Long short-term memory RNN for biomedical named entity recognition
- PMID:29084508
- PMCID: PMC5663060
- DOI: 10.1186/s12859-017-1868-5
Long short-term memory RNN for biomedical named entity recognition
Abstract
Background: Biomedical named entity recognition(BNER) is a crucial initial step of information extraction in biomedical domain. The task is typically modeled as a sequence labeling problem. Various machine learning algorithms, such as Conditional Random Fields (CRFs), have been successfully used for this task. However, these state-of-the-art BNER systems largely depend on hand-crafted features.
Results: We present a recurrent neural network (RNN) framework based on word embeddings and character representation. On top of the neural network architecture, we use a CRF layer to jointly decode labels for the whole sentence. In our approach, contextual information from both directions and long-range dependencies in the sequence, which is useful for this task, can be well modeled by bidirectional variation and long short-term memory (LSTM) unit, respectively. Although our models use word embeddings and character embeddings as the only features, the bidirectional LSTM-RNN (BLSTM-RNN) model achieves state-of-the-art performance - 86.55% F1 on BioCreative II gene mention (GM) corpus and 73.79% F1 on JNLPBA 2004 corpus.
Conclusions: Our neural network architecture can be successfully used for BNER without any manual feature engineering. Experimental results show that domain-specific pre-trained word embeddings and character-level representation can improve the performance of the LSTM-RNN models. On the GM corpus, we achieve comparable performance compared with other systems using complex hand-crafted features. Considering the JNLPBA corpus, our model achieves the best results, outperforming the previously top performing systems. The source code of our method is freely available under GPL at https://github.com/lvchen1989/BNER .
Keywords: Biomedical named entity recognition; Character representation; LSTM; Recurrent neural network; Word embeddings.
Conflict of interest statement
Ethics approval and consent to participate
Not applicable.
Consent for publication
Not applicable.
Competing interests
The authors declare that they have no competing interests.
Publisher’s Note
Springer Nature remains neutral with regard to jurisdictional claims in published maps and institutional affiliations.
Figures
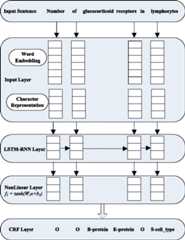
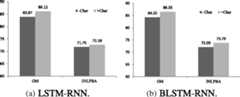
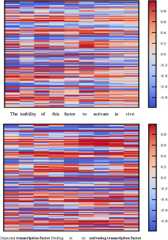
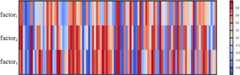
Similar articles
- Character-level neural network for biomedical named entity recognition.Gridach M.Gridach M.J Biomed Inform. 2017 Jun;70:85-91. doi: 10.1016/j.jbi.2017.05.002. Epub 2017 May 11.J Biomed Inform. 2017.PMID:28502909
- Character level and word level embedding with bidirectional LSTM - Dynamic recurrent neural network for biomedical named entity recognition from literature.Gajendran S, D M, Sugumaran V.Gajendran S, et al.J Biomed Inform. 2020 Dec;112:103609. doi: 10.1016/j.jbi.2020.103609. Epub 2020 Oct 26.J Biomed Inform. 2020.PMID:33122119
- Entity recognition from clinical texts via recurrent neural network.Liu Z, Yang M, Wang X, Chen Q, Tang B, Wang Z, Xu H.Liu Z, et al.BMC Med Inform Decis Mak. 2017 Jul 5;17(Suppl 2):67. doi: 10.1186/s12911-017-0468-7.BMC Med Inform Decis Mak. 2017.PMID:28699566Free PMC article.
- A Review on Electronic Health Record Text-Mining for Biomedical Name Entity Recognition in Healthcare Domain.Ahmad PN, Shah AM, Lee K.Ahmad PN, et al.Healthcare (Basel). 2023 Apr 28;11(9):1268. doi: 10.3390/healthcare11091268.Healthcare (Basel). 2023.PMID:37174810Free PMC article.Review.
- A Review of Algorithm & Hardware Design for AI-Based Biomedical Applications.Wei Y, Zhou J, Wang Y, Liu Y, Liu Q, Luo J, Wang C, Ren F, Huang L.Wei Y, et al.IEEE Trans Biomed Circuits Syst. 2020 Apr;14(2):145-163. doi: 10.1109/TBCAS.2020.2974154. Epub 2020 Feb 17.IEEE Trans Biomed Circuits Syst. 2020.PMID:32078560Review.
Cited by
- A hybrid approach for named entity recognition in Chinese electronic medical record.Ji B, Liu R, Li S, Yu J, Wu Q, Tan Y, Wu J.Ji B, et al.BMC Med Inform Decis Mak. 2019 Apr 9;19(Suppl 2):64. doi: 10.1186/s12911-019-0767-2.BMC Med Inform Decis Mak. 2019.PMID:30961597Free PMC article.
- Deep neural model with self-training for scientific keyphrase extraction.Zhu X, Lyu C, Ji D, Liao H, Li F.Zhu X, et al.PLoS One. 2020 May 15;15(5):e0232547. doi: 10.1371/journal.pone.0232547. eCollection 2020.PLoS One. 2020.PMID:32413094Free PMC article.
- Exploring deep learning methods for recognizing rare diseases and their clinical manifestations from texts.Segura-Bedmar I, Camino-Perdones D, Guerrero-Aspizua S.Segura-Bedmar I, et al.BMC Bioinformatics. 2022 Jul 6;23(1):263. doi: 10.1186/s12859-022-04810-y.BMC Bioinformatics. 2022.PMID:35794528Free PMC article.
- A hybrid neural network model for predicting kidney disease in hypertension patients based on electronic health records.Ren Y, Fei H, Liang X, Ji D, Cheng M.Ren Y, et al.BMC Med Inform Decis Mak. 2019 Apr 4;19(Suppl 2):51. doi: 10.1186/s12911-019-0765-4.BMC Med Inform Decis Mak. 2019.PMID:30961614Free PMC article.
- Extraction of chemical-protein interactions from the literature using neural networks and narrow instance representation.Antunes R, Matos S.Antunes R, et al.Database (Oxford). 2019 Jan 1;2019:baz095. doi: 10.1093/database/baz095.Database (Oxford). 2019.PMID:31622463Free PMC article.
References
- Kim JD, Ohta T, Tsuruoka Y, Tateisi Y, Collier N. Proceedings of the International Joint Workshop on Natural Language Processing in Biomedicine and Its Applications. Geneva: Association for Computational Linguistics; 2004. Introduction to the bio-entity recognition task at jnlpba.
- Cho H, Okazaki N, Miwa M, Tsujii J. Nersuite: a named entity recognition toolkit. Tsujii Laboratory, Department of Information Science, University of Tokyo, Tokyo, Japan [http://nersuite.nlplab.org/index.html]. 2010.
MeSH terms
LinkOut - more resources
Full Text Sources
Other Literature Sources