AutoDensity: an automated method to measure mammographic breast density that predicts breast cancer risk and screening outcomes
- PMID:24020331
- PMCID: PMC3978575
- DOI: 10.1186/bcr3474
AutoDensity: an automated method to measure mammographic breast density that predicts breast cancer risk and screening outcomes
Abstract
Introduction: While Cumulus - a semi-automated method for measuring breast density - is utilised extensively in research, it is labour-intensive and unsuitable for screening programmes that require an efficient and valid measure on which to base screening recommendations. We develop an automated method to measure breast density (AutoDensity) and compare it to Cumulus in terms of association with breast cancer risk and breast cancer screening outcomes.
Methods: AutoDensity automatically identifies the breast area in the mammogram and classifies breast density in a similar way to Cumulus, through a fast, stand-alone Windows or Linux program. Our sample comprised 985 women with screen-detected cancers, 367 women with interval cancers and 4,975 controls (women who did not have cancer), sampled from first and subsequent screening rounds of a film mammography screening programme. To test the validity of AutoDensity, we compared the effect estimates using AutoDensity with those using Cumulus from logistic regression models that tested the association between breast density and breast cancer risk, risk of small and large screen-detected cancers and interval cancers, and screening programme sensitivity (the proportion of cancers that are screen-detected). As a secondary analysis, we report on correlation between AutoDensity and Cumulus measures.
Results: AutoDensity performed similarly to Cumulus in all associations tested. For example, using AutoDensity, the odds ratios for women in the highest decile of breast density compared to women in the lowest quintile for invasive breast cancer, interval cancers, large and small screen-detected cancers were 3.2 (95% CI 2.5 to 4.1), 4.7 (95% CI 3.0 to 7.4), 6.4 (95% CI 3.7 to 11.1) and 2.2 (95% CI 1.6 to 3.0) respectively. For Cumulus the corresponding odds ratios were: 2.4 (95% CI 1.9 to 3.1), 4.1 (95% CI 2.6 to 6.3), 6.6 (95% CI 3.7 to 11.7) and 1.3 (95% CI 0.9 to 1.8). Correlation between Cumulus and AutoDensity measures was 0.63 (P < 0.001).
Conclusions: Based on the similarity of the effect estimates for AutoDensity and Cumulus inmodels of breast density and breast cancer risk and screening outcomes, we conclude that AutoDensity is a valid automated method for measuring breast density from digitised film mammograms.
Figures
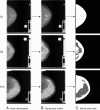
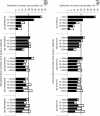
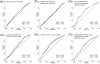
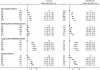
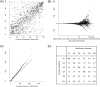
Similar articles
- A comparison of five methods of measuring mammographic density: a case-control study.Astley SM, Harkness EF, Sergeant JC, Warwick J, Stavrinos P, Warren R, Wilson M, Beetles U, Gadde S, Lim Y, Jain A, Bundred S, Barr N, Reece V, Brentnall AR, Cuzick J, Howell T, Evans DG.Astley SM, et al.Breast Cancer Res. 2018 Feb 5;20(1):10. doi: 10.1186/s13058-018-0932-z.Breast Cancer Res. 2018.PMID:29402289Free PMC article.
- Mammographic density defined by higher than conventional brightness threshold better predicts breast cancer risk for full-field digital mammograms.Nguyen TL, Aung YK, Evans CF, Yoon-Ho C, Jenkins MA, Sung J, Hopper JL, Song YM.Nguyen TL, et al.Breast Cancer Res. 2015 Nov 18;17:142. doi: 10.1186/s13058-015-0654-4.Breast Cancer Res. 2015.PMID:26581435Free PMC article.
- Predicting interval and screen-detected breast cancers from mammographic density defined by different brightness thresholds.Nguyen TL, Aung YK, Li S, Trinh NH, Evans CF, Baglietto L, Krishnan K, Dite GS, Stone J, English DR, Song YM, Sung J, Jenkins MA, Southey MC, Giles GG, Hopper JL.Nguyen TL, et al.Breast Cancer Res. 2018 Dec 13;20(1):152. doi: 10.1186/s13058-018-1081-0.Breast Cancer Res. 2018.PMID:30545395Free PMC article.
- Supplemental Screening for Breast Cancer in Women With Dense Breasts: A Systematic Review for the U.S. Preventive Services Task Force.Melnikow J, Fenton JJ, Whitlock EP, Miglioretti DL, Weyrich MS, Thompson JH, Shah K.Melnikow J, et al.Ann Intern Med. 2016 Feb 16;164(4):268-78. doi: 10.7326/M15-1789. Epub 2016 Jan 12.Ann Intern Med. 2016.PMID:26757021Free PMC article.Review.
- Breast cancer screening in the era of density notification legislation: summary of 2014 Massachusetts experience and suggestion of an evidence-based management algorithm by multi-disciplinary expert panel.Freer PE, Slanetz PJ, Haas JS, Tung NM, Hughes KS, Armstrong K, Semine AA, Troyan SL, Birdwell RL.Freer PE, et al.Breast Cancer Res Treat. 2015 Sep;153(2):455-64. doi: 10.1007/s10549-015-3534-9. Epub 2015 Aug 20.Breast Cancer Res Treat. 2015.PMID:26290416Free PMC article.Review.
Cited by
- A Review on Automatic Mammographic Density and Parenchymal Segmentation.He W, Juette A, Denton ER, Oliver A, Martí R, Zwiggelaar R.He W, et al.Int J Breast Cancer. 2015;2015:276217. doi: 10.1155/2015/276217. Epub 2015 Jun 11.Int J Breast Cancer. 2015.PMID:26171249Free PMC article.Review.
- Understanding mammographic breast density profile in China: A Sino-Australian comparative study of breast density using real-world data from cancer screening programs.Li T, Li J, Heard R, Gandomkar Z, Ren J, Dai M, Brennan P.Li T, et al.Asia Pac J Clin Oncol. 2022 Dec;18(6):696-705. doi: 10.1111/ajco.13763. Epub 2022 Mar 3.Asia Pac J Clin Oncol. 2022.PMID:35238173Free PMC article.
- Automatic Estimation of Volumetric Breast Density Using Artificial Neural Network-Based Calibration of Full-Field Digital Mammography: Feasibility on Japanese Women With and Without Breast Cancer.Wang J, Kato F, Yamashita H, Baba M, Cui Y, Li R, Oyama-Manabe N, Shirato H.Wang J, et al.J Digit Imaging. 2017 Apr;30(2):215-227. doi: 10.1007/s10278-016-9922-9.J Digit Imaging. 2017.PMID:27832519Free PMC article.
- Long-term excess risk of breast cancer after a single breast density measurement.Rebolj M, Blyuss O, Chia KS, Duffy SW.Rebolj M, et al.Eur J Cancer. 2019 Aug;117:41-47. doi: 10.1016/j.ejca.2019.05.009. Epub 2019 Jun 21.Eur J Cancer. 2019.PMID:31229948Free PMC article.
- Fully Automated Breast Density Segmentation and Classification Using Deep Learning.Saffari N, Rashwan HA, Abdel-Nasser M, Kumar Singh V, Arenas M, Mangina E, Herrera B, Puig D.Saffari N, et al.Diagnostics (Basel). 2020 Nov 23;10(11):988. doi: 10.3390/diagnostics10110988.Diagnostics (Basel). 2020.PMID:33238512Free PMC article.
References
- Screening Monograph No 4/2009. Prepared by the National Breast and Ovarian Cancer Centre for the Australian Government Department of Health and Ageing. Canberra: Australian Government; 2009. BreastScreen Australia evaluation: mortality (ecological) study.
Publication types
MeSH terms
Related information
LinkOut - more resources
Full Text Sources
Other Literature Sources
Medical
Research Materials