Grey Model Optimized by Particle Swarm Optimization for Data Analysis and Application of Multi-Sensors
- PMID:30071641
- PMCID: PMC6111666
- DOI: 10.3390/s18082503
Grey Model Optimized by Particle Swarm Optimization for Data Analysis and Application of Multi-Sensors
Abstract
Data on the effective operation of new pumping station is scarce, and the unit structure is complex, as the temperature changes of different parts of the unit are coupled with multiple factors. The multivariable grey system prediction model can effectively predict the multiple parameter change of a nonlinear system model by using a small amount of data, but the value of itsq parameters greatly influences the prediction accuracy of the model. Therefore, the particle swarm optimization algorithm is used to optimize theq parameters and the multi-sensor temperature data of a pumping station unit is processed. Then, the change trends of the temperature data are analyzed and predicted. Comparing the results with the unoptimized multi-variable grey model and the BP neural network prediction method trained under insufficient data conditions, it is proved that the relative error of the multi-variable grey model after optimizing theq parameters is smaller.
Keywords: multi-sensor temperature data; multivariable grey system prediction; pumping station; q parameters; temperature change.
Conflict of interest statement
The authors declare no conflict of interest.
Figures
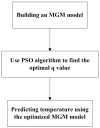
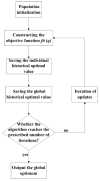
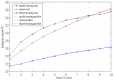
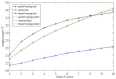
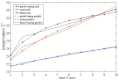
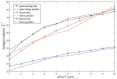
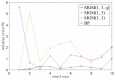
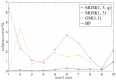
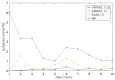
Similar articles
- An optimized Nash nonlinear grey Bernoulli model based on particle swarm optimization and its application in prediction for the incidence of Hepatitis B in Xinjiang, China.Zhang L, Zheng Y, Wang K, Zhang X, Zheng Y.Zhang L, et al.Comput Biol Med. 2014 Jun;49:67-73. doi: 10.1016/j.compbiomed.2014.02.008. Epub 2014 Feb 20.Comput Biol Med. 2014.PMID:24747730
- Short-term prediction of COVID-19 spread using grey rolling model optimized by particle swarm optimization.Ceylan Z.Ceylan Z.Appl Soft Comput. 2021 Sep;109:107592. doi: 10.1016/j.asoc.2021.107592. Epub 2021 Jun 9.Appl Soft Comput. 2021.PMID:34121965Free PMC article.
- Study on an Assembly Prediction Method of RV Reducer Based on IGWO Algorithm and SVR Model.Jin S, Cao M, Qian Q, Zhang G, Wang Y.Jin S, et al.Sensors (Basel). 2022 Dec 29;23(1):366. doi: 10.3390/s23010366.Sensors (Basel). 2022.PMID:36616963Free PMC article.
- Prediction and Optimization of Electrospun Polyacrylonitrile Fiber Diameter Based on Grey System Theory.Zhou Q, Lin L, Chen G, Du Z.Zhou Q, et al.Materials (Basel). 2019 Jul 11;12(14):2237. doi: 10.3390/ma12142237.Materials (Basel). 2019.PMID:31336710Free PMC article.
- The Prediction of the Gas Utilization Ratio based on TS Fuzzy Neural Network and Particle Swarm Optimization.Zhang S, Jiang H, Yin Y, Xiao W, Zhao B.Zhang S, et al.Sensors (Basel). 2018 Feb 20;18(2):625. doi: 10.3390/s18020625.Sensors (Basel). 2018.PMID:29461469Free PMC article.
Cited by
- A Comparative Study on the Prediction of Occupational Diseases in China with Hybrid Algorithm Combing Models.Lu Y, Yan H, Zhang L, Liu J.Lu Y, et al.Comput Math Methods Med. 2019 Sep 29;2019:8159506. doi: 10.1155/2019/8159506. eCollection 2019.Comput Math Methods Med. 2019.PMID:31662788Free PMC article.
- Research on an Equivalent Heat Source Model of the AC Arc in the Short Gap of a Copper-Core Cable and a Fire Risk Assessment Method.Li Y, Zhang R, Yang K, Qi Y.Li Y, et al.Sensors (Basel). 2024 Feb 23;24(5):1443. doi: 10.3390/s24051443.Sensors (Basel). 2024.PMID:38474981Free PMC article.
References
- Sinay J., Pacaiova H., Oravec M. Present state of machinery safety assessment; Proceedings of the 16th International DAAAM Symposium: Intelligent Manufacturing and Automation: Focus on Young Researchers and Scientists; Opatija, Croatia. 19–21 October 2005; pp. 347–348.
- Carle P.F., Alford T., Bibelhausen D. Machinery Condition Assessment Module. 7,593,784. U.S. Patent No. 2009 Sep 22
- Liu X.Y., Qiu D. Analyzing and Treating the Failure of Unit 1 Governor Screw Pump of Wuqiangxi Hydropower Station. North China Electr. Power. 2010;4:016.
- Qiu B., Wang T., Wei Q.L., Tang Z.J., Gong W.M. Common fault analysis of large pumping stations. J. Drain. Irrig. Eng. 1999;2:20–24.
- Bi Z., Li C., Li X., Gao H. Research on Fault Diagnosis for Pumping Station Based on T-S Fuzzy Fault Tree and Bayesian Network. J. Electr. Comput. Eng. 2017;2017:1–11. doi: 10.1155/2017/6175429. - DOI
LinkOut - more resources
Full Text Sources
Other Literature Sources