., and Tuomisto, H.: Using digital soil maps to inferedaphic affinities of plant species in Amazonia: Problems and prospects,Ecol. Evol., 7, 8463–8477,https://doi.org/10.1002/ece3.3242, 2017.
Munzert, M., Kießling, G., Übelhör, W., Nätscher, L., andNeubert, K.-H.: Expanded measurement uncertainty of soil parameters derivedfrom proficiency-testing data, J. Plant Nutr. Soil Sci.,170, 722–728,https://doi.org/10.1002/jpln.200620701, 2007.
Nave, L., Johnson, K., van Ingen, C., Agarwal, D., Humphrey, M., and Beekwilder, N.: ISCN Database V3-1,https://doi.org/10.17040/ISCN/1305039, 2017.
OGC: Soil Data IE (Interoperability Experiment), available at:https://www.opengeospatial.org/projects/initiatives/soildataie, last access: 14 June 2019.
Olson, D. M., Dinerstein, E., Wikramanayake, E. D., Burgess, N. D., Powell,G. V. N., Underwood, E. C., D'amico, J. A., Itoua, I., Strand, H. E.,Morrison, J. C., Loucks, C. J., Allnutt, T. F., Ricketts, T. H., Kura, Y.,Lamoreux, J. F., Wettengel, W. W., Hedao, P., and Kassem, K. R.: TerrestrialEcoregions of the World: A New Map of Life on Earth: A new global map ofterrestrial ecoregions provides an innovative tool for conservingbiodiversity, BioScience, 51, 933–938,https://doi.org/10.1641/0006-3568(2001)051[0933:TEOTWA]2.0.CO;2, 2001.
Olson, R. J., Johnson, K. R., Zheng, D. L., and Scurlock, J. M. O.: Globaland regional ecosystem modelling: databases of model drivers and validationmeasurements, Oak Ridge National Laboratory, Oak Ridge, ORNL/TM-2001/196, 95pp., 2001.
Orgiazzi, A., Ballabio, C., Panagos, P., Jones, A., andFernández-Ugalde, O.: LUCAS Soil, the largest expandable soil datasetfor Europe: a review, Eur. J. Soil Sci., 69, 140–153,https://doi.org/10.1111/ejss.12499, 2018.
Rayment, E. R. and Lyons, D. J.: Soil chemical methods – Australasia, CSIROPublishing, 495 pp., 2011.
Ribeiro, E., Batjes, N. H., and Van Oostrum, A. J. M.: World SoilInformation Service (WoSIS) – Towards the standardization and harmonizationof world soil data, Procedures Manual 2018, ISRIC – World Soil Information,Wageningen, ISRIC Report 2018/01, 166 pp., 2018.
Rossel, R. A. V. and McBratney, A. B.: Soil chemical analytical accuracyand costs: implications from precision agriculture, Aust. J.Exp. Agr., 38, 765–775,https://doi.org/10.1071/EA97158, 1998.
Rossiter, D.: Accessing WoSIS from R – “Snapshot” version, available at:https://www.isric.org/sites/default/files/WoSIS_Snapshot_With_R_2.pdf (last access: 30 January 2020), 2019.
Sanderman, J., Hengl, T., and Fiske, G. J.: Soil carbon debt of 12,000 yearsof human land use, P. Natl. Acad. Sci. USA, 36, 9575–9580,https://doi.org/10.1073/pnas.1706103114, 2017.
Sayre, R., Dangermond, J., Frye, C., Vaughan, R., Aniello, P., Breyer, S.,Cribbs, D., Hopkins, D., Nauman, R., Derrenbacher, W., Burton, D., Grosse,A., True, D., Metzger, M., Hartmann, J., Moosdorf, N., Dürr, H.,Paganini, M., DeFourny, P., Arino, O., and Maynard, S.: A New Map of GlobalEcological Land Units – An Ecophysiographic Stratification Approach,Association of American Geographers, Washington DC, 46 pp., 2014.
Schoeneberger, P. J., Wysocki, D. A., Benham, E. C., and Soil Survey Staff:Field book for describing and sampling soils (ver. 3.0), National SoilSurvey Center Natural Resources Conservation Service, U.S. Department ofAgriculture, Lincoln (NE), 2012.
Soil Survey Staff: Soil Survey Laboratory Information Manual (Ver. 2.0),National Soil Survey Center, Soil Survey Laboratory, USDA-NRCS, Lincoln(NE), Soil Survey Investigation Report No. 45, 506 pp., 2011.
Soil Survey Staff: Kellogg Soil Survey Laboratory Methods Manual, Version5.0, edited by: Burt, R. and Soil Survey Staff, U.S. Department of Agriculture,Natural Resources Conservation Service, Lincoln (Nebraska), 1001 pp., 2014a.
Soil Survey Staff: Keys to Soil Taxonomy, 12th Edn., USDA-Natural ResourcesConservation Service, Washington, DC, 2014b.
Suvannang, N., Hartmann, C., Yakimenko, O., Solokha, M., Bertsch, F., andMoody, P.: Evaluation of the First Global Soil Laboratory Network (GLOSOLAN)online survey for assessing soil laboratory capacities, Global SoilPartnership (GSP)/Food and Agriculture Organization of the United Nations(FAO), Rome, GLOSOLAN/18/Survey Report, 54 pp., 2018.
Terhoeven-Urselmans, T., Shepherd, K. D., Chabrillat, S., and Ben-Dor, E.:Application of a global soil spectral library as tool for soil qualityassessment in Sub-Saharan Africa, A EUFAR Workshop on QuantitativeApplications of Soil Spectroscopy, p. 15, 5–16 April 2010.
Tóth, G., Jones, A., and Montanarella, L.: LUCAS Topsoil survey:methodology, data and results Land Resource Management Unit – Soil Action,European Commission Joint Research Centre Institute for Environment andSustainability, 141 pp., 2013.
USDA-NCSS: National Cooperative Soil Survey (NCSS) Soil CharacterizationDatabase, United States Department of Agriculture, Natural ResourcesConservation Service, Lincoln, 2018.
van Engelen, V. W. P. and Dijkshoorn, J. A.: Global and National Soils andTerrain Digital Databases (SOTER) - Procedures manual (Ver. 2.0), IUSS,ISRIC and FAO, Wageningen, ISRIC Report 2013/04, 198 pp., 2013.
van Ittersum, M. K., Cassman, K. G., Grassini, P., Wolf, J., Tittonell, P.,and Hochman, Z.: Yield gap analysis with local to global relevance – Areview, Field Crop. Res., 143, 4–17, 2013.
Van Looy, K., Bouma, J., Herbst, M., Koestel, J., Minasny, B., Mishra, U.,Montzka, C., Nemes, A., Pachepsky, Y., Padarian, J., Schaap, M., Tóth,B., Verhoef, A., Vanderborght, J., van der Ploeg, M., Weihermüller, L.,Zacharias, S., Zhang, Y., and Vereecken, H. C. R. G.: Pedotransfer functionsin Earth system science: challenges and perspectives, Rev. Geophys.,55, 1199–1256,https://doi.org/10.1002/2017RG000581, 2017.
van Reeuwijk, L. P.: On the way to improve international soil classificationand correlation: the variability of soil analytical data, ISRIC, Wageningen,Annual Report 1983, 7–13, 1983.
van Reeuwijk, L. P.: Guidelines for quality management in soil and plantlaboratories, FAO, Rome, 143 pp., 1998.
Viscarra Rossel, R. A., Behrens, T., Ben-Dor, E., Brown, D. J., Demattê,J. A. M., Shepherd, K. D., Shi, Z., Stenberg, B., Stevens, A., Adamchuk, V.,Aïchi, H., Barthès, B. G., Bartholomeus, H. M., Bayer, A. D.,Bernoux, M., Böttcher, K., Brodský, L., Du, C. W., Chappell, A.,Fouad, Y., Genot, V., Gomez, C., Grunwald, S., Gubler, A., Guerrero, C.,Hedley, C. B., Knadel, M., Morrás, H. J. M., Nocita, M., Ramirez-Lopez,L., Roudier, P., Campos, E. M. R., Sanborn, P., Sellitto, V. M., Sudduth, K.A., Rawlins, B. G., Walter, C., Winowiecki, L. A., Hong, S. Y., and Ji, W.:A global spectral library to characterize the world's soil, Earth-Sci.Rev., 155, 198–230,https://doi.org/10.1016/j.earscirev.2016.01.012, 2016.
WEPAL: ISE Reference Material – A list with all available ISE referencematerial samples, WEPAL (Wageningen Evaluating Programmes for AnalyticalLaboratories), Wageningen, 110 pp., 2019.
Wilkinson, M. D., Dumontier, M., Aalbersberg, I. J., Appleton, G., Axton,M., Baak, A., Blomberg, N., Boiten, J.-W., da Silva Santos, L. B., Bourne,P. E., Bouwman, J., Brookes, A. J., Clark, T., Crosas, M., Dillo, I., Dumon,O., Edmunds, S., Evelo, C. T., Finkers, R., Gonzalez-Beltran, A., Gray, A.J. G., Groth, P., Goble, C., Grethe, J. S., Heringa, J., 't Hoen, P. A. C.,Hooft, R., Kuhn, T., Kok, R., Kok, J., Lusher, S. J., Martone, M. E., Mons,A., Packer, A. L., Persson, B., Rocca-Serra, P., Roos, M., van Schaik, R.,Sansone, S.-A., Schultes, E., Sengstag, T., Slater, T., Strawn, G., Swertz,M. A., Thompson, M., van der Lei, J., van Mulligen, E., Velterop, J.,Waagmeester, A., Wittenburg, P., Wolstencroft, K., Zhao, J., and Mons, B.:The FAIR Guiding Principles for scientific data management and stewardship,Scientific Data, 3, 160018,https://doi.org/10.1038/sdata.2016.18, 2016.
- Abstract
- Introduction
- WoSIS workflow
- Data screening, quality control and standardisation
- Spatial distribution of soil profiles and number of observations
- Distributing the standardised data
- Discussion
- Data availability
- Conclusions
- Appendix A
- Appendix B: Structure of the “September 2019” WoSIS snapshot
- Appendix C
- Appendix D: Distribution of soil profiles by eco-region and by biome
- Author contributions
- Competing interests
- Acknowledgements
- Financial support
- Review statement
- References
- Abstract
- Introduction
- WoSIS workflow
- Data screening, quality control and standardisation
- Spatial distribution of soil profiles and number of observations
- Distributing the standardised data
- Discussion
- Data availability
- Conclusions
- Appendix A
- Appendix B: Structure of the “September 2019” WoSIS snapshot
- Appendix C
- Appendix D: Distribution of soil profiles by eco-region and by biome
- Author contributions
- Competing interests
- Acknowledgements
- Financial support
- Review statement
- References