FUBAR: a fast, unconstrained bayesian approximation for inferring selection
- PMID:23420840
- PMCID: PMC3670733
- DOI: 10.1093/molbev/mst030
FUBAR: a fast, unconstrained bayesian approximation for inferring selection
Abstract
Model-based analyses of natural selection often categorize sites into a relatively small number of site classes. Forcing each site to belong to one of these classes places unrealistic constraints on the distribution of selection parameters, which can result in misleading inference due to model misspecification. We present an approximate hierarchical Bayesian method using a Markov chain Monte Carlo (MCMC) routine that ensures robustness against model misspecification by averaging over a large number of predefined site classes. This leaves the distribution of selection parameters essentially unconstrained, and also allows sites experiencing positive and purifying selection to be identified orders of magnitude faster than by existing methods. We demonstrate that popular random effects likelihood methods can produce misleading results when sites assigned to the same site class experience different levels of positive or purifying selection--an unavoidable scenario when using a small number of site classes. Our Fast Unconstrained Bayesian AppRoximation (FUBAR) is unaffected by this problem, while achieving higher power than existing unconstrained (fixed effects likelihood) methods. The speed advantage of FUBAR allows us to analyze larger data sets than other methods: We illustrate this on a large influenza hemagglutinin data set (3,142 sequences). FUBAR is available as a batch file within the latest HyPhy distribution (http://www.hyphy.org), as well as on the Datamonkey web server (http://www.datamonkey.org/).
Figures
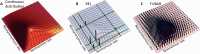

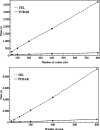
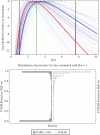







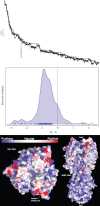




Similar articles
- bModelTest: Bayesian phylogenetic site model averaging and model comparison.Bouckaert RR, Drummond AJ.Bouckaert RR, et al.BMC Evol Biol. 2017 Feb 6;17(1):42. doi: 10.1186/s12862-017-0890-6.BMC Evol Biol. 2017.PMID:28166715Free PMC article.
- Bayesian random local clocks, or one rate to rule them all.Drummond AJ, Suchard MA.Drummond AJ, et al.BMC Biol. 2010 Aug 31;8:114. doi: 10.1186/1741-7007-8-114.BMC Biol. 2010.PMID:20807414Free PMC article.
- Phylogenetic MCMC algorithms are misleading on mixtures of trees.Mossel E, Vigoda E.Mossel E, et al.Science. 2005 Sep 30;309(5744):2207-9. doi: 10.1126/science.1115493.Science. 2005.PMID:16195459
- Identifiability of parameters in MCMC Bayesian inference of phylogeny.Rannala B.Rannala B.Syst Biol. 2002 Oct;51(5):754-60. doi: 10.1080/10635150290102429.Syst Biol. 2002.PMID:12396589
- Markov chain Monte Carlo inference for Markov jump processes via the linear noise approximation.Stathopoulos V, Girolami MA.Stathopoulos V, et al.Philos Trans A Math Phys Eng Sci. 2012 Dec 31;371(1984):20110541. doi: 10.1098/rsta.2011.0541. Print 2013 Feb 13.Philos Trans A Math Phys Eng Sci. 2012.PMID:23277599
Cited by
- Phylogenetic Inference of the 2022 Highly Pathogenic H7N3 Avian Influenza Outbreak in Northern Mexico.Navarro-Lopez R, Xu W, Gomez-Romero N, Velazquez-Salinas L, Berhane Y.Navarro-Lopez R, et al.Pathogens. 2022 Nov 1;11(11):1284. doi: 10.3390/pathogens11111284.Pathogens. 2022.PMID:36365034Free PMC article.
- The strength of selection is consistent across both domains of the MHC class I peptide-binding groove in birds.Minias P, He K, Dunn PO.Minias P, et al.BMC Ecol Evol. 2021 May 8;21(1):80. doi: 10.1186/s12862-021-01812-x.BMC Ecol Evol. 2021.PMID:33964878Free PMC article.
- Whole genome characteristics of hedgehog coronaviruses from Poland and analysis of the evolution of the Spike protein for its interspecies transmission potential.Domanska-Blicharz K, Lisowska A, Opolska J, Ruszkowski JJ, Gogulski M, Pomorska-Mól M.Domanska-Blicharz K, et al.BMC Vet Res. 2024 Sep 21;20(1):424. doi: 10.1186/s12917-024-04277-4.BMC Vet Res. 2024.PMID:39304831Free PMC article.
- Global Prevalence and Hemagglutinin Evolution of H7N9 Avian Influenza Viruses from 2013 to 2022.Liu Q, Zeng H, Wu X, Yang X, Wang G.Liu Q, et al.Viruses. 2023 Nov 4;15(11):2214. doi: 10.3390/v15112214.Viruses. 2023.PMID:38005891Free PMC article.
- Genomic characterization and evolution of SARS-CoV-2 of a Canadian population.Zhang M, Li L, Luo M, Liang B.Zhang M, et al.PLoS One. 2021 Mar 4;16(3):e0247799. doi: 10.1371/journal.pone.0247799. eCollection 2021.PLoS One. 2021.PMID:33662015Free PMC article.
References
- Anisimova M, Kosiol C. Investigating protein-coding sequence evolution with probabilistic codon substitution models. Mol Biol Evol. 2009;26:255–271. - PubMed
- Bush RM, Fitch WM, Bender CA, Cox NJ. Positive selection on the H3 hemagglutinin gene of human influenza virus A. Mol Biol Evol. 1999;16:1457–1465. - PubMed
- Cadar D, Cságola A, Kiss T, Tuboly T. Capsid protein evolution and comparative phylogeny of novel porcine parvoviruses. Mol Phylogenet Evol. 2013;66:243–253. - PubMed
- Caton AJ, Brownlee GG, Yewdell JW, Gerhard W. The antigenic structure of the influenza virus A/PR/8/34 hemagglutinin (H1 subtype) Cell. 1982;31:417–427. - PubMed
- Corti D, Voss J, Gamblin SJ, et al. (23 co-authors) A neutralizing antibody selected from plasma cells that binds to group 1 and group 2 influenza A hemagglutinins. Science. 2011;333:850–856. - PubMed
Publication types
MeSH terms
Related information
Grants and funding
LinkOut - more resources
Full Text Sources
Other Literature Sources