
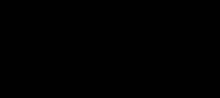
(III.I.1-1) we can use a two step procedure to solve the problem of heteroskedasticity as follows:  | divide each observation by the S.D. of the error term for that observation | |  | apply LS to the transformed observations | |
This procedure is called theWeighted Least Squares (WLS). Of course there exist a lot of alternative transformations. One of the most popular transformations is the Neperian logarithm, since it gives more weight to small-valued observations and less weight to large ones. The transformation of time series according to the logarithmic and related transformations is (in econometrics) mostly assumed to be theory-related. Another method is specially designed to solve the problem ofmultiplicative heteroskedasticity. Suppose 
(III.I.1-2) 
From (III.I.1-2) we find 
(III.I.1-3) 

(III.I.1-4) The only question remaining is "How to estimate alpha?". We may rewrite (III.I.1-2) by taking logarithms 
(III.I.1-5) and since 
(III.I.1-6) it is obvious that 
(III.I.1-7) Now we put all t elements from (III.I.1-7) in matrices and obtain estimates of alpha for the model 
(III.I.1-8) by solving 
(III.I.1-9) Once the alpha parameter vector has been computed this information can be used in the followingEstimated Generalized Least Squares estimator (EGLS) 
(III.I.1-10) Consider the following multiple regression equation (to be used in subsequent illustrations):
Detection of heteroskedasticity can be achieved by many different tests. If we assume a linear statistical model of the form 
(III.I.1-11) then a test for heteroskedasticity, according toGlejser, can be obtained by testing 
in one of the following models 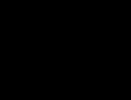
(III.I.1-12) (and many others...). Warning: this test should only be used if the endogenous variable is NOT used as lagged exogenous variable. Furthermore the Gleisjer tests assume ADDITIVE heteroskedasticity. All OLS assumptions should be satisfied. Below you �ll find an example of how Gleisjer tests can be applied to test for heteroskedasticity (this test is applied to our example-equation):
Another popular test is the so-calledlikelihood ratio test for heteroskedasticity 
(III.I.1-13) 

(III.I.1-14) which can be used for testing statistical significance. TheGoldfeld-Quandt test for heteroskedasticity uses test-equations for each exogenous variable (except the constant term). This test is widely applicable, and fairly unproblematic w.r.t. it�s properties. The Goldfeld-Quandt test uses two regressions of the endogenous variable on each variable separately; the first regression is based on LOW values of the exogenous variable, the second regression is based on HIGH values of the exogenous variable. Note that a pre-specified number of values in-between LOW and HIGH values are NOT used in these regressions. Below you �ll find an example of how the Goldfeld-Quandt test can be applied to test for heteroskedasticity (this test is applied to our example-equation):
ThePark tests for heteroskedasticity uses a test-equation for each exogenous variable: the logarithms of squared residuals are explained by the logarithm of the absolute values of the exogenous variable. Warning: this test should only be used if the endogenous variable is NOT used as lagged exogenous variable. Furthermore the Park tests assume MULTIPLICATIVE heteroskedasticity. All OLS assumptions should be satisfied. Below you will find an example of how the Park test can be applied to test for heteroskedasticity (this test is applied to our example-equation):
TheBreusch-Pagan test for heteroskedasticity uses a test-equation: the squared residuals divided by the residual variance are explained by all exogenous variables. The test statistic is computed as half the difference between the Total Sum of Squares and the Sum of Squared Residuals, which has a Chi-square distribution. Warning: this test should only be used if the endogenous variable is NOT used as lagged exogenous variable AND if the number of observations is VERY LARGE. All OLS assumptions should be satisfied, including normality of the error term. Below you �ll find an example of how the Breusch-Pagan test can be applied to test for heteroskedasticity (this test is applied to our example-equation):
TheSquared Residuals versus Squared Fit test for heteroskedasticity uses a test-equation: the squared residuals are explained by the squared interpolation forecast of the original regression. This test is fairly unproblematic and can be used in almost all cases. The t-statistic of the Squared-Fit-parameter indicates whether heteroskedasticity is present or not. Below you �ll find an example of how the Squared Residuals versus Squared Fittest can be applied to test for heteroskedasticity (this test is applied to our example-equation):
TheARCH(p) test is used to test for Autoregressive Conditional Heteroskedasticity: the squared residuals are explained by it�s lagged values (p is the number of lags included in the test-equation). The presence of Conditional Heteroskedasticity is tested by the use of an F-statistic. Below you �ll find an example of how the ARCH(p)test can be applied to test for Conditional Heteroskedasticity (this test is applied to our example-equation):
|