the Creative Commons Attribution 4.0 License.
the Creative Commons Attribution 4.0 License.
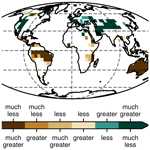
Tipping the ENSO into a permanent El Niño can trigger state transitions in global terrestrial ecosystems
Some large-scale components of the Earth's climate systemhave been identified as policy-relevant “tipping elements”, meaning thatanthropogenic forcing and perturbations may push them across a tipping pointthreshold, with potential global scale impact on ecosystems and concomitantenvironmental and social phenomena. A pronounced change in the amplitudeand/or frequency of the El Niño–Southern Oscillation (ENSO) is amongsuch tipping elements. Here, we use the Planet Simulator (PlaSim), an Earthsystem model of intermediate complexity, to investigate the potential impacton global climate and terrestrial ecosystems of shifting the current dynamicsof the ENSO into a permanent El Niño. When forced with sea surfacetemperature (SST) derived from observations, the PlaSim model yields arealistic representation of large-scale climatological patterns, includingrealistic estimates of the global energy and water balances, and grossprimary productivity (GPP). In a permanent El Niño state, we foundsignificant differences in the global distribution of water and energyfluxes, and associated impacts on GPP, indicating that vegetation productiondecreases in the tropics, whereas it increases in temperate regions. Weidentify regions in which these El Niño-induced changes are consistentwith potential state transitions in global terrestrial ecosystems, includingpotential greening of western North America, dieback of the Amazonrainforest, and further aridification of south-eastern Africa and Australia.
- Article
(8281 KB) - Full-text XML
- Supplement
(7769 KB) - BibTeX
- EndNote
Earth's current climatology can be considered a steady state of anon-equilibrium system(Kleidon, 2010) in which constant influx ofsolar radiation and internal feedback mechanisms stall equilibrium, so thatproperties of the climate system can remain relatively close to mean values.However, it is becoming increasingly clear that the Earth's climate system asa whole, as well as some of its large-scale components or subsystems, havetipping points (i.e. critical thresholds in forcing and/or a feature of thesystem) at which the system shifts from one state (e.g. current climate) toanother (e.g. future climate)(Lenton et al., 2008;Scheffer et al., 2009).Perturbations related to human-induced climate change could push severalEarth's subsystems past a tipping point(Lenton, 2011;Barnosky et al., 2012), potentially changing the modes of natural climaticvariability(Cai et al., 2015).
Lenton et al. (2008) identified several tipping elements in theEarth's climate system. A tipping element is a large-scale component of theEarth system that may pass over a tipping point with the potential to alterglobal climate. A pronounced change in the amplitude and/or frequency of theEl Niño–Southern Oscillation (ENSO), including its shift into apermanent El Niño state, is among the policy-relevant tipping elementsidentified byLenton et al. (2008). Although there is no definitiveevidence at present for specific changes in ENSO behaviour in response toclimate change(McPhaden et al., 2006;Cai et al., 2015), global climate modellingstudies do indicate the possibility of ENSO intensification during the 21stcentury due to anthropogenic forcing(Timmermann et al., 1999;Guilyardi, 2006). Greenhouse warming in climate projections can lead tomore frequent El Niño events, because it diminishes temperature gradientsin the eastern equatorial Pacific Ocean, which facilitate the changes inconvection zones that are observed during this phenomenon(Cai et al., 2014;Latif et al., 2015).
Through atmospheric teleconnections, ENSO exerts strong effects on manifoldenvironmental and social systems at global to regional scales(Diaz and Markgraf, 2000;Cai et al., 2015;Yeh et al., 2018;McPhaden et al., 2006), includingmajor implications for the functioning of terrestrial ecosystems(Holmgren et al., 2001). For instance, in Amazonia, temperature andprecipitation changes associated with ENSO have been found to alter the netcarbon flux to the atmosphere and seasonal inundation of floodplains(Foley et al., 2002). Moreover, El Niño-induced droughts in Amazoniaincrease the likelihood of forest fires that modify landscape features andhave top-down effects that damage not only vegetation but also faunalpopulations(Alencar et al., 2006). Similarly, in Australia's drylands,ENSO is a modulator of biomass removal through fires and grazing(Holmgren et al., 2006). Such land-use impacts are particularlyimportant in places where the population largely depends on agriculture, suchas south-eastern Africa, where ENSO also affects rainfall patterns(Indeje et al., 2000). Therefore, and despite the inherent uncertainty ofclimate change projections, the potential impact of shifting the state of theENSO is of global concern(Lenton et al., 2008;Cai et al., 2014).Advancing the quantitative understanding of the range of expected physicalclimate changes (let alone tipping events) and their potential impact ondifferent sectors and/or regions is crucial for decision-making related toglobal change(Lenton and Ciscar, 2013;Zebiak et al., 2015).
Here, we used the Planet Simulator(PlaSim; Fraedrich et al., 2005a,b) to investigate thepotential impact on global climate of shifting the dynamics of the ENSO intoa permanent El Niño state and examine the potential consequences of thistipping event on global terrestrial ecosystems. Our aim was to see whatEarth's climate could be if the climatology of sea surface temperature (SST)were that observed during a warm phase of ENSO. Thus, we simulated a controlscenario and compared it to current climate using data sets derived fromobservations, in order to understand the model's limitations. Then, we set upa permanent El Niño experiment and compared it to said controlsimulation, focusing on the impacts on water deficit and terrestrialecosystems. We also briefly discuss how this permanent El Niño experimentrelates to data and simulations from the warm Pliocene period, when it hasbeen suggested that sustained El Niño conditions occurred(Wara et al., 2005). The exploratory nature of our modelling approachis more about identifying potential impacts than about estimating theirlikelihood.
PlaSim is a climate model of intermediate complexity. This kind of model iswell suited for our goals because of its ability to parsimoniously simulatefeedback mechanisms between many components of the climate system overlong-term simulation ensembles covering a global domain(Claussen et al., 2002). The dynamical core of PlaSim is based on PUMA(Fraedrich et al., 2005c), an atmospheric general circulation model thatsolves the moist primitive equations representing the conservation ofmomentum, mass, and energy in the Earth's atmosphere. It uses a verticalσ coordinate and the spectral transform method with triangulartruncation over a sphere(Orszag, 1970;Hoskins and Simmons, 1975).Unresolved subgrid-scale processes such as radiation, moist and dryconvection, large-scale precipitation, surface fluxes, and vertical andhorizontal diffusion are parameterized(Lunkeit et al., 2011).
The atmospheric model is coupled to a five-layer land surface model thatincludes parameterizations for soil temperature, snow cover, and abucket-type runoff transport scheme(Sausen et al., 1994). A dynamicvegetation model (SimBA) included in PlaSim allows to representvegetation–atmosphere feedback mechanisms through climate-induced changes inlarge-scale surface parameters such as albedo, roughness, and water-holdingcapacity(Kleidon, 2006). The ocean can be represented throughprescribed climatological values of SST or using dynamical models(Fraedrich, 2012;Semtner Jr., 1976). For more details about themodel description, we refer the reader toFraedrich et al. (2005a) andLunkeit et al. (2011).
PlaSim has been successfully used to study the evolution of the Earth'sclimate system under different physical scenarios and forcings. One of thefirst applications of PlaSim was to estimate the maximum possible influenceof vegetation on the global climate through simulation of two extremescenarios: “green planet” and “desert world”(Fraedrich et al., 2005b).More recent applications have studied the planetary climate system's responseto, for instance, vegetation–atmosphere feedback mechanisms(Dekker et al., 2010;Bathiany et al., 2012), large-scalechanges in orography(Garreaud et al., 2010;Schmittner et al., 2011), andvariations in astronomical forcings(Boschi et al., 2013;Linsenmeier et al., 2015). The model has also been used for paleoclimatestudies(Henrot et al., 2009) and snowball Earth experiments(Spiegl et al., 2015;Lucarini et al., 2013). Some of these studieshave also explored the existence of alternative equilibrium states of theplanetary climate system, as well as the mechanisms behind potential statetransitions(Dekker et al., 2010;Bathiany et al., 2012;Boschi et al., 2013;Linsenmeier et al., 2015).
For all simulations, PlaSim was implemented with a spectral horizontalresolution of T21 (about 5.6∘ on a Gaussian grid) and10 non-equally-spacedσ vertical levels. Though relatively coarse,this resolution allows a realistic representation of large-scale atmosphericcirculation(Linsenmeier et al., 2015) and baroclinic structures that areimportant in the energy transport poleward in a slowly rotating andphase-locked planet like Earth(Lucarini et al., 2013). Allsimulations were integrated for 500 years at 40 min time steps, with modeloutput being stored as daily averages. Such long-term simulations allow themodel to approach an equilibrium state(Lucarini et al., 2017), whichwe characterized using the last 30 years of each simulation. The dynamicvegetation model SimBA was used to allow variations in terrestrialecosystems. The solar constant value was set to 1365 W m−2. ForatmosphericCO2, we used values of 360 and 415 ppm; however,differences among simulations were modest enough that here we present onlythe results obtained with the latter, which is closer to current estimates ofthis variable(Dlugokencky and Tans, 2019). All other model physicalconstants, planetary values and tuning parameters were kept at their defaultsetting(Fraedrich et al., 2005b).
The ocean was represented through prescribed SST values that were used todefine two scenarios: control (CTL) and permanent El Niño (PEN).Differences between scenarios are entirely determined by differences in theprescribed SST annual cycle (Figs. 1 and S1 in theSupplement). Due to the inherent chaotic nature of atmospheric dynamics, eachscenario was studied using an equally weighted ensemble of three simulationswith different initial conditions, which were varied through a random noisedisturbance in the surface pressure field(e.g. Kunz et al., 2009).Though we found little sensitivity to these variations in initial conditions(Fig. S2), we nevertheless used the ensemble means in our analyses. Averageresults from different scenarios were compared using a Student'st test ata significance level ofα=0.05 and 30 degrees of freedom(corresponding to a 30-year simulation period).
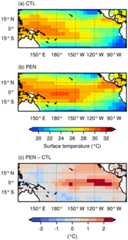
Figure 1Annual mean of prescribed SST climatology in the equatorial PacificOcean region for CTL (a) and PEN (b) simulations, and theirabsolute differences (c).
3.1 Control scenario and model evaluation
The CTL scenario is based on present-day climatology and therefore was firstused to assess the model's ability to realistically represent the Earth'sclimate system and then used as reference for comparison with the PENscenario. The CTL scenario is characterized by a climatological annual cycleof SST values obtained from the second phase of the Atmospheric ModelIntercomparison Project (AMIP II) for the period 1979–2010(Hurrell et al., 2008). Regridding to model resolution and adjusting theleast possible temperature to be 271.38 K was done following recommendationsbyTaylor et al. (2000).
Observational gridded data sets used to compare with the model results forthe CTL scenario are 1987–2016 monthly values of precipitation from theGlobal Precipitation Climatology Project(GPCP; Adler et al., 2003);1988–2017 monthly values of near-surface air temperature from theHadley Centre – Climatic Research Unit (HadCRUT4) temperature data set(Morice et al., 2012); 1979–2004 monthly values of mean sea levelpressure from the Hadley Centre Sea Level Pressure (HadSLP2) data set(Allan and Ansell, 2006); 2000–2015 monthly values of gross primaryproductivity (GPP) from the Moderate Resolution Imaging Spectroradiometer(MODIS) product MOD17A2(Zhao et al., 2005); and 1987–2016 monthlyvalues of free air temperature, as well as zonal and meridional winds atdifferent pressure levels (1000–50 hPa) from the European Centre forMedium-Range Weather Forecasts (ECMWF) Interim reanalysis(ERA-Interim; Dee et al., 2011). The global energy and water balancesproduced by PlaSim were compared with estimates based on ERA-Interim andthose reported byTrenberth et al. (2011). More details about alldata sets used in this work are shown in Tables S1 and S2 as part of theSupplement.
3.2 Permanent El Niño
In this scenario, the prescribed climatological annual cycle of SST wasreplaced with the annual cycle of El Niño that occurred between June 2015and May 2016, using monthly values from the AMIP II data set. This is thestrongest El Niño recorded so far, surpassing strong events from lastcentury: 1982–1983 and 1997–1998(Jiménez-Muñoz et al., 2016).Figure 1c shows the annual mean differences between the PENand CTL scenarios for the equatorial Pacific region. It can be seen that thisEl Niño event depicted well the first main mode of spatiotemporalvariability of SST anomalies (the mature phase of ENSO) and did not exhibitthe El Niño Modoki second main mode(Ashok et al., 2007). The PENclimatology has a global annual warm bias of+0.4 ∘C, with bothboreal winter (DJF) and autumn (SON) seasons having the largest positivedeviations (Fig. S1). The warm bias is greater specifically in the equatorialPacific Ocean, where it reaches an annual mean value of+0.8 ∘C.
Wara et al. (2005) suggest the possibility that permanentEl Niño-like conditions have occurred during the warm early Plioceneperiod. Using environmental reconstructions from isotopes and bioindicators,they found that the zonal west-to-east gradient of SST stayed very close tothe one observed during modern El Niño events. Although more recentstudies suggest that their reconstruction may underestimate the Pacific warmpool temperature and its variability(Zhang et al., 2014), it is stillinteresting to compare PEN scenario with data from the warm Pliocene period.Thus, we compared the SST forcing of PEN with the paleoenvironmentalreconstruction data set PRISM3(Dowsett et al., 2009), which covers saidperiod and has been used in several Pliocene modelling experiments(Haywood et al., 2016). PEN forcing is on average about 1 ∘Cwarmer in the tropics, though differences in this region are not allsignificant (Fig. S3). PEN is cooler than PRISM3 elsewhere. The west-to-eastzonal gradient was computed for CTL and PEN scenarios, as well as for PRISM3,at the sites shown in Fig. S3. For PEN, the gradient is 1.5 ∘C,whereas for PRISM3 it is 1.8 ∘C, and for CTL it is 3.1 ∘C.Since this gradient is a good indicator of strength of the Walker circulation(Wara et al., 2005), it is expected that circulation will be weaker inPEN than in the mid-Pliocene and much weaker than in the CTL experiment.
3.3 Climatological water deficit estimation
In this study, we focus on the consequences that such a PEN climate scenariocould have for terrestrial ecosystems. We used the maximum climatologicalwater deficit (MCWD) as defined byAragao et al. (2007), which is anindicator of accumulated water stress during dry seasons and has been linkedto ecosystem degradation via hydraulic failure in plants(Leitold et al., 2018), fires(Brando et al., 2014), and deforestation(Costa and Pires, 2010). We followed an approach similar toMalhi et al. (2009), who plotted MCWD versus mean annualprecipitation (MAP) to study the possibility of land cover transitions underclimate change projections in the Amazon rainforest.Malhi et al. (2009) found that most savannah biomes in Amazonia(i.e. Cerrado) had rainfall rates below 1500 mm year−1 and accumulatedwater deficits less than−300 mm. UnlikeMalhi et al. (2009), ouraim was global and therefore, in order to be able to calculate MCWD, we couldnot use a single fixed evapotranspiration rate (ET) value for the full extentof our domain. Instead, we used annual mean ET for every grid point from theCTL scenario as a proxy of expected ET with current land cover. Thesereference values (Fig. S4) agree with the approximation used byMalhi et al. (2009) of 100 mm per month average ET for Amazonia.Likewise, we assumed soil saturation at the wettest time of the year for eachgrid point and set the cumulative water deficit to zero for this month(Fig. S5).
Additionally, we judged useful to combine, in a single global map,statistically significant differences between scenarios in MAP and MCWD, inorder to support our discussion about the impact that a PEN scenario couldhave on terrestrial ecosystems. For this map, we defined 10 % of the CTLvalue as a threshold to identify places where differences between scenarioswere the largest. In this way, what we mean by “much greater/less” is thatthe difference exceeds the said threshold. Otherwise, even though both MAPand MCWD changed significantly for a particular grid point, this change wasnot as pronounced and only “greater/less” are used. Different coloursrepresent the possible combinations of changes in both variables.
4.1 Control climate
A comparison between PlaSim results for the CTL scenario and estimates fromERA-Interim andTrenberth et al. (2011, henceforth TFM11) showsthat simulations produce realistic estimates of the global energy and waterbudgets (Fig. 2). At the top of the atmosphere (TOA), themagnitude of the net energy imbalance (i.e. the difference Δ betweenincoming and outgoing radiation) is lower for PlaSim( W m−2) than it is for ERA-Interim( W m−2) and TFM11 ( W m−2). Previousstudies have reported that PlaSim's energy imbalance is closer to zero thanit is for many state-of-the-art climate models(Lucarini et al., 2010). Differences between PlaSim andobservations indicate that the model overestimates the outgoing solarradiation at TOA, as a result of more solar radiation being reflected both atthe surface and atmosphere, and less solar radiation being absorbed by theatmosphere. However, the model's estimate of solar radiation absorbed at thesurface falls in between those of ERA-Interim and TFM11. The surface energybalance in the model shows realistic estimates of thermal, latent heat (LH),and sensible heat (SH) fluxes, with a slightly overestimated Bowenratio (SH ∕ LH) of 0.23 as compared to 0.21 in both ERA-Interim andTFM11. These biases in the surface fluxes are likely to be related toprescribed values of aerodynamic roughness length(Yang et al., 2002), which has also been related to ERA-Interimbiases(Zhou and Wang, 2016). The model's representation of thegreenhouse effect through the estimate of thermal energy emitted by theatmosphere falls also within estimates from ERA-Interim and TFM11.
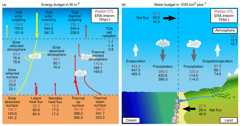
Figure 2Global average annual mean energy (a) andwater (b) budgets for the CTL simulation compared with ERA-InterimandTrenberth et al. (2011). Units are shown in the title of eachpanel.
As for the water budget, PlaSim presents a sensible distribution of wateramong ocean, land, and atmosphere, preserving key hydrological cyclecharacteristics: greater evaporation than precipitation over the oceans andgreater precipitation than evapotranspiration over continental areas(Fig. 2b). As compared to TFM11, atmospheric transport fromocean to land, as well as continental runoff, are underestimated both inPlaSim and ERA-Interim, but unlike the reanalysis, the water balance for themodel shows conservation. This is partly because the model values are notaffected by any assimilation scheme, as is the reanalysis(Seager and Henderson, 2013). Overall, PlaSim estimates of global waterfluxes fall within the uncertainty ranges proposed by TFM11 for ERA-Interimand other reanalyses.
There are statistically significant differences between PlaSim andobservational data in the global annual mean distribution of near-surface airtemperature (Fig. 3c), precipitation rate(Fig. 3f), GPP (Fig. 3i) and mean sea levelpressure (Fig. 3l). However, these biases are within typicalranges obtained for more comprehensive state-of-the-art climate models(Stevens et al., 2013) and cannot be entirely attributed to modeldeficiencies because of uncertainties in the observational data setsthemselves(e.g. Prein and Gobiet, 2017). Importantly, large-scale spatialpatterns of near-surface air temperature, precipitation rate, GPP, and meansea level pressure (Fig. 3), as well as their seasonality(Figs. S6–S9), are realistically represented by PlaSim.
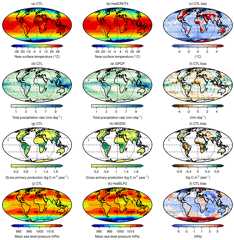
Figure 3Annual mean resultsfor the CTL simulation compared with observational data: near-surfacetemperature in CTL (a) and HadCRUT4 (b) and modelbias (c); total precipitation in CTL (d) andGPCP (e) and model bias (f); GPP in CTL (g) andMODIS (h) and model bias (i); and mean sea level pressurein CTL (j) and HadSLP2 (k) and model bias (l). Inthe bias panels, white depicts grid points with statistically non-significantdifference (α=0.05). Grid lines are spaced every 30∘ from theEquator and 90∘ from Greenwich, in latitude and longitude,respectively.
Differences between PlaSim results and HadCRUT4 (Fig. 3a–c)indicate that the model exhibits a global average cold bias of about−0.4 ∘C. This global bias combines an average warm bias of+0.9 ∘C over continental areas and an average cold bias of−1.4 ∘C over the oceans. The global average annual meantemperature in the CTL simulation (13.9 ∘C) is similar to that fromobservations (14.3 ∘C). Seasonal variations of temperature aresensibly simulated too (Fig. S6). Some of the largest temperature biasesoccur on land near polar regions, especially in Greenland and Antarctica, andregions with complex terrain such as the Himalayas mountain range. Biasesover complex terrain are related to known limitations even instate-of-the-art climate models, in part because the orography representationhas to be smoothed to keep dynamical stability(Flato et al., 2013).Warm biases in western Africa and Australia and slightly cold biases in theSahara desert and Amazonia are also observed. Strongest biases take place inboreal summer (JJA) and autumn (SON) seasons, when the global average biaseson land are+1.7 and+1.6 ∘C, respectively. Over the oceans,temperatures from the model are colder than observations year-round, with amaximum of−1.6 ∘C in boreal winter (DJF).
Annual mean daily precipitation from the CTL simulation is depicted inFig. 3d. The large-scale patterns exhibited in GPCP(Fig. 3e) are present: the equatorial maximum in theIntertropical Convergence Zone (ITCZ), middle latitude peaks ofsynoptic-scale weather systems, and low precipitation rates in the polarregions. There are high precipitation rates over the western equatorialPacific and the Western Hemisphere warm pools. The PlaSim global averageannual mean precipitation rate reaches 2.8 mm d−1, whereas forobservational data, it is 2.7 mm d−1. Concerning seasonal variation(Fig. S7), tropical precipitation shows latitudinal displacement and hasmaximum peaks in south-eastern Asia in the boreal winter (DJF) andsummer (JJA) seasons. In northern South America, the maximum of precipitationcrosses the Equator twice a year, unlike over the eastern Pacific where theITCZ stays north of the Equator for all seasons. PlaSim also displays thedouble ITCZ band that has been described for other global climate models(Lin, 2007), especially during the boreal spring season (MAM).
Figure 3g shows the dynamic vegetation results of CTL for GPP oncontinental areas. Global average annual mean for the CTL simulation reaches0.7 kg C m−2 year−1, whereas for MODIS data it is0.8 kg C m−2 year−1. Although large deviations with respect toMODIS can be seen in Fig. 3i, these are similar to thosereported in other modelling studies and could be related to the climate datasets used as input in the simulations(Ardö, 2015;Wu et al., 2018). Differences between this model and other data sets (whetherderived from observations or not) occur because parameterization schemes toobtain GPP indirectly from climate variables are different. Nevertheless, themodel shows a realistic estimate of the GPP distribution with highproductivity near the Equator and low productivity elsewhere. Also thelocation of the subtropical deserts is consistent with observations(Fig. 3h). Furthermore, the model is able to represent seasonalchanges in primary productivity (Fig. S8). There is a year-round highproductivity around the Equator, whereas for the Northern Hemisphere andSouthern Hemisphere it is at the maximum in their respective summer seasonand it is at the minimum in their respective winter season.
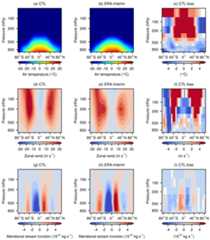
Figure 4Zonal average annual means results for the CTL simulation comparedwith ERA-Interim at different pressure levels for air temperature inCTL (a), ERA-Interim (b), and model bias (c);zonal wind in CTL (d), ERA-Interim (e), and modelbias (f); and meridional mass stream function in CTL (g),ERA-Interim (h), and model bias (i). In the bias panels,white depicts grid points with statistically non-significant difference(α=0.05).
When compared with observations, PlaSim also displays a very similar annualmean pattern of high- and low-pressure cells in the subtropics(Fig. 3j and k). The largest differences are seen nearAntarctica, where the model overestimates sea level pressure(Fig. 3l). Seasonally, the model is also able to represent theappearance of semi-permanent pressure systems throughout the year (Fig. S9).For instance, in the Northern Hemisphere, the Aleutian and Icelandic lows andthe Pacific, Azores, and Siberian highs are seen in winter, whereas only thePacific and Bermuda highs are seen in the summer season.
Regarding general circulation, the temperature gradient in the atmosphere forthe CTL simulation has a very similar structure as the one from ERA-Interimreanalysis (Fig. 4a and b). Differences are relatively smallbelow 500 hPa and away from the poles (Fig. 4c). Strongestbiases are found around 200 hPa. In the upper troposphere, the model is muchwarmer in the tropics and much cooler near the North Pole. This behaviour iscommon to most general circulation models, which have difficultiesparameterizing moist thermodynamic processes(Santer et al., 2017).Convective heating is overestimated in the Kuo-type convection schemeimplemented in PlaSim(Arakawa, 2004).
The distribution of temperature is closely related to the zonal wind velocity(Fig. 4d and e). The location of the subtropical jet streamsin the CTL simulation is in agreement with ERA-Interim, with the zonal windspeeds of the jet streams being higher in PlaSim. As with temperature, maindifferences are found in the upper troposphere and the lower stratosphereabove 200 hPa (Fig. 4f). This is a known problem with somegeneral circulation models that display too-fast zonal jet streams due to acoarse representation of the planet's orography and simple representation ofthe low-level drag(Pithan et al., 2016). The trade winds in PlaSimprevail around the Equator but are slower than in the reanalysis below300 hPa and faster above. As compared to ERA-Interim, zonal wind speed isunderestimated eastward in PlaSim at 60∘ S at all pressure levels.
PlaSim adequately simulates the thermally induced Hadley cell and itsdisplacement across the Equator for the annual mean (Fig. 4gand h), with clockwise rotation when in the Southern Hemisphere andanti-clockwise in the Northern Hemisphere. As described for observations,when in the Southern Hemisphere, the cell is stronger. Similarly, theindirect Ferrell cell can be seen moving in the opposite direction as theformer (different colour in Fig. 4g and h). Differences inthe stream function occur for the Hadley cell at both hemispheres, which isslower and shorter in PlaSim (Fig. 4i). Because of this, theindirect Ferrell cell is also smaller and is almost absent when in theNorthern Hemisphere. Polar cells are present but not visible in any of thesefigures because of the chosen contour levels.
Altogether, the PlaSim CTL simulation realistically represents thebroad-scale patterns seen in observational data of present-day climate. Inspite of PlaSim's simplicity and coarse resolution of simulations, the modelproduces a sensible picture of the current climatological state of theEarth's climate system. Our results generally agree with previous studiesshowing that PlaSim is able to simulate many dominant features of thepresent-day climate(e.g. Garreaud et al., 2010;Lucarini et al., 2017). Global averages ofannual mean and seasonal mean values for CTL and observational data sets arealso summarized in Table S3.
4.2 Permanent El Niño climate
To be able to judge this scenario on account of its different ENSO state withrespect to CTL, we checked that model bias was similar in PlaSim duringEl Niño conditions and CTL. Consequently, we compared the PEN scenario tothe observational data sets during very strong El Niño events, except forMODIS data which only include moderate ones (Fig. S10). Model bias for PENvariables is very similar to that shown in Fig. 3, and alsothere is good agreement in the broad-scale patterns seen in PEN andobservations during El Niño events. Figure S11 shows more clearly thatmodel bias is very similar in both experiments; hence, we can attribute mostof the differences between CTL and PEN to their ENSO condition.
Warmer-than-usual equatorial Pacific Ocean temperatures, as set in the PENscenario, lead to statistically significant changes in the global energy andwater budgets (Fig. 5). For the energy balance, a warmerocean surface results in higher long-wave radiation being emitted, as seen inthe heat fluxes and the thermal upward radiation. It also diminishes surfacesnow and sea ice formation, which is followed by a low planetary effectivealbedo, and therefore less solar incoming radiation is reflected. Like theocean, the atmosphere also becomes warmer and its thermal emission increases.Ultimately, this leads to less incoming solar radiation being reflected andmore long-wave radiation being emitted back to space. These two processes donot balance each other in the model, and therefore the net imbalance at TOAbecomes slightly more negative in PEN than in the CTL simulation(Fig. 5a). As expected, the PEN scenario shows much warmersurface and atmosphere than CTL.
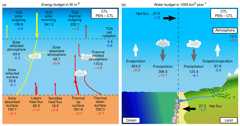
Figure 5Global average annual mean energy (a) andwater (b) budgets for the PEN simulation compared with the CTLsimulation. Units are shown in the title of each panel. En dashes (–) showstatistically non-significant differences(α=0.05).
According to PEN simulation outputs, water is redistributed throughout theworld (Fig. 5b). A warmer atmosphere stores more water, butthe net transport of water from the ocean to land is reduced. This may beexplained by changes in wind patterns over the Pacific Ocean, linked to theWalker circulation, which keep most water vapour above the ocean surface, asis typical during El Niño conditions(Diaz and Markgraf, 2000). Consistently,evaporation and precipitation increase over the ocean, while they decrease onland. The values in Fig. 5 are also presented in tabularform in Tables S4 and S5, including the results of the significance test.
There are statistically significant differences in the near-surface airtemperatures for the PEN scenario as compared to the CTL simulation(Fig. 6a). PEN has an average global annual mean increase ofapproximately 0.5 ∘C. This temperature change is greater on landwith an average 0.8 ∘C increase, than over the oceans, where thechange reaches 0.5 ∘C. In the tropics, variation occurs mainly inthe equatorial Pacific region (as expected) but also in Central America,northern South America, Amazonia, the Sahel region in Africa, and northernAustralia. Moreover, air over the North Atlantic Ocean is slightly colder,while the Arctic Ocean near Canada and Siberia is warmer. Air over some oceanareas in the Southern Hemisphere, close to Antarctica, becomes colder in PENthan in CTL. No significant differences in temperature were seen in most ofNorth America. Seasonally, boreal winter (DJF) shows the greatest warming onland, especially for Australia (Fig. S12). South America is the warmestduring boreal summer (JJA).
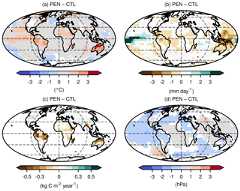
Figure 6Differences in annual means results for the PEN simulation comparedwith CTL simulation (PEN minus CTL) for near-surfacetemperature (a), daily precipitation (b), GPP (c),and mean sea level pressure (d). In all panels, white depicts gridpoints with statistically non-significant difference (α=0.05). Gridlines are spaced every 30∘ from the Equator and 90∘ fromGreenwich, in latitude and longitude, respectively.
Regarding precipitation, PEN leads to an average rise in global meanprecipitation of approximately 0.1 mm d−1 and a decrease incontinental areas of about the same value (Fig. 6b).Precipitation over the tropical Atlantic Ocean is also slightly reduced inthe PEN scenario. On land, the most conspicuous differences occur near theEquator, in Central America and in Amazonia, where precipitation is reduced.Conversely, in the Northern Hemisphere above midlatitudes, land areasexperience slightly higher precipitation rates. Similar to temperature,boreal winter (DJF) exhibits the largest deviations (Fig. S12), with above-(below)-CTL rainfall in equatorial eastern (western) Pacific Ocean.
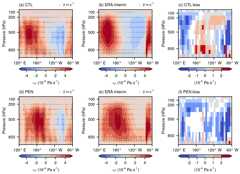
Figure 7Meridional divergent circulation in the equatorial Pacific for theCTL simulation (a), climatological ERA-Interim (b), and CTLbias in vertical velocity values (c), as well as for the PENsimulation (d), ERA-Interim mean of 1997–1998 and2015–2016 (e), and PEN bias in vertical velocityvalues (f). Vectors are plotted using the divergent meridional windcomponent and the negative vertical velocity in pressure units (−ω),averaged over latitudes 5∘ S to 5∘ N. Filled contours showthe magnitude of the vertical velocity. In all bias panels, white is forstatistically non-significant differences (α=0.05).
Higher temperatures and precipitation rates in temperate zones result inhigher productivity rates (Fig. 6c). The opposite happens inregions where temperatures increase, while precipitation rates decrease, asin Central America and northern South America and Amazonia. In Africa, nearthe Middle East, there is a relatively small increase in productivity relatedto increased precipitation. On the contrary, below the Sahel region andsouth-eastern Africa, GPP diminishes where precipitation declined withrespect to the CTL simulation. The same happens in Australia. Globally, adecrease in productivity of about 0.1 kg C m−2 year−1 occurs inthe PEN scenario. PEN results also show decreased productivity in SouthAmerica year-round (Fig. S13).
PEN simulation shows the Southern Oscillation hemispheric zonal dipole insurface pressure (Fig. 6d). In the tropics, there is anoverall increase in surface pressure over the oceans (except in the easternPacific), which is related to drier air and reduced transport of moistureinto continental areas. As expected, lower (higher) surface pressureconditions remain year-round in eastern (western) equatorial Pacific Ocean(Fig. S13). The North Atlantic region has lower surface pressure throughoutthe year but most markedly in boreal winter (DJF).
The atmosphere becomes warmer in PEN than it is in the CTL simulation at mostpressure levels and latitudes (Fig. S14a). There is only a slight cold biasin the Northern Hemisphere above 200 hPa. The hotspot in the tropics in theupper troposphere is much warmer in the PEN simulation. This result has beenpreviously reported and seems to be related to changes in the radiativeforcing of the troposphere layer(Lin et al., 2017). Wind speed increaseseastward (or lessens westward) in the model atmosphere (Fig. S14b). Notably,in the midlatitudes in the middle troposphere, the zonal velocity riseseastward, moving the subtropical jet streams towards the Equator as commonlyseen during El Niño conditions. As for the Hadley and Ferrell cells(Fig. S14c), their magnitude increases slightly in both hemispheres, as hasbeen previously stated(Oort and Yienger, 1996).
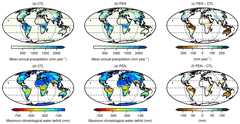
Figure 8Annual mean results for the PEN simulation compared with the CTLsimulation for MAP in CTL (a) and PEN (b) and theirabsolute difference (c), and MCWD in CTL (d) andPEN (e) and their absolute difference (f). In thedifference panels, white depicts grid points with statisticallynon-significant difference (α=0.05). Grid lines are spaced every30∘ from the Equator and 90∘ from Greenwich, in latitude andlongitude, respectively.
The Walker circulation in the equatorial Pacific is shown inFig. 7 for CTL and PEN simulations. These are compared toERA-Interim climatology and ERA-Interim during very strong El Niñoconditions, respectively. We observe very similar broad-scale structures insimulations and the reanalysis. In CTL, air rises in the west Pacific nearlongitude 150∘ E, flows eastward and sinks around longitude120∘ W. This is the expected behaviour during normal conditions(Collins et al., 2010) and is also displayed in ERA-Interim climatology.It seems like PlaSim underestimates slightly both upward and downward motionsas compared to the reanalysis. In the PEN simulation, convection is displacedtowards the central Pacific, while subsidence in the east is decreased. Thisis very similar to what happens in ERA-Interim for very strong El Niñoevents (mean of 1997–1998 and 2015–2016), although in the reanalysissubsidence in the eastern Pacific ceases almost completely. Overall, PlaSimis able to adequately show the weakening of the Walker circulation duringEl Niño-like conditions.
4.3 Climatological water deficit
Results for both MAP and MCWD are shown in Fig. 8. In terms ofMAP, the PEN simulation clearly shows rainfall decline in the tropics,whereas it increases in northern temperate latitudes. A similar situation isobserved for MCWD. As described in Sect. 3.3, we combinedthe differences in MAP and MCWD between scenarios in a single map that isshown in Fig. 9. This map resembles others that display theglobal effects of El Niño(e.g. Holmgren et al., 2001). According tothese results, tropical ecosystems seem to be the most vulnerable to a PENscenario, whereas northern temperate ecosystems could enhance their primaryproductivity due to greater water availability. We identify the followingregions as the most vulnerable: Central America, northern and central SouthAmerica, south-eastern Africa, and Australia. On the other hand, some partsof western North America and a wide band in Eurasia have greaterprecipitation, and cumulative water deficit has diminished.Figure 10 shows how conditions are different in CTL and PENscenarios, in terms of both MAP and MCWD, in each of these world regions. Inthis figure, for every grid point in a region of interest inFig. 9, markers are placed at their PEN value and a solid lineshows the distance to what they are in the CTL scenario. Most grid points inthe vulnerable regions (Fig. 10b, c, e, and f) shift towardsmuch less precipitation conditions with greater water deficits (see the shiftto the left). Using a similar approach asMalhi et al. (2009) forAmazonia, but in a global context near the tropics, these results indicatethat a PEN scenario can shift terrestrial ecosystems towards more aridconditions. In the case of ecosystems in North America and Eurasia(Fig. 10a and d), a PEN scenario drives them towards wetterconditions (see the shift to the right).
4.4 Warm Pliocene period
The possibility that sustained El Niño conditions might have occurredduring the Pliocene epoch compels us to compare the PEN experiment withprevious simulations of the warm Pliocene period.Haywood and Valdes (2004) simulated this period (using aCO2concentration of 400 ppm) and found global surface temperature to rise.Similarly, near-surface temperature increases in PEN scenario with respect toCTL. In both cases, global warming is greater on land than on the oceans.Regarding average precipitation rate, it increases slightly both in PEN andin the Pliocene simulation with respect to their control scenarios. Anotherinteresting correspondence is the fact that inHaywood and Valdes (2004)and in PEN, annual mean precipitation decreases in Central America and SouthAmerica (tropical regions), whereas it increases in the Northern Hemisphere.Particularly for Amazonia, both studies show that average temperature risesand precipitation decreases.Fedorov et al. (2013) report that in themid-Pliocene warm period there might have been strengthening of themeridional circulation, leading to aridification of Africa and Australia,which is similar to what we observe in PEN results. However, they also reportpossible aridification of North America, and this is the opposite of what isseen in PEN (Fig. 9). In spite of the similarities between PENand the mid-Pliocene warm period simulations, a biome reconstruction of theLate Pliocene period(Salzmann et al., 2011) seems to disagree with ourresults, specially for Africa and Australia, since it shows more vegetatedregions than we would expect with the water deficit changes observed in PEN.
Global terrestrial ecosystems can have alternative stable equilibrium sates(Scheffer et al., 2001) and can shift between them when externalperturbations and/or internal dynamics force them past critical thresholds(Barnosky et al., 2012;Andersen et al., 2009). Alternativeequilibria have already been identified for some biomes in the world. Intropical South America, in the eastern Amazon River basin, where there iscurrently a rainforest, simulation results suggest the presence of analternative savannah (Cerrado) state(Oyama and Nobre, 2003). In both the Saharaand Sahel regions in northern Africa, geological records and modellingresults suggest that environmental conditions could have supported a “greenSahara” and a “wet Sahel”, but climate shifts and atmosphere–vegetationfeedback mechanisms could have stabilized their current contrasting states(Brovkin et al., 1998;Foley et al., 2003). Similarly for the wet tropicsin Australia, bi-stability between rainforest and savannah-like sclerophyllland cover has also been studied(Warman and Moles, 2009). Forest,savannah, and desert seem to be three possible alternative stable states forterrestrial ecosystems(Hirota et al., 2011;Staver et al., 2011).
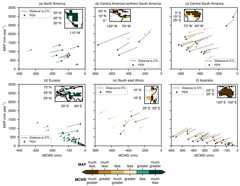
Figure 10Changes in MAP and MCWD in the PEN scenario compared to CTL fordifferent world regions. For every grid point shown in the zoomed map axes,we use markers (triangles) to show the value of both variables in the PENscenario, and a straight line shows the distance to the value they have inthe CTL scenario.
Malhi et al. (2009) studied the possibility of aclimate-change-induced state transition in the Amazon rainforest. Estimatingwater availability and accumulated water stress along a dry season, theyidentified transition areas between rainforest and savannah alternativestates for this large-scale ecosystem. Here, we extended this approach toother global ecosystems in order to evaluate whether a PEN scenario couldtrigger state transitions not only in Amazonia but also in other globalecosystems. The most noticeable effects suggested by the PlaSim model includea potential greening of western North America, dieback of the Amazonrainforest, drying of south-eastern Africa, and further aridification ofAustralia.
Increased precipitation in parts of North America is a known effect of strongEl Niño events(Ropelewski and Halpert, 1986) and can be seen in PENresults, as well as reductions in water deficit. In Fig. 10a, weobserve that significant changes in these variables (with respect to CTL)were greater in grid points around the Great Basin region, which is anendorheic watershed that includes arid and semi-arid biomes, and is locatedclose to the Mojave and Sonoran deserts. Several stable states for vegetationin said ecosystems include shrublands, short-grass steppes, and desertgrasslands(Laycock, 1991). Recently, it has been found thatEl Niño is linked to increased primary production in North America(Hu et al., 2019), as well as to growth and persistence of vegetation inthese desert areas(Huxman and Smith, 2001). Thus, in a PEN scenario,greater water availability could help avoid further aridification in thisregion and even trigger greening of some parts of it.
The opposite situation occurs in Central America and northern South Americaas seen in Fig. 10b. In this case, there is less annualprecipitation and the water deficit increases. Such results agree withobservations during El Niño events which show negative anomalies inseveral hydrological variables like rainfall and river flows for tropical South America and Central America(Poveda et al., 2006). In spite of many factors being at play,including the meridional displacement of the ITCZ, complex topography, andtwo different neighbouring oceans, ENSO has been found to be the greatestcause of interannual variability in this region(Poveda et al., 2006), andtherefore sustained El Niño conditions are very likely to have anoticeable impact as depicted in Fig. 10b. Since this region isconsidered a biodiversity hotspot in the world(Myers et al., 2000),ecosystem degradation could be related to a demise in its number ofbiological species due to the drastic changes in water availability observedin PEN. Moreover, hydropower generation is a common practice in this part ofthe world(Karmalkar et al., 2011;Poveda et al., 2003) and a change ofover 10 % in annual precipitation could hinder energy production.
Our results show that PEN can be a driver of forest dieback in the Amazon(Fig. 10c). El Niño reduces annual precipitation andincreases the water deficit across the dry season for this region(Cox et al., 2004;Malhi et al., 2009). This rainfall reduction couldperhaps threaten the existence of parts of the rainforest because resilienceto dry conditions is limited and a long-lasting drought can increase treemortality(Phillips et al., 2009). Most forest species operate within anarrow margin of hydraulic safety and therefore are vulnerable todrought-induced embolisms which reduce productivity and increase the risk offorest decline(Choat et al., 2012). Additionally, El Niño-inducedfires (not simulated by PlaSim) could further exacerbate forest degradationor transition from evergreen to seasonal tropical forests(Barlow and Peres, 2004;Brando et al., 2014). Consequences of this aremanifold, but one important aspect to consider is the global impact theAmazon rainforest has as a carbon sink(Cox et al., 2004;Pan et al., 2011).A PEN-induced dieback in the Amazon could tip the rainforest from a sink ofcarbon to a possible source(Davidson et al., 2012). This could in turnamplify the magnitude of extreme hydrological and weather events that occurduring El Niño, such as droughts, in a positive-feedback-like manner(Zemp et al., 2017;Salazar et al., 2018), as well as disrupt mechanisms ofriver flow regulation(Salazar et al., 2018;Mercado-Bettin et al., 2019) andcontinental precipitation distribution(Molina et al., 2019;Weng et al., 2018). Near-synchronous effects of thissort between different tipping elements could give rise to a “tippingcascade” that could contribute to a planetary-scale state shift(Lenton and Williams, 2013). Despite potential forest collapse in most of theAmazon, our results also agree withBetts et al. (2008) that some areasin western Amazonia (adjacent to the Andes) are less vulnerable and could actas a refuge for biodiversity. Consequences of a PEN scenario in the Amazonrainforest would have global impact, since it is the largest remaining intactforest in the world, which provide globally significant environmentalbenefits(Watson et al., 2018;Ellison et al., 2017).
In Eurasia (Fig. 10d), water availability rises in the PENscenario across central Europe, the Middle East around the Caspian Sea, andcentral Asia. This is a known feature of El Niño which increasesprecipitation rates in the region(Ineson and Scaife, 2009). Increased primaryproduction is another behaviour observed during El Niño years in Eurasia(Buermann et al., 2003) that is present in PEN. Particularly incentral Asia, a PEN scenario shows wetter conditions near Karakum andKyzylkum deserts, where it has been found recently that shrublands areextremely sensitive to climatic variations and that drought is the key driverof vegetation loss(Jiang et al., 2017). Therefore, we believe thatunder sustained El Niño conditions, desertification processes in centralAsia could be inhibited or slowed down. Compared to North America, changesare not as pronounced in precipitation and water deficit; hence, we believegreening mechanisms are less likely for Eurasia. The fact that both NorthAmerica and Eurasia exhibit similar results in the PEN scenario can berelated to warmer winter and spring seasons and a cooler summer season thatleads to greater primary productivity in both places, which is observed intemperate forests during El Niño years(Malhi et al., 1999).
The influence of ENSO on rainfall patterns in south-eastern Africa wasidentified long ago(Nicholson and Kim, 1997). This happens directlyvia atmospheric teleconnections(Ratnam et al., 2014) and also throughwarming of the Indian and Atlantic oceans(Giannini et al., 2008).El Niño in this region is linked to below average rainfall and droughts(Rouault and Richard, 2005). This is present in our results south of theGreat Horn of Africa, where the PEN scenario creates a greater water deficitand much less precipitation (Fig. 10e). Our results agree withGizaw and Gan (2017), who discussed the impact on Africa of increasedfrequency of El Niño episodes using climate projections andstate-of-the-art general circulation models. They also found south-easternAfrica to be particularly vulnerable. Earlier modelling studies found thatsuch savannah ecosystems in Africa are closely related to climatevariability, which keeps the system in between desert-like and forest-likeequilibrium states(Zeng and Neelin, 2000). More recent work also associates thesavannah equilibrium state to rainfall variability(Staver et al., 2011).Hence, tipping the ENSO permanently could trigger land cover transitions fromforest to savannah and/or savannah to desert-like biomes. This is problematicnot only in terms of ecosystem degradation but also in terms of putting atrisk food security and hydropower generation(Lyon, 2014). In thecase of west Africa, the impacts of ENSO have been contested because of themany other climatic drivers that come together in this area(Nicholson, 2013). However, ENSO events have been linked to climaticvariability in both the Sahel and Guinea coastal regions(Sheen et al., 2017;Joly and Voldoire, 2009;Tippett and Giannini, 2006). These tworegions are connected via the west African monsoon in boreal summer, whichbrings moisture inland from the Atlantic Ocean(Nicholson et al., 2017).During El Niño conditions, a coherent drying pattern for the Sahel hasbeen identified(Pomposi et al., 2016). PlaSim simulation resultsalso support the role of the warm ENSO phase in modulating rainfall in westAfrica and show it leads to a drier state in southern Sahel and Guinea coastregions (Fig. 8c). This southward expansion of the Sahel dryconditions into Guinea Coast, agrees with the notion of potential enlargementof the world's deserts due to climate change(Zeng and Yoon, 2009).Though this region only has a single grid point highlighted inFig. 9 (due to small changes in water deficit), we believe aPEN scenario could also push its arid and semi-arid ecosystems intovegetation–atmosphere positive feedback mechanisms of desertification.
El Niño events have had different effects on rainfall in Australia,depending on how far east the Walker circulation moves(Wang and Hendon, 2007). In this case, we show that a PEN based on thestrong El Niño of 2015–2016 is able to alter climate with potentiallysevere ecological consequences (Fig. 10f). Likewise, in spite ofobserved weakening of teleconnections between Australia rainfall and ENSO(Ashcroft et al., 2016), our results indicate that a PEN scenario couldcause significant widespread aridification of Australia. Coupling thisprocess with land cover changes could give rise to synergistic effects thatcould potentially tip Australia's climate towards a much drought-pronestate(McAlpine et al., 2009). Furthermore, extreme weather events(such as droughts) and changes in local hydrology have been identified as keydrivers of terrestrial and marine Australian ecosystems towards tippingpoints(Laurance et al., 2011). Hence, following our results, a PEN couldoriginate a “tipping cascade” in this continent.
Thus, the response of tropical and temperate ecosystems to PEN seems to beasymmetric: while tropical ecosystems are degraded (GPP is generallyreduced), temperate ecosystems are enhanced (GPP increases). This, combinedwith land cover changes (human or PEN induced), could turn tropical biomesinto global net sources of carbon that, according to current estimates(Pan et al., 2011), could not be counterbalanced by extratropical forests.
This study has provided additional evidence that PlaSim can realisticallyrepresent the current state of the Earth's climate system. Despite its coarseresolution and simplified parameterizations, the model is able to display thelarge-scale patterns of atmospheric dynamics seen in observational data sets.In describing some of its limitations, we found that it displays commonbiases seen in other state-of-the-art climate models. Therefore, PlaSimprovides an adequate framework to study the dynamics of the Earth system (asa whole), including complex climate feedback mechanisms, using longsimulations.
Our key finding is that a permanent tipping of the ENSO into a very strongwarm phase (El Niño) can trigger state transitions in global terrestrialecosystems, with asymmetric effects between the tropics and extratropicalregions. We identified some regions that seem to be more susceptible toshifting into more arid biomes: Central America, the Amazon rainforest,south-eastern Africa, and Australia, whereas parts of North America andEurasia could become more productive. These results also suggest thepossibility that a permanent El Niño can trigger tipping cascades. It isimportant to mention that despite there being other sources of variabilityfor these ecosystems (for instance, the Atlantic Ocean temperaturesvariation), here, we focused only on the consequences that an observed verystrong El Niño-based SST climatology could have on continental areas,without linking any simulated anomalies to a specific region in space. Thismeans that despite the Pacific Ocean showing the largest anomalies (as inEl Niño), we cannot attribute all of our results to changes only in thisregion. Even though a modelling study of this sort is not enough to provethat such state shifts will occur in a climate change scenario, it does showa consistent picture and should raise concern about the conservation ofglobal ecosystems and related water resources management practices in a worldwith more frequent and/or intense El Niño events(Latif et al., 2015;Cai et al., 2014).
All observational data sets and reanalyses used in thisstudy are publicly available online. Model results are readily available uponrequest.
The supplement related to this article is available online at: https://doi.org/10.5194/esd-10-631-2019-supplement.
MDV performed the simulations. All authors participatedin the design of the experiments, the discussion of the results, and the writing of the paper.
The authors declare that they have no conflict of interest.
We thank Daniel Ruiz Carrascal and Luis F. Salazar for insightful comments on an early version of the manuscript. We also thank Stephen Sitch and Lina Mercado for their valuable remarks. Finally, we thank Rui A. P. Perdigão and two anonymous reviewers for their helpful observations.
This research has been supported by the Universidad deAntioquia (Estudiante Instructor program) and the UT-GRA (Programa deinvestigación en la gestión de riesgo asociado con cambioclimático y ambiental en cuencas hidrográficas) (grant no. 543-2011 Colciencias).
This paper was edited by Rui A. P. Perdigão and reviewed by two anonymous referees.
Adler, R. F., Huffman, G. J., Chang, A., Ferraro, R., Xie, P.-P., Janowiak, J., Rudolf, B., Schneider, U., Curtis, S., Bolvin, D., Gruber, A., Susskind, J., Arkin, P., and Nelkin, E.: The Version-2 Global Precipitation Climatology Project (GPCP) Monthly Precipitation Analysis (1979–Present), J. Hydrometeorol., 4, 1147–1167,https://doi.org/10.1175/1525-7541(2003)004<1147:TVGPCP>2.0.CO;2, 2003. a
Alencar, A., Nepstad, D., and Diaz, M. C. V.: Forest understory fire in theBrazilian Amazon in ENSO and non-ENSO years: area burned and committed carbon emissions, Earth Interact., 10, 1–17,https://doi.org/10.1175/EI150.1, 2006. a
Allan, R. and Ansell, T.: A New Globally Complete Monthly Historical GriddedMean Sea Level Pressure Dataset (HadSLP2): 1850–2004, J. Climate, 19, 5816–5842,https://doi.org/10.1175/JCLI3937.1, 2006. a
Andersen, T., Carstensen, J., Hernandez-Garcia, E., and Duarte, C. M.:Ecological Thresholds And Regime Shifts: Approaches To Identification, TrendsEcol. Evol., 24, 49–57,https://doi.org/10.1016/j.tree.2008.07.014, 2009. a
Aragao, L. E. O., Malhi, Y., Roman-Cuesta, R. M., Saatchi, S., Anderson, L. O., and Shimabukuro, Y. E.: Spatial Patterns And Fire Response Of RecentAmazonian Droughts, Geophys. Res. Lett., 34, L07701,https://doi.org/10.1029/2006GL028946, 2007. a
Arakawa, A.: The cumulus parameterization problem: past, present, and future,J. Climate, 17, 2493–2525, 2004. a
Ardö, J.: Comparison between remote sensing and a dynamic vegetation model for estimating terrestrial primary production of Africa, Carbon Balance Manage., 10, 1–15, 2015. a
Ashcroft, L., Gergis, J., and Karoly, D. J.: Long-term Stationarity Of El Niño–Southern Oscillation Teleconnections In Southeastern Australia, Clim. Dynam., 46, 2991–3006,https://doi.org/10.1007/s00382-015-2746-3, 2016. a
Ashok, K., Behera, S. K., Rao, S. A., Weng, H., and Yamagata, T.: El NiñoModoki and its possible teleconnection, J. Geophys. Res.-Oceans, 112, 1–27, 2007. a
Barlow, J. and Peres, C. A.: Ecological Responses To El Niño-InducedSurface Fires In Central Brazilian Amazonia: Management Implications ForFlammable Tropical Forests, Philos. T. Roy. Soc. B, 359, 367–380,https://doi.org/10.1098/rstb.2003.1423, 2004. a
Barnosky, A. D., Hadly, E. A., Bascompte, J., Berlow, E. L., Brown, J. H.,Fortelius, M., Getz, W. M., Harte, J., Hastings, A., Marquet, P. A., Martinez, N. D., Mooers, A., Roopnarine, P., Vermeij, G., Williams, J. W., Gillespie, R., Kitzes, J., Marshall, C., Matzke, N., Mindell, D. P., Revilla, E., and Smith, A. B.: Approaching A State Shift In Earth's Biosphere, Nature, 486, 52–58,https://doi.org/10.1038/nature11018, 2012. a,b
Bathiany, S., Claussen, M., and Fraedrich, K.: Implications Of ClimateVariability For The Detection Of Multiple Equilibria And For Rapid Transitions In The Atmosphere-Vegetation System, Clim. Dynam., 38, 1775–1790,https://doi.org/10.1007/s00382-011-1037-x, 2012. a,b
Betts, R. A., Malhi, Y., and Roberts, J. T.: The Future Of The Amazon: NewPerspectives From Climate, Ecosystem And Social Sciences, Philos. T. Roy. Soc. B, 363, 1729–1735,https://doi.org/10.1098/rstb.2008.0011, 2008. a
Boschi, R., Lucarini, V., and Pascale, S.: Bistability Of The Climate AroundThe Habitable Zone: A Thermodynamic Investigation, Icarus, 226, 1724–1742,https://doi.org/10.1016/j.icarus.2013.03.017, 2013. a,b
Brando, P. M., Balch, J. K., Nepstad, D. C., Morton, D. C., Putz, F. E., Coe,M. T., Silvério, D., Macedo, M. N., Davidson, E. A., Nóbrega, C. C.,Alencar, A., and Soares-Filho, B. S.: Abrupt Increases In Amazonian Tree Mortality Due To Drought-Fire Interactions, P. Natl. Acad. Sci. USA, 111, 6347–6352,https://doi.org/10.1073/pnas.1305499111, 2014. a,b
Brovkin, V., Claussen, M., Petoukhov, V., and Ganopolski, A.: On The Stability Of The Atmosphere-Vegetation System In The Sahara/Sahel Region, J.Geophys. Res.-Atmos., 103, 31613–31624,https://doi.org/10.1029/1998JD200006, 1998. a
Buermann, W., Anderson, B., Tucker, C. J., Dickinson, R. E., Lucht, W., Potter, C. S., and Myneni, R. B.: Interannual covariability in Northern Hemisphere air temperatures and greenness associated with El Niño–Southern Oscillation and the Arctic Oscillation, J. Geophys. Res.-Atmos., 108, 4396,https://doi.org/10.1029/2002JD002630, 2003. a
Cai, W., Borlace, S., Lengaigne, M., Van Rensch, P., Collins, M., Vecchi, G.,Timmermann, A., Santoso, A., McPhaden, M. J., Wu, L., England, M. H., Wang, G., Guilyardi, E., and Jin, F.-F.: Increasing Frequency Of Extreme El Niño Events Due To Greenhouse Warming, Nat. Clim. Change, 4, 111–116,https://doi.org/10.1038/nclimate2100, 2014. a,b,c
Cai, W., Santoso, A., Wang, G., Yeh, S.-W., An, S.-I., Cobb, K. M., Collins,M., Guilyardi, E., Jin, F.-F., Kug, J.-S., Lengaigne, M., McPhaden, M. J., Takahashi, K., Timmermann, A., Vecchi, G., Watanabe, M., and Wu, L.: ENSO And Greenhouse Warming, Nat. Clim. Change, 5, 849–859,https://doi.org/10.1038/nclimate2743, 2015. a,b,c
Choat, B., Jansen, S., Brodribb, T. J., Cochard, H., Delzon, S., Bhaskar, R.,Bucci, S. J., Feild, T. S., Gleason, S. M., Hacke, U. G., Jacobsen, A. L., Lens, F., Maherali, H., Martínez-Vilalta, J., Mayr, S., Mencuccini, M., Mitchell, P. J., Nardini, A., Pittermann, J., Pratt, R. B., Sperry, J. S., Westoby, M., Wright, I. J., and Zanne, A. E.: Global Convergence In The Vulnerability Of Forests To Drought, Nature, 491, 752–755,https://doi.org/10.1038/nature11688, 2012. a
Claussen, M., Mysak, L., Weaver, A., Crucifix, M., Fichefet, T., Loutre, M.-F., Weber, S., Alcamo, J., Alexeev, V., Berger, A., Calov, R., Ganopolski, A., Goosse, H., Lohmann, G., Lunkeit, F., Mokhov, I., Petoukhov, V., Stone, P., and Wang, Z.: Earth System Models Of Intermediate Complexity: Closing The Gap In The Spectrum Of Climate SystemModels, Clim. Dynam., 18, 579–586,https://doi.org/10.1007/s00382-001-0200-1, 2002. a
Collins, M., An, S.-I., Cai, W., Ganachaud, A., Guilyardi, E., Jin, F.-F.,Jochum, M., Lengaigne, M., Power, S., Timmermann, A., Vecchi, G., and Wittenberg, A.: The impact of global warming on the tropical Pacific Ocean and El Niño, Nat. Geosci., 3, 391–397,https://doi.org/10.1038/ngeo868, 2010. a
Costa, M. H. and Pires, G. F.: Effects Of Amazon And Central BrazilDeforestation Scenarios On The Duration Of The Dry Season In The Arc OfDeforestation, Int. J. Climatol., 30, 1970–1979,https://doi.org/10.1002/joc.2048, 2010. a
Cox, P. M., Betts, R., Collins, M., Harris, P. P., Huntingford, C., and Jones, C.: Amazonian Forest Dieback Under Climate-Carbon Cycle Projections For The 21st Century, Theor. Appl. Climatol., 78, 137–156,https://doi.org/10.1007/s00704-004-0049-4, 2004. a,b
Davidson, E. A., de Araújo, A. C., Artaxo, P., Balch, J. K., Brown, I. F., Bustamante, M. M., Coe, M. T., DeFries, R. S., Keller, M., Longo, M., Munger, J. W., Schroeder, W., Soares-Filho, B. S., Souza, C. M., and Wofsy, S. C.: The Amazon Basin In Transition, Nature, 481, 321–328,https://doi.org/10.1038/nature10717, 2012. a
Dee, D. P., Uppala, S. M., Simmons, A. J., Berrisford, P., Poli, P., Kobayashi, S., Andrae, U., Balmaseda, M. A., Balsamo, G., Bauer, P., Bechtold, P., Beljaars, A. C. M., van de Berg, L., Bidlot, J., Bormann, N., Delsol, C., Dragani, R., Fuentes, M., Geer, A. J., Haimberger, L., Healy, S. B., Hersbach, H., Hólm, E. V., Isaksen, L., Kållberg, P., Köhler, M., Matricardi, M., McNally, A. P., Monge-Sanz, B. M., Morcrette, J.-J., Park, B.-K., Peubey, C., de Rosnay, P., Tavolato, C., Thépaut, J.-N., andVitart, F.: The ERA-Interim Reanalysis: Configuration And Performance Of The Data Assimilation System, Q. J. Roy. Meteorol. Soc., 137, 553–597,https://doi.org/10.1002/qj.828, 2011. a
Dekker, S., De Boer, H., Brovkin, V., Fraedrich, K., Wassen, M., and Rietkerk, M.: Biogeophysical Feedbacks Trigger Shifts In The ModelledVegetation–Atmosphere System At Multiple Scales, Biogeosciences, 7, 1237–1245,https://doi.org/10.5194/bg-7-1237-2010, 2010. a,b
Diaz, H. F. and Markgraf, V.: El Niño and the Southern Oscillation:Multiscale variability and global and regional impacts, Cambridge UniversityPress, Cambridge, 2000. a,b
Dlugokencky, E. and Tans, P.: Globally averaged marine surface monthly meandata, available at:https://www.esrl.noaa.gov/gmd/ccgg/trends/, last access: 8 August 2019. a
Dowsett, H., Robinson, M., and Foley, K.: Pliocene three-dimensional globalocean temperature reconstruction, Clim. Past, 5, 769–783,https://doi.org/10.5194/cp-5-769-2009, 2009. a
Ellison, D., Morris, C. E., Locatelli, B., Sheil, D., Cohen, J., Murdiyarso,D., Gutierrez, V., Van Noordwijk, M., Creed, I. F., Pokorny, J., Gaveau, D., Spracklen, D. V., Bargués Tobella, A., Ilstedt, U., Teuling, A. J., Gebrehiwot, S. G., Sands, D. C., Muys, B., Verbist, B., Springgay, E., Sugandi, Y., and Sullivan, C. A.: Trees, forests and water: Cool insights for a hot world, Global Environ. Change, 43, 51–61,https://doi.org/10.1016/j.gloenvcha.2017.01.002, 2017. a
Fedorov, A., Brierley, C., Lawrence, K. T., Liu, Z., Dekens, P., and Ravelo,A.: Patterns and mechanisms of early Pliocene warmth, Nature, 496, 43–49,https://doi.org/10.1038/nature12003, 2013. a
Flato, G., Marotzke, J., Abiodun, B., Braconnot, P., Chou, S. C., Collins, W. J., Cox, P., Driouech, F., Emori, S., Eyring, V., Forest, C., Gleckler, P., Guilyardi, E., Jakob, C., Kattsov, V., Reason, C., and Rummukaine, M.: Evaluation Of Climate Models, in: Climate Change 2013: the Physical Science Basis, Contribution of Working Group I to the Fifth Assessment Report of the Intergovernmental Panel on Climate Change, edited by: Stocker, T. F., Qin, D., Plattner, G.-K., Tignor, M., Allen, S. K., Boschung, J., Nauels, A., Xia, Y., Bex, V., and Midgley, P. M., Cambridge University Press, Cambridge, 741–866,https://doi.org/10.1017/CBO9781107415324.020, 2013. a
Foley, J. A., Botta, A., Coe, M. T., and Costa, M. H.: El Niño–SouthernOscillation and the climate, ecosystems and rivers of Amazonia, Global Biogeochem. Cy., 16, 1132,https://doi.org/10.1029/2002GB001872, 2002. a
Foley, J. A., Coe, M. T., Scheffer, M., and Wang, G.: Regime Shifts In TheSahara And Sahel: Interactions Between Ecological And Climatic Systems InNorthern Africa, Ecosystems, 6, 524–532,https://doi.org/10.1007/s10021-002-0227-0, 2003. a
Fraedrich, K.: A Suite Of User-Friendly Global Climate Models: HysteresisExperiments, Eur. Phys. J. Plus, 127, 1–9,https://doi.org/10.1140/epjp/i2012-12053-7, 2012. a
Fraedrich, K., Jansen, H., Kirk, E., Luksch, U., and Lunkeit, F.: The PlanetSimulator: Towards A User Friendly Model, Meteorol. Z., 14, 299–304,https://doi.org/10.1127/0941-2948/2005/0043, 2005a. a,b
Fraedrich, K., Jansen, H., Kirk, E., and Lunkeit, F.: The Planet Simulator:Green Planet And Desert World, Meteorol. Z., 14, 305–314,https://doi.org/10.1127/0941-2948/2005/0044, 2005b. a,b,c
Fraedrich, K., Kirk, E., Luksch, U., and Lunkeit, F.: The Portable UniversityModel Of The Atmosphere (PUMA): Storm Track Dynamics And Low-FrequencyVariability, Meteorol. Z., 14, 735–745,https://doi.org/10.1127/0941-2948/2005/0074, 2005c. a
Garreaud, R. D., Molina, A., and Farias, M.: Andean Uplift, Ocean Cooling AndAtacama Hyperaridity: A Climate Modeling Perspective, Earth Planet. Sc. Lett., 292, 39–50,https://doi.org/10.1016/j.epsl.2010.01.017, 2010. a,b
Giannini, A., Biasutti, M., Held, I. M., and Sobel, A. H.: A Global Perspective On African Climate, Climatic Change, 90, 359–383,https://doi.org/10.1007/s10584-008-9396-y, 2008. a
Gizaw, M. S. and Gan, T. Y.: Impact Of Climate Change And El NiñoEpisodes On Droughts In Sub-Saharan Africa, Clim. Dynam., 49, 665–682,https://doi.org/10.1007/s00382-016-3366-2, 2017. a
Guilyardi, E.: El Niño-mean state-seasonal cycle interactions in amulti-model ensemble, Clim. Dynam., 26, 329–348, 2006. a
Haywood, A. M. and Valdes, P. J.: Modelling Pliocene warmth: contribution ofatmosphere, oceans and cryosphere, Earth Planet. Sc. Lett., 218, 363–377,https://doi.org/10.1016/S0012-821X(03)00685-X, 2004. a,b
Haywood, A. M., Dowsett, H. J., and Dolan, A. M.: Integrating geologicalarchives and climate models for the mid-Pliocene warm period, Nat. Commun., 7, 10646,https://doi.org/10.1038/ncomms10646, 2016. a
Henrot, A.-J., François, L., Brewer, S., and Munhoven, G.: Impacts OfLand Surface Properties And AtmosphericCO2 On The Last GlacialMaximum Climate: A Factor Separation Analysis, Clim. Past, 5, 183–202,https://doi.org/10.5194/cp-5-183-2009, 2009. a
Hirota, M., Holmgren, M., Van Nes, E. H., and Scheffer, M.: Global resilienceof tropical forest and savanna to critical transitions, Science, 334,232–235,https://doi.org/10.1126/science.1210657, 2011. a
Holmgren, M., Scheffer, M., Ezcurra, E., Gutiérrez, J. R., and Mohren, G. M.: El Niño Effects On The Dynamics Of Terrestrial Ecosystems, TrendsEcol. Evol., 16, 89–94,https://doi.org/10.1016/S0169-5347(00)02052-8, 2001. a,b
Holmgren, M., Stapp, P., Dickman, C. R., Gracia, C., Graham, S., Gutiérrez, J. R., Hice, C., Jaksic, F., Kelt, D. A., Letnic, M., Lima, M., López, B., Meserve, P. L., Milstead, W. B., Polis, G. A., Previtali, M. A., Richter, M., Sabaté, S., and Squeo, F. A.: A synthesis of ENSO effects on drylands in Australia, North America and South America, Adv. Geosci., 6, 69–72,https://doi.org/10.5194/adgeo-6-69-2006, 2006. a
Hoskins, B. and Simmons, A.: A multi-layer spectral model and the semi-implicit method, Q. J. Roy. Meteorol. Soc., 101, 637–655,https://doi.org/10.1002/qj.49710142918, 1975. a
Hu, L., Andrews, A. E., Thoning, K. W., Sweeney, C., Miller, J. B., Michalak,A. M., Dlugokencky, E., Tans, P. P., Shiga, Y. P., Mountain, M., Nehrkorn, T., Montzka, S. A., McKain, K., Kofler, J., Trudeau, M., Michel, S. E., Biraud, S. C., Fischer, M. L., Worthy, D. E. J., Vaughn, B. H., White, J. W. C., Yadav, V., Basu, S., and van der Velde, I. R.: Enhanced North American carbon uptake associated with El Niño, Sci. Adv., 5, eaaw0076,https://doi.org/10.1126/sciadv.aaw0076, 2019. a
Hurrell, J. W., Hack, J. J., Shea, D., Caron, J. M., and Rosinski, J.: A NewSea Surface Temperature And Sea Ice Boundary Dataset For The CommunityAtmosphere Model, J. Climate, 21, 5145–5153,https://doi.org/10.1175/2008JCLI2292.1, 2008. a
Huxman, T. E. and Smith, S. D.: Photosynthesis in an invasive grass and native forb at elevatedCO2 during an El Niño year in the Mojave Desert, Oecologia, 128, 193–201,https://doi.org/10.1007/s004420100658, 2001. a
Indeje, M., Semazzi, F. H., and Ogallo, L. J.: ENSO signals in East African rainfall seasons, Int. J. Climatol., 20, 19–46,https://doi.org/10.1002/(SICI)1097-0088(200001)20:1<19::AID-JOC449>3.0.CO;2-0, 2000. a
Ineson, S. and Scaife, A.: The role of the stratosphere in the European climate response to El Niño, Nat. Geoscience, 2, 32–36,https://doi.org/10.1038/ngeo381, 2009. a
Jiang, L., Jiapaer, G., Bao, A., Guo, H., and Ndayisaba, F.: Vegetation dynamics and responses to climate change and human activities in Central Asia, Sci. Total Environ., 599, 967–980,https://doi.org/10.1016/j.scitotenv.2017.05.012, 2017. a
Jiménez-Muñoz, J. C., Mattar, C., Barichivich, J.,Santamaría-Artigas, A., Takahashi, K., Malhi, Y., Sobrino, J. A., andvan der Schrier, G.: Record-Breaking Warming And Extreme Drought In TheAmazon Rainforest During The Course Of El Niño 2015–2016, Scient. Rep., 6, 33130,https://doi.org/10.1038/srep33130, 2016. a
Joly, M. and Voldoire, A.: Influence Of ENSO On The West African Monsoon:Temporal Aspects And Atmospheric Processes, J. Climate, 22, 3193–3210,https://doi.org/10.1175/2008JCLI2450.1, 2009. a
Karmalkar, A. V., Bradley, R. S., and Diaz, H. F.: Climate change in CentralAmerica and Mexico: regional climate model validation and climate changeprojections, Clim. Dynam., 37, 605–629,https://doi.org/10.1007/s00382-011-1099-9, 2011. a
Kleidon, A.: The Climate Sensitivity To Human Appropriation Of VegetationProductivity And Its Thermodynamic Characterization, Global Planet. Change, 54, 109–127,https://doi.org/10.1016/j.gloplacha.2006.01.016, 2006. a
Kleidon, A.: Non-Equilibrium Thermodynamics, Maximum Entropy Production AndEarth-System Evolution, Philos. T. Roy. Soc. Lond. A, 368, 181–196,https://doi.org/10.1098/rsta.2009.0188, 2010. a
Kunz, T., Fraedrich, K., and Lunkeit, F.: Response Of Idealized Baroclinic Wave Life Cycles To Stratospheric Flow Conditions, J. Atmos. Sci., 66, 2288–2302,https://doi.org/10.1175/2009JAS2827.1, 2009. a
Latif, M., Semenov, V. A., and Park, W.: Super El Niños In Response ToGlobal Warming In A Climate Model, Climatic Change, 132, 489–500,https://doi.org/10.1007/s10584-015-1439-6, 2015. a,b
Laurance, W. F., Dell, B., Turton, S. M., Lawes, M. J., Hutley, L. B.,McCallum, H., Dale, P., Bird, M., Hardy, G., Prideaux, G., Gawne, B., McMahon, C. R., Yu, R., Hero, J.-M., Schwarzkopf, L., Krockenberger, A., Douglas, M., Silvester, E., Mahony, M., Vella, K., Saikia, U., Wahren, C.-H., Xu, Z., Smith, B., and Cocklin, C.: The 10 Australian Ecosystems Most Vulnerable To Tipping Points, Biol. Conserv., 144, 1472–1480,https://doi.org/10.1016/j.biocon.2011.01.016, 2011. a
Laycock, W. A.: Stable states and thresholds of range condition on NorthAmerican rangelands: a viewpoint, Rangeland Ecol. Manage./J. Range Manage. Arch., 44, 427–433, 1991. a
Leitold, V., Morton, D. C., Longo, M., dos Santos, M. N., Keller, M., andScaranello, M.: El Niño Drought Increased Canopy Turnover In Amazon Forests, New Phytol., 219, 959–971,https://doi.org/10.1111/nph.15110, 2018. a
Lenton, T. M.: Early Warning Of Climate Tipping Points, Nat. Clim. Change,1, 201–209,https://doi.org/10.1038/nclimate1143, 2011. a
Lenton, T. M. and Ciscar, J. C.: Integrating Tipping Points Into Climate Impact Assessments, Climatic Change, 117, 585–597,https://doi.org/10.1007/s10584-012-0572-8, 2013. a
Lenton, T. M. and Williams, H. T.: On The Origin Of Planetary-Scale TippingPoints, Trends Ecol. Evol., 28, 380–382,https://doi.org/10.1016/j.tree.2013.06.001, 2013. a
Lenton, T. M., Held, H., Kriegler, E., Hall, J. W., Lucht, W., Rahmstorf, S.,and Schellnhuber, H. J.: Tipping Elements In The Earth's Climate System, P.Natl. Acad. Sci. USA, 105, 1786–1793,https://doi.org/10.1073/pnas.0705414105, 2008. a,b,c,d
Lin, J.-L.: The double-ITCZ problem in IPCC AR4 coupled GCMs:Ocean-atmosphere feedback analysis, J. Climate, 20, 4497–4525, 2007. a
Lin, P., Paynter, D., Ming, Y., and Ramaswamy, V.: Changes of the tropicaltropopause layer under global warming, J. Climate, 30, 1245–1258, 2017. a
Linsenmeier, M., Pascale, S., and Lucarini, V.: Climate Of Earth-Like Planets With High Obliquity And Eccentric Orbits: Implications For HabitabilityConditions, Planet. Space Sci., 105, 43–59,https://doi.org/10.1016/j.pss.2014.11.003, 2015. a,b,c
Lucarini, V., Fraedrich, K., and Lunkeit, F.: Thermodynamic Analysis OfSnowball Earth Hysteresis Experiment: Efficiency, Entropy Production AndIrreversibility, Q. J. Roy. Meteorol. Soc., 136, 2–11,https://doi.org/10.1002/qj.543, 2010. a
Lucarini, V., Pascale, S., Boschi, R., Kirk, E., and Iro, N.: Habitability And Multistability In Earth-Like Planets, Astron. Nachr., 334, 576–588,https://doi.org/10.1002/asna.201311903, 2013. a,b
Lucarini, V., Ragone, F., and Lunkeit, F.: Predicting Climate Change UsingResponse Theory: Global Averages And Spatial Patterns, J. Stat. Phys., 166, 1036–1064,https://doi.org/10.1007/s10955-016-1506-z, 2017. a,b
Lunkeit, F., Borth, H., Böttinger, M., Fraedrich, K., Jansen, H., Kirk, E., Kleidon, A., Luksch, U., Paiewonsky, P., Schubert, S., Sielmann, S., and Wan, H.: Planet Simulator Reference Manual Version 16, MeteorologischesInstitut, Universität Hamburg, Hamburg, 2011. a,b
Lyon, B.: Seasonal Drought In The Greater Horn Of Africa And Its RecentIncrease During The March–May Long Rains, J. Climate, 27, 7953–7975,https://doi.org/10.1175/JCLI-D-13-00459.1, 2014. a
Malhi, Y., Baldocchi, D., and Jarvis, P.: The Carbon Balance Of Tropical,Temperate And Boreal Forests, Plant Cell Environ., 22, 715–740,https://doi.org/10.1046/j.1365-3040.1999.00453.x, 1999. a
Malhi, Y., Aragão, L. E., Galbraith, D., Huntingford, C., Fisher, R., Zelazowski, P., Sitch, S., McSweeney, C., and Meir, P.: Exploring The Likelihood And Mechanism Of A Climate-Change-Induced Dieback Of The Amazon Rainforest, P. Natl. Acad. Sci. USA, 106, 20610–20615,https://doi.org/10.1073/pnas.0804619106, 2009. a,b,c,d,e,f,g
McAlpine, C., Syktus, J., Ryan, J., Deo, R., McKeon, G., McGowan, H., andPhinn, S.: A Continent Under Stress: Interactions, Feedbacks And RisksAssociated With Impact Of Modified Land Cover On Australia's Climate, Global Change Biol., 15, 2206–2223,https://doi.org/10.1111/j.1365-2486.2009.01939.x, 2009. a
McPhaden, M. J., Zebiak, S. E., and Glantz, M. H.: ENSO As An IntegratingConcept In Earth Science, Science, 314, 1740–1745,https://doi.org/10.1126/science.1132588, 2006. a,b
Mercado-Bettin, D., Salazar, J., and Villegas, J.: Long-term water balancepartitioning explained by physical and ecological characteristics in worldriver basins, Ecohydrology, 12, e2072,https://doi.org/10.1002/eco.2072, 2019. a
Molina, R. D., Salazar, J. F., Martínez, J. A., Villegas, J. C., andArias, P. A.: Forest-induced exponential growth of precipitation alongclimatological wind streamlines over the Amazon, J. Geophys. Res.-Atmos., 124, 2589–2599,https://doi.org/10.1029/2018JD029534, 2019. a
Morice, C. P., Kennedy, J. J., Rayner, N. A., and Jones, P. D.: QuantifyingUncertainties In Global And Regional Temperature Change Using An Ensemble OfObservational Estimates: The HadCRUT4 Data Set, J. Geophys. Res.-Atmos., 117, 1–22,https://doi.org/10.1029/2011jd017187, 2012. a
Myers, N., Mittermeier, R. A., Mittermeier, C. G., Da Fonseca, G. A., and Kent, J.: Biodiversity hotspots for conservation priorities, Nature, 403, 853–858,https://doi.org/10.1038/35002501, 2000. a
Nicholson, S. E.: The West African Sahel: A Review Of Recent Studies On The Rainfall Regime And Its Interannual Variability, ISRN Meteorol., 2013, 453521,https://doi.org/10.1155/2013/453521, 2013. a
Nicholson, S. E. and Kim, J.: The Relationship Of The El Niño–SouthernOscillation To African Rainfall, Int. J. Climatol., 17, 117–135,https://doi.org/10.1002/(SICI)1097-0088(199702)17:2<117::AID-JOC84>3.0.CO;2-O, 1997. a
Nicholson, S. E., Funk, C., and Fink, A. H.: Rainfall Over The African Continent From The 19th Through The 21st Century, Global Planet. Change, 165, 114–127,https://doi.org/10.1016/j.gloplacha.2017.12.014, 2017. a
Oort, A. H. and Yienger, J. J.: Observed Interannual Variability In The Hadley Circulation And Its Connection To ENSO, J. Climate, 9, 2751–2767,https://doi.org/10.1175/1520-0442(1996)009<2751:OIVITH>2.0.CO;2, 1996. a
Orszag, S. A.: Transform Method For The Calculation Of Vector-Coupled Sums:Application To The Spectral Form Of The Vorticity Equation, J. Atmos. Sci., 27, 890–895,https://doi.org/10.1175/1520-0469(1970)027<0890:TMFTCO>2.0.CO;2, 1970. a
Oyama, M. D. and Nobre, C. A.: A New Climate-Vegetation Equilibrium State forTropical South America, Geophys. Res. Lett., 30, 2199,https://doi.org/10.1029/2003gl018600, 2003. a
Pan, Y., Birdsey, R. A., Fang, J., Houghton, R., Kauppi, P. E., Kurz, W. A.,Phillips, O. L., Shvidenko, A., Lewis, S. L., Canadell, J. G., Ciais, P., Jackson, R. B., Pacala, S. W., McGuire, A. D., Piao, S., Rautiainen, A., Sitch, S., and Hayes, D.: A Large And Persistent Carbon Sink In The World's Forests, Science, 333, 988–993,https://doi.org/10.1126/science.1201609, 2011. a,b
Phillips, O. L., Aragão, L. E., Lewis, S. L., Fisher, J. B., Lloyd, J.,López-González, G., Malhi, Y., Monteagudo, A., Peacock, J., Quesada,C. A., van der Heijden, G., Almeida, S., Amaral, I., Arroyo, L., Aymard, G., Baker, T. R., Bánki, O., Blanc, L., Bonal, D., Brando, P., Chave, J., Alves de Oliveira, Á. C., Cardozo, N. D., Czimczik, C. I., Feldpausch, T. R., Freitas, M. A., Gloor, E., Higuchi, N., Jiménez, E., Lloyd, G., Meir, P., Mendoza, C., Morel, A., Neill, D. A., Nepstad, D., Patiño, S., Peñuela, M. C., Prieto, A., Ramírez, F., Schwarz, M., Silva, J., Silveira, M., Thomas, A. S., ter Steege, H., Stropp, J., Vásquez, R., Zelazowski, P., Alvarez Dávila, E., Andelman, S., Andrade, A., Chao, K.-J., Erwin, T., Di Fiore, A., Honorio, E. C., Keeling, H., Killeen, T. J., Laurance, W. F., Peña Cruz, A., Pitman, N. C. A., Núñez Vargas, P., Ramírez-Angulo, H., Rudas, A., Salamão, R., Silva, N., Terborgh, J., and Torres-Lezama, A.: Drought Sensitivity Of The Amazon Rainforest, Science, 323, 1344–1347,https://doi.org/10.1126/science.1164033, 2009. a
Pithan, F., Shepherd, T. G., Zappa, G., and Sandu, I.: Climate Model Biases In Jet Streams, Blocking And Storm Tracks Resulting From Missing OrographicDrag, Geophys. Res. Lett., 43, 7231–7240,https://doi.org/10.1002/2016gl069551, 2016. a
Pomposi, C., Giannini, A., Kushnir, Y., and Lee, D. E.: Understanding PacificOcean Influence On Interannual Precipitation Variability In The Sahel, Geophys. Res. Lett., 43, 9234–9242,https://doi.org/10.1002/2016GL069980, 2016. a
Poveda, G., Mesa, O. J., and Waylen, P. R.: Nonlinear forecasting of riverflows in Colombia based upon ENSO and its associated economic value forhydropower generation, in: Climate and water, Springer, 351–371,https://doi.org/10.1007/978-94-015-1250-3_15, 2003. a
Poveda, G., Waylen, P. R., and Pulwarty, R. S.: Annual and inter-annualvariability of the present climate in northern South America and southernMesoamerica, Palaeogeogr. Palaeocl. Palaeoecol., 234, 3–27,https://doi.org/10.1016/j.palaeo.2005.10.031, 2006. a,b
Prein, A. F. and Gobiet, A.: Impacts Of Uncertainties In European GriddedPrecipitation Observations On Regional Climate Analysis, Int. J. Climatol., 37, 305–327,https://doi.org/10.1002/joc.4706, 2017. a
Ratnam, J., Behera, S., Masumoto, Y., and Yamagata, T.: Remote Effects Of El Niño And Modoki Events On The Austral Summer Precipitation Of SouthernAfrica, J. Climate, 27, 3802–3815,https://doi.org/10.1175/JCLI-D-13-00431.1, 2014. a
Ropelewski, C. F. and Halpert, M. S.: North American precipitation andtemperature patterns associated with the El Niño/Southern Oscillation (ENSO), Mon. Weather Rev., 114, 2352–2362,https://doi.org/10.1175/1520-0493(1986)114<2352:NAPATP>2.0.CO;2, 1986. a
Rouault, M. and Richard, Y.: Intensity And Spatial Extent Of Droughts InSouthern Africa, Geophys. Res. Lett., 32, L15702,https://doi.org/10.1029/2005GL022436, 2005. a
Salazar, J. F., Villegas, J. C., Rendón, A. M., Rodríguez, E., Hoyos,I., Mercado-Bettín, D., and Poveda, G.: Scaling properties revealregulation of river flows in the Amazon through a forest reservoir, Hydrol.Earth Syst. Sci., 22, 1735–1748,https://doi.org/10.5194/hess-22-1735-2018, 2018. a,b
Salzmann, U., Williams, M., Haywood, A. M., Johnson, A. L., Kender, S., andZalasiewicz, J.: Climate and environment of a Pliocene warm world, Palaeogeogr. Palaeocl. Palaeoecol., 309, 1–8,https://doi.org/10.1016/j.palaeo.2011.05.044, 2011. a
Santer, B. D., Solomon, S., Pallotta, G., Mears, C., Po-Chedley, S., Fu, Q.,Wentz, F., Zou, C.-Z., Painter, J., Cvijanovic, I., and Bonfils, C.: Comparing tropospheric warming in climate models and satellite data, J. Climate, 30, 373–392, 2017. a
Sausen, R., Schubert, S., and Dümenil, L.: A model of river runoff for use in coupled atmosphere-ocean models, J. Hydrol., 155, 337–352,https://doi.org/10.1016/0022-1694(94)90177-5, 1994. a
Scheffer, M., Carpenter, S., Foley, J. A., Folke, C., and Walker, B.:Catastrophic Shifts In Ecosystems, Nature, 413, 591–596,https://doi.org/10.1038/35098000, 2001. a
Scheffer, M., Bascompte, J., Brock, W. A., Brovkin, V., Carpenter, S. R.,Dakos, V., Held, H., Van Nes, E. H., Rietkerk, M., and Sugihara, G.:Early-Warning Signals For Critical Transitions, Nature, 461, 53–59,https://doi.org/10.1038/nature08227, 2009. a
Schmittner, A., Silva, T. A., Fraedrich, K., Kirk, E., and Lunkeit, F.: Effects Of Mountains And Ice Sheets On Global Ocean Circulation, J. Climate, 24, 2814–2829,https://doi.org/10.1175/2010JCLI3982.1, 2011. a
Seager, R. and Henderson, N.: Diagnostic computation of moisture budgets in the ERA-Interim reanalysis with reference to analysis of CMIP-archivedatmospheric model data, J. Climate, 26, 7876–7901,https://doi.org/10.1175/JCLI-D-13-00018.1, 2013. a
Semtner Jr., A. J.: A model for the thermodynamic growth of sea ice in numerical investigations of climate, J. Phys. Oceanog., 6, 379–389,https://doi.org/10.1175/1520-0485(1976)006<0379:AMFTTG>2.0.CO;2, 1976. a
Sheen, K., Smith, D., Dunstone, N., Eade, R., Rowell, D., and Vellinga, M.:Skilful Prediction Of Sahel Summer Rainfall On Inter-Annual And Multi-YearTimescales, Nat. Commun., 8, 14966,https://doi.org/10.1038/ncomms14966, 2017. a
Spiegl, T., Paeth, H., and Frimmel, H.: Evaluating Key Parameters For TheInitiation Of A Neoproterozoic Snowball Earth With A Single Earth System Model Of Intermediate Complexity, Earth Planet. Sc. Lett., 415, 100–110,https://doi.org/10.1016/j.epsl.2015.01.035, 2015. a
Staver, A. C., Archibald, S., and Levin, S. A.: The Global Extent AndDeterminants Of Savanna And Forest As Alternative Biome States, Science, 334,230–232,https://doi.org/10.1126/science.1210465, 2011. a,b
Stevens, B., Giorgetta, M., Esch, M., Mauritsen, T., Crueger, T., Rast, S.,Salzmann, M., Schmidt, H., Bader, J., Block, K., Brokopf, R., Fast, I.,Kinne, S., Kornblueh, L., Lohmann, U., Pincus, R., Reichler, T., and Roeckner, E.: Atmospheric Component Of The MPI-M Earth System Model: ECHAM6, J. Adv. Model. Earth Syst., 5, 146–172,https://doi.org/10.1002/jame.20015, 2013. a
Taylor, K. E., Williamson, D., and Zwiers, F.: The sea surface temperature and sea-ice concentration boundary conditions for AMIP II simulations, Tech. Rep. 60, Program for Climate Model Diagnosis and Intercomparison, Lawrence Livermore National Laboratory, University of California, California, 2000. a
Timmermann, A., Oberhuber, J., Bacher, A., Esch, M., Latif, M., and Roeckner,E.: Increased El Niño Frequency In A Climate Model Forced By FutureGreenhouse Warming, Nature, 398, 694–697,https://doi.org/10.1038/19505, 1999. a
Tippett, M. K. and Giannini, A.: Potentially Predictable Components OfAfrican Summer Rainfall In An SST-forced GCM Simulation, J. Climate, 19, 3133–3144,https://doi.org/10.1175/JCLI3779.1, 2006. a
Trenberth, K. E., Fasullo, J. T., and Mackaro, J.: Atmospheric MoistureTransports From Ocean To Land And Global Energy Flows In Reanalyses, J. Climate, 24, 4907–4924,https://doi.org/10.1175/2011jcli4171.1, 2011. a,b,c
Wang, G. and Hendon, H. H.: Sensitivity Of Australian Rainfall To Inter-El Niño Variations, J. Climate, 20, 4211–4226,https://doi.org/10.1175/JCLI4228.1, 2007. a
Wara, M. W., Ravelo, A. C., and Delaney, M. L.: Permanent El Niño-likeconditions during the Pliocene warm period, Science, 309, 758–761,https://doi.org/10.1126/science.1112596, 2005. a,b,c
Warman, L. and Moles, A. T.: Alternative stable states in Australia's WetTropics: a theoretical framework for the field data and a field-case for the theory, Landsc. Ecol., 24, 1–13,https://doi.org/10.1007/s10980-008-9285-9, 2009. a
Watson, J. E., Evans, T., Venter, O., Williams, B., Tulloch, A., Stewart, C.,Thompson, I., Ray, J. C., Murray, K., Salazar, A., McAlpine, C., Potapov, P., Walston, J., Robinson, J. G., Painter, M., Wilkie, D., Filardi, C., Laurance, W. F., Houghton, R. A., Maxwell, S., Grantham, H., Samper, C., Wang, S., Laestadius, L., Runting, R. K., Silva-Chávez, G. A., Ervin, J., and Lindenmayer, D.: The Exceptional Value Of Intact Forest Ecosystems, Nat. Ecol. Evol., 2, 599–610,https://doi.org/10.1038/s41559-018-0490-x, 2018. a
Weng, W., Luedeke, M. K., Zemp, D. C., Lakes, T., and Kropp, J. P.: Aerial and surface rivers: downwind impacts on water availability from land use changes in Amazonia, Hydrol. Earth Syst. Sci., 22, 911–927,https://doi.org/10.5194/hess-22-911-2018, 2018. a
Wu, Z., Boke-Olén, N., Fensholt, R., Ardö, J., Eklundh, L., andLehsten, V.: Effect of climate dataset selection on simulations of terrestrial GPP: Highest uncertainty for tropical regions, PloS One, 13,e0199383,https://doi.org/10.1371/journal.pone.0199383, 2018. a
Yang, K., Koike, T., Fujii, H., Tamagawa, K., and Hirose, N.: Improvement ofSurface Flux Parametrizations With A Turbulence-Related Length, Q. J. Roy. Meteorol. Soc., 128, 2073–2087,https://doi.org/10.1256/003590002320603548, 2002. a
Yeh, S.-W., Cai, W., Min, S.-K., McPhaden, M. J., Dommenget, D., Dewitte, B.,Collins, M., Ashok, K., An, S.-I., Yim, B.-Y., and Kug, J.-S.: ENSO Atmospheric Teleconnections And Their Response To Greenhouse Gas Forcing, Rev. Geophys., 56, 185–206,https://doi.org/10.1002/2017RG000568, 2018. a
Zebiak, S. E., Orlove, B., Muñoz, Á. G., Vaughan, C., Hansen, J., Troy, T., Thomson, M. C., Lustig, A., and Garvin, S.: Investigating El Niño–Southern Oscillation And Society Relationships, WileyInterdisciplin. Rev.: Clim. Change, 6, 17–34,https://doi.org/10.7916/D84X577H, 2015. a
Zemp, D. C., Schleussner, C.-F., Barbosa, H. M., Hirota, M., Montade, V.,Sampaio, G., Staal, A., Wang-Erlandsson, L., and Rammig, A.: Self-amplifiedAmazon Forest Loss Due To Vegetation-Atmosphere Feedbacks, Nat. Commun., 8, 14681,https://doi.org/10.1038/ncomms14681, 2017. a
Zeng, N. and Neelin, J. D.: The Role Of Vegetation-Climate Interaction AndInterannual Variability In Shaping The African Savanna, J. Climate, 13, 2665–2670,https://doi.org/10.1175/1520-0442(2000)013<2665:TROVCI>2.0.CO;2, 2000. a
Zeng, N. and Yoon, J.: Expansion Of The World's Deserts Due ToVegetation-Albedo Feedback Under Global Warming, Geophys. Res. Lett., 36, L17401,https://doi.org/10.1029/2009GL039699, 2009. a
Zhang, Y. G., Pagani, M., and Liu, Z.: A 12-million-year temperature history of the tropical Pacific Ocean, Science, 344, 84–87,https://doi.org/10.1126/science.1246172, 2014. a
Zhao, M., Heinsch, F. A., Nemani, R. R., and Running, S. W.: ImprovementS OfThe MODIS Terrestrial Gross And Net Primary Production Global Data Set,Remote Sens. Environ., 95, 164–176,https://doi.org/10.1016/j.rse.2004.12.011, 2005. a
Zhou, C. and Wang, K.: Evaluation Of Surface Fluxes In ERA-Interim UsingFlux Tower Data, J. Climate, 29, 1573–1582,https://doi.org/10.1175/JCLI-D-15-0523.1, 2016. a