A Weighted Random Forests Approach to Improve Predictive Performance
- PMID:24501613
- PMCID: PMC3912194
- DOI: 10.1002/sam.11196
A Weighted Random Forests Approach to Improve Predictive Performance
Abstract
Identifying genetic variants associated with complex disease in high-dimensional data is a challenging problem, and complicated etiologies such as gene-gene interactions are often ignored in analyses. The data-mining method Random Forests (RF) can handle high-dimensions; however, in high-dimensional data, RF is not an effective filter for identifying risk factors associated with the disease trait via complex genetic models such as gene-gene interactions without strong marginal components. Here we propose an extension called Weighted Random Forests (wRF), which incorporates tree-level weights to emphasize more accurate trees in prediction and calculation of variable importance. We demonstrate through simulation and application to data from a genetic study of addiction that wRF can outperform RF in high-dimensional data, although the improvements are modest and limited to situations with effect sizes that are larger than what is realistic in genetics of complex disease. Thus, the current implementation of wRF is unlikely to improve detection of relevant predictors in high-dimensional genetic data, but may be applicable in other situations where larger effect sizes are anticipated.
Keywords: Random Forests; gene-gene interactions; genetic data; genome wide association; high-dimensional data; weighting.
Figures
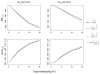
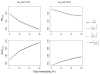
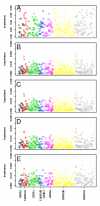
Similar articles
- SNP interaction detection with Random Forests in high-dimensional genetic data.Winham SJ, Colby CL, Freimuth RR, Wang X, de Andrade M, Huebner M, Biernacka JM.Winham SJ, et al.BMC Bioinformatics. 2012 Jul 15;13:164. doi: 10.1186/1471-2105-13-164.BMC Bioinformatics. 2012.PMID:22793366Free PMC article.
- Genome-wide association data classification and SNPs selection using two-stage quality-based Random Forests.Nguyen TT, Huang J, Wu Q, Nguyen T, Li M.Nguyen TT, et al.BMC Genomics. 2015;16 Suppl 2(Suppl 2):S5. doi: 10.1186/1471-2164-16-S2-S5. Epub 2015 Jan 21.BMC Genomics. 2015.PMID:25708662Free PMC article.
- A comparative study of forest methods for time-to-event data: variable selection and predictive performance.Liu Y, Zhou S, Wei H, An S.Liu Y, et al.BMC Med Res Methodol. 2021 Sep 25;21(1):193. doi: 10.1186/s12874-021-01386-8.BMC Med Res Methodol. 2021.PMID:34563138Free PMC article.
- Multigenic modeling of complex disease by random forests.Sun YV.Sun YV.Adv Genet. 2010;72:73-99. doi: 10.1016/B978-0-12-380862-2.00004-7.Adv Genet. 2010.PMID:21029849Review.
- Random forests for genetic association studies.Goldstein BA, Polley EC, Briggs FB.Goldstein BA, et al.Stat Appl Genet Mol Biol. 2011;10(1):32. doi: 10.2202/1544-6115.1691. Epub 2011 Jul 12.Stat Appl Genet Mol Biol. 2011.PMID:22889876Free PMC article.Review.
Cited by
- PM2.5 Is Insufficient to Explain Personal PAH Exposure.Bramer LM, Dixon HM, Rohlman D, Scott RP, Miller RL, Kincl L, Herbstman JB, Waters KM, Anderson KA.Bramer LM, et al.Geohealth. 2024 Feb 10;8(2):e2023GH000937. doi: 10.1029/2023GH000937. eCollection 2024 Feb.Geohealth. 2024.PMID:38344245Free PMC article.
- Differentiation of Clear Cell and Non-clear-cell Renal Cell Carcinoma through CT-based Radiomics Models and Nomogram.Cheng D, Abudikeranmu Y, Tuerdi B.Cheng D, et al.Curr Med Imaging. 2023;19(9):1005-1017. doi: 10.2174/1573405619666221121164235.Curr Med Imaging. 2023.PMID:36411581Free PMC article.
- What makes a good prediction? Feature importance and beginning to open the black box of machine learning in genetics.Musolf AM, Holzinger ER, Malley JD, Bailey-Wilson JE.Musolf AM, et al.Hum Genet. 2022 Sep;141(9):1515-1528. doi: 10.1007/s00439-021-02402-z. Epub 2021 Dec 4.Hum Genet. 2022.PMID:34862561Free PMC article.Review.
- National Veterans Health Administration inpatient risk stratification models for hospital-acquired acute kidney injury.Cronin RM, VanHouten JP, Siew ED, Eden SK, Fihn SD, Nielson CD, Peterson JF, Baker CR, Ikizler TA, Speroff T, Matheny ME.Cronin RM, et al.J Am Med Inform Assoc. 2015 Sep;22(5):1054-71. doi: 10.1093/jamia/ocv051. Epub 2015 Jun 23.J Am Med Inform Assoc. 2015.PMID:26104740Free PMC article.
- Predicting Anticoagulation Need for Otogenic Intracranial Sinus Thrombosis: A Machine Learning Approach.Kaufmann MR, Camilon PR, Levi JR, Devaiah AK.Kaufmann MR, et al.J Neurol Surg B Skull Base. 2021 Apr;82(2):233-243. doi: 10.1055/s-0040-1713105. Epub 2020 Oct 5.J Neurol Surg B Skull Base. 2021.PMID:33777638Free PMC article.
References
- McCarthy MI, Abecasis GR, Cardon LR, Goldstein DB, Little J, Ioannidis JP, Hirschhorn JN. Genome-wide association studies for complex traits: consensus, uncertainty and challenges. Nature reviews Genetics. 2008;9(5):356–369. - PubMed
- Breiman L. Random Forests. Mach Learn. 2001;45:5–32.
Grants and funding
LinkOut - more resources
Full Text Sources
Other Literature Sources