Decoding tumour phenotype by noninvasive imaging using a quantitative radiomics approach
- PMID:24892406
- PMCID: PMC4059926
- DOI: 10.1038/ncomms5006
Decoding tumour phenotype by noninvasive imaging using a quantitative radiomics approach
Erratum in
- Nat Commun. 2014;5:4644. Cavalho, Sara [corrected to Carvalho, Sara]
Abstract
Human cancers exhibit strong phenotypic differences that can be visualized noninvasively by medical imaging. Radiomics refers to the comprehensive quantification of tumour phenotypes by applying a large number of quantitative image features. Here we present a radiomic analysis of 440 features quantifying tumour image intensity, shape and texture, which are extracted from computed tomography data of 1,019 patients with lung or head-and-neck cancer. We find that a large number of radiomic features have prognostic power in independent data sets of lung and head-and-neck cancer patients, many of which were not identified as significant before. Radiogenomics analysis reveals that a prognostic radiomic signature, capturing intratumour heterogeneity, is associated with underlying gene-expression patterns. These data suggest that radiomics identifies a general prognostic phenotype existing in both lung and head-and-neck cancer. This may have a clinical impact as imaging is routinely used in clinical practice, providing an unprecedented opportunity to improve decision-support in cancer treatment at low cost.
Figures
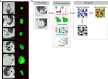
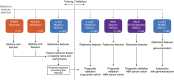
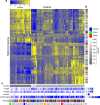
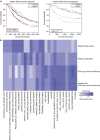
Comment in
- [Vulnerabilities of radiomics: Why the most popular radiomics signature accidentally measured tumor volume].Putz F, Fietkau R.Putz F, et al.Strahlenther Onkol. 2021 Apr;197(4):361-364. doi: 10.1007/s00066-021-01747-8. Epub 2021 Feb 3.Strahlenther Onkol. 2021.PMID:33537912Free PMC article.German.No abstract available.
Similar articles
- Comparison of a radiomic biomarker with volumetric analysis for decoding tumour phenotypes of lung adenocarcinoma with different disease-specific survival.Yuan M, Zhang YD, Pu XH, Zhong Y, Li H, Wu JF, Yu TF.Yuan M, et al.Eur Radiol. 2017 Nov;27(11):4857-4865. doi: 10.1007/s00330-017-4855-3. Epub 2017 May 18.Eur Radiol. 2017.PMID:28523350
- Prediction of malignant and benign of lung tumor using a quantitative radiomic method.Jun Wang, Xia Liu, Di Dong, Jiangdian Song, Min Xu, Yali Zang, Jie Tian.Jun Wang, et al.Annu Int Conf IEEE Eng Med Biol Soc. 2016 Aug;2016:1272-1275. doi: 10.1109/EMBC.2016.7590938.Annu Int Conf IEEE Eng Med Biol Soc. 2016.PMID:28268557
- Robust Radiomics feature quantification using semiautomatic volumetric segmentation.Parmar C, Rios Velazquez E, Leijenaar R, Jermoumi M, Carvalho S, Mak RH, Mitra S, Shankar BU, Kikinis R, Haibe-Kains B, Lambin P, Aerts HJ.Parmar C, et al.PLoS One. 2014 Jul 15;9(7):e102107. doi: 10.1371/journal.pone.0102107. eCollection 2014.PLoS One. 2014.PMID:25025374Free PMC article.
- Radiomic biomarkers for head and neck squamous cell carcinoma.Tanadini-Lang S, Balermpas P, Guckenberger M, Pavic M, Riesterer O, Vuong D, Bogowicz M.Tanadini-Lang S, et al.Strahlenther Onkol. 2020 Oct;196(10):868-878. doi: 10.1007/s00066-020-01638-4. Epub 2020 Jun 3.Strahlenther Onkol. 2020.PMID:32495038Review.
- ¹⁸F-fluorodeoxyglucose-positron emission tomography/computed tomography in malignancies of the thyroid and in head and neck squamous cell carcinoma: a review of the literature.Lauridsen JK, Rohde M, Thomassen A.Lauridsen JK, et al.PET Clin. 2015 Jan;10(1):75-88. doi: 10.1016/j.cpet.2014.09.006. Epub 2014 Nov 22.PET Clin. 2015.PMID:25455881Review.
Cited by
- Morphological similarity and white matter structural mapping of new daily persistent headache: a structural connectivity and tract-specific study.Zhang D, Zong F, Mei Y, Zhao K, Qiu D, Xiong Z, Li X, Tang H, Zhang P, Zhang M, Zhang Y, Yu X, Wang Z, Liu Y, Sui B, Wang Y.Zhang D, et al.J Headache Pain. 2024 Nov 4;25(1):191. doi: 10.1186/s10194-024-01899-9.J Headache Pain. 2024.PMID:39497095Free PMC article.
- Identifying the histologic subtypes of non-small cell lung cancer with computed tomography imaging: a comparative study of capsule net, convolutional neural network, and radiomics.Liu H, Jiao Z, Han W, Jing B.Liu H, et al.Quant Imaging Med Surg. 2021 Jun;11(6):2756-2765. doi: 10.21037/qims-20-734.Quant Imaging Med Surg. 2021.PMID:34079739Free PMC article.
- Predicting Local Failure after Partial Prostate Re-Irradiation Using a Dosiomic-Based Machine Learning Model.Pirrone G, Matrone F, Chiovati P, Manente S, Drigo A, Donofrio A, Cappelletto C, Borsatti E, Dassie A, Bortolus R, Avanzo M.Pirrone G, et al.J Pers Med. 2022 Sep 13;12(9):1491. doi: 10.3390/jpm12091491.J Pers Med. 2022.PMID:36143276Free PMC article.
- Radiomics Based on Multiparametric Magnetic Resonance Imaging to Predict Extraprostatic Extension of Prostate Cancer.Xu L, Zhang G, Zhao L, Mao L, Li X, Yan W, Xiao Y, Lei J, Sun H, Jin Z.Xu L, et al.Front Oncol. 2020 Jun 16;10:940. doi: 10.3389/fonc.2020.00940. eCollection 2020.Front Oncol. 2020.PMID:32612953Free PMC article.
- Radiomics Nomogram for Prediction of Peritoneal Metastasis in Patients With Gastric Cancer.Huang W, Zhou K, Jiang Y, Chen C, Yuan Q, Han Z, Xie J, Yu S, Sun Z, Hu Y, Yu J, Liu H, Xiao R, Xu Y, Zhou Z, Li G.Huang W, et al.Front Oncol. 2020 Aug 20;10:1416. doi: 10.3389/fonc.2020.01416. eCollection 2020.Front Oncol. 2020.PMID:32974149Free PMC article.
References
- Buckler A. J., Bresolin L., Dunnick N. R. & Sullivan D. C. Group. A collaborative enterprise for multi-stakeholder participation in the advancement of quantitative imaging. Radiology 258, 906–914 (2011). - PubMed
- Jaffe C. C. Measures of response: RECIST, WHO, and new alternatives. J. Clin. Oncol. 24, 3245–3251 (2006). - PubMed
Publication types
MeSH terms
Related information
Grants and funding
LinkOut - more resources
Full Text Sources
Other Literature Sources
Medical
Molecular Biology Databases