A Fast Algorithm for Estimating Two-Dimensional Sample Entropy Based on an Upper Confidence Bound and Monte Carlo Sampling
- PMID:38392410
- PMCID: PMC10887568
- DOI: 10.3390/e26020155
A Fast Algorithm for Estimating Two-Dimensional Sample Entropy Based on an Upper Confidence Bound and Monte Carlo Sampling
Abstract
The two-dimensional sample entropy marks a significant advance in evaluating the regularity and predictability of images in the information domain. Unlike the direct computation of sample entropy, which incurs a time complexity of O(N2) for the series withN length, the Monte Carlo-based algorithm for computing one-dimensional sample entropy (MCSampEn) markedly reduces computational costs by minimizing the dependence onN. This paper extends MCSampEn to two dimensions, referred to as MCSampEn2D. This new approach substantially accelerates the estimation of two-dimensional sample entropy, outperforming the direct method by more than a thousand fold. Despite these advancements, MCSampEn2D encounters challenges with significant errors and slow convergence rates. To counter these issues, we have incorporated an upper confidence bound (UCB) strategy in MCSampEn2D. This strategy involves assigning varied upper confidence bounds in each Monte Carlo experiment iteration to enhance the algorithm's speed and accuracy. Our evaluation of this enhanced approach, dubbed UCBMCSampEn2D, involved the use of medical and natural image data sets. The experiments demonstrate that UCBMCSampEn2D achieves a 40% reduction in computational time compared to MCSampEn2D. Furthermore, the errors with UCBMCSampEn2D are only 30% of those observed in MCSampEn2D, highlighting its improved accuracy and efficiency.
Keywords: Monte Carlo algorithm; sample entropy; upper confidence bound strategy.
Conflict of interest statement
The authors declare no conflicts of interest.
Figures
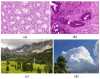
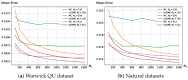
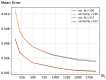
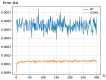
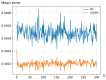
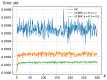
Similar articles
- A Super Fast Algorithm for Estimating Sample Entropy.Liu W, Jiang Y, Xu Y.Liu W, et al.Entropy (Basel). 2022 Apr 8;24(4):524. doi: 10.3390/e24040524.Entropy (Basel). 2022.PMID:35455187Free PMC article.
- Lower confidence bounds for prediction accuracy in high dimensions via AROHIL Monte Carlo.Dobbin KK, Cooke S.Dobbin KK, et al.Bioinformatics. 2011 Nov 15;27(22):3129-34. doi: 10.1093/bioinformatics/btr542. Epub 2011 Sep 28.Bioinformatics. 2011.PMID:21965820
- Fast computation of sample entropy and approximate entropy in biomedicine.Pan YH, Wang YH, Liang SF, Lee KT.Pan YH, et al.Comput Methods Programs Biomed. 2011 Dec;104(3):382-96. doi: 10.1016/j.cmpb.2010.12.003. Epub 2011 Jan 5.Comput Methods Programs Biomed. 2011.PMID:21208680
- A polar-coordinate-based pencil beam algorithm for VMAT dose computation with high-resolution gantry angle sampling.Tseng W, Yan G, Liu H, Kahler D, Li J, Liu C, Lu B.Tseng W, et al.Med Phys. 2022 Jun;49(6):4026-4042. doi: 10.1002/mp.15638. Epub 2022 Apr 12.Med Phys. 2022.PMID:35355285
- Metropolis sampling in pedigree analysis.Sobel E, Lange K.Sobel E, et al.Stat Methods Med Res. 1993;2(3):263-82. doi: 10.1177/096228029300200305.Stat Methods Med Res. 1993.PMID:8261261Review.
References
Grants and funding
LinkOut - more resources
Full Text Sources