the Creative Commons Attribution 4.0 License.
the Creative Commons Attribution 4.0 License.
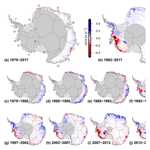
Four decades of Antarctic surface elevation changes from multi-mission satellite altimetry
We developed a multi-mission satellite altimetry analysis over the AntarcticIce Sheet which comprises Seasat, Geosat, ERS-1, ERS-2, Envisat, ICESat andCryoSat-2. After a consistent reprocessing and a stepwise calibration of theinter-mission offsets, we obtained monthly grids of multi-mission surfaceelevation change (SEC) with respect to the reference epoch09/2010 (in the format of month/year) from 1978 to 2017. A validation with independent elevationchanges from in situ and airborne observations as well as a comparison with afirn model proves that the different missions and observation modes have beensuccessfully combined to a seamless multi-mission time series. For coastalEast Antarctica, even Seasat and Geosat provide reliable information and,hence, allow for the analysis of four decades of elevation changes. Thespatial and temporal resolution of our result allows for the identificationof when and where significant changes in elevation occurred. These timeseries add detailed information to the evolution of surface elevation in suchkey regions as Pine Island Glacier, Totten Glacier, Dronning Maud Land orLake Vostok. After applying a density mask, we calculated time series of masschanges and found that the Antarctic Ice Sheet north of 81.5∘ S waslosing mass at an average rate of Gt yr−1 between 1992 and2017, which accelerated to Gt yr−1 after 2010.
- Article
(9419 KB) - Full-text XML
- Supplement
(2797 KB) - BibTeX
- EndNote
Satellite altimetry is fundamental for detecting and understanding changes inthe Antarctic Ice Sheet (AIS;Rémy and Parouty, 2009;Shepherd et al., 2018).Since 1992, altimeter missions have revealed dynamic thinning of severaloutlet glaciers of the West Antarctica Ice Sheet (WAIS) and have put narrow limits on elevationchanges in most parts of East Antarctica. Rates of surface elevation changeare not constant in time(Shepherd et al., 2012). Ice flow acceleration hascaused dynamic thinning to accelerate(Mouginot et al., 2014;Hogg et al., 2017).Variations in surface mass balance (SMB) and firn compaction rate also causeinterannual variations of surface elevation(Horwath et al., 2012;Shepherd et al., 2012;Lenaerts et al., 2013). Consequently, differentrates of change have been reported from altimeter missions that coverdifferent time intervals. For example, ERS-1 and ERS-2 data over the interval1992–2003 revealed negative elevation rates in eastern Dronning Maud Landand Enderby Land (25–60∘ E) and positive rates in PrincessElizabeth Land (70–100∘ E)(Wingham et al., 2006b), while Envisat dataover the interval 2003–2010 revealed the opposite pattern(Flament and Rémy, 2012). Two large snowfall events in 2009 and 2011 inducedstepwise elevation changes in Dronning Maud Land(Lenaerts et al., 2013;Shepherd et al., 2012).
As a consequence, mean linear rates derived from a single mission havelimited significance in characterizing the long-term evolution of the icesheet(Wouters et al., 2013). Data from different altimeter missions need to belinked over a time span that is as long as possible in order to better distinguishand understand the long-term evolution and the natural variability of icesheet volume and mass.
Missions with similar sensor characteristics have been combined, e.g., byWingham et al. (2006b, ERS-1 and ERS-2) andLi and Davis (2008, ERS-2 andEnvisat).Fricker and Padman (2012) use Seasat, ERS-1, ERS-2 andEnvisat to determine elevation changes of Antarctic ice shelves. They applyconstant biases, determined over open ocean, to cross-calibrate the missions.In contrast to ocean-based calibration,Zwally et al. (2005) foundsignificant differences for the biases over ice sheets with a distinctspatial pattern(see also Frappart et al., 2016).Khvorostovsky (2012)showed that the correction of inter-mission offsets over an ice sheet is nottrivial.Paolo et al. (2016) cross-calibrated ERS-1, ERS-2 and Envisat on eachgrid cell using overlapping epochs, andAdusumilli et al. (2018) extended thesetime series by including CryoSat-2 data. We use a very similar approach forconventional radar altimeter measurements with overlapping mission periods.Moreover, we also include measurements of the nonoverlapping missions Seasatand Geosat and measurements with different sensor characteristics, such asICESat laser altimetry or CryoSat-2 interferometric synthetic aperture radar(SARIn) mode, making the combination of the observations even morechallenging.
Here we present an approach for combining seven different satellite altimetrymissions over the AIS. Using refined waveform retracking and slope correctionof the radar altimetry (RA) data, we ensure the consistency of the surfaceelevation measurements and improve their precision by up to 50 %. In thefollowing stepwise procedure, we first process the measurements from allmissions jointly using the repeat-altimetry method. We then form monthly timeseries for each individual mission data set. Finally, we merge all timeseries from both radar and laser altimetry. For this last step, we employdifferent approaches of inter-mission offset estimation, depending on thetemporal overlap or nonoverlap of the missions and on the similarity ordissimilarity of their altimeter sensors.
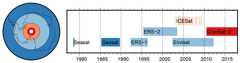
Figure 1Spatial and temporal coverage of the satellite altimetry data usedin this study. The colors denote the maximum southern extent of themeasurements (dark blue: 72∘ S, light blue: 81.5∘ S,orange: 86∘ S, red: 88∘ S) and thus the size of therespective polar gap.
We arrive at consistent and seamless time series of gridded surface elevationdifferences with respect to a reference epoch (09/2010; in the format of month/year) which we madepublicly available athttps://doi.pangaea.de/10.1594/PANGAEA.897390(Schröder et al., 2019). The resulting monthly grids with a 10 kmspatial resolution were obtained by smoothing with a moving window over3 months and a spatial Gaussian weighting with2σ=20 km. Weevaluate our results and their estimated uncertainties by a comparison withindependent in situ and airborne data sets, satellite gravimetry estimatesand regional climate model outputs. We illustrate that these time series ofsurface elevation change (SEC) allow for the study of geometry changes andderived mass changes of the AIS in unprecedented detail. The recent elevationchanges of Pine Island Glacier in West Antarctica, Totten Glacier in EastAntarctica and Shirase Glacier of Dronning Maud Land in East Antarctica areput in context with the extended time series from satellite altimetry.Finally, we calculate ice sheet mass balances from these data for the coveredregions. A comparison with independent data indicates a high consistency ofthe different data sets but also reveals remaining discrepancies.
2.1Altimetry data
We use the ice sheet surface elevation observations from seven satellitealtimetry missions: Seasat, Geosat, ERS-1, ERS-2, Envisat, ICESat andCryoSat-2. Figure 1 gives an overview of theirtemporal and spatial coverage. The data of the two early missions, Seasat andGeosat, were obtained from the Radar Ice Altimetry project at Goddard SpaceFlight Center (GSFC). For the ESA missions, we used the data of the REAPERreprocessing project(Brockley et al., 2017) of ERS-1 and ERS-2, the RA-2Sensor and Geophysical Data Record (SGDR) of Envisat in version 2.1 andBaseline C Level 2I data of CryoSat-2. For ICESat we used GLA12 ofrelease 633 from the National Snow and Ice Data Center (NSIDC). Furtherdetails concerning the data set versions used and the data-editing criteriaapplied to remove corrupted measurements in a preprocessing step are given inthe Supplement.
As illustrated in Fig. 1, due to the inclinationof 108∘, Seasat and Geosat measurements only cover the coastalregions of the East Antarctic Ice Sheet (EAIS) and the northern tip of theAntarctic Peninsula Ice Sheet (APIS) north of 72∘ S, which is about25 % of the total ice sheet area. With the launch of ERS-1, the polar gapwas reduced to areas south of 81.5∘ S, resulting in coverage of79 % of the area. The polar gap is even smaller for ICESat(86∘ S) and CryoSat-2 (88∘ S), leading to a nearly completecoverage of the AIS in recent epochs.
ERS-1 and ERS-2 measurements were performed in two different modes,distinguished by the width of the tracking time window and the correspondingtemporal resolution of the recorded waveform. The ice mode is coarser thanthe ocean mode, in order to increase the chance of capturing the radar returnfrom rough topographic surfaces(Scott et al., 1994). While the ice mode wasemployed for the majority of measurements, a significant number ofobservations have also been performed in the ocean mode over Antarctica (22 %for ERS-1, 2 % for ERS-2). We use the data from both modes, as the oceanmode provides a higher precision while the ice mode is more reliable in steepterrain (see Figs. S1 and S3 in the Supplement). However, as there is a regionally varying biasbetween the modes, we treat them as two separate data sets, similar toPaolo et al. (2016).
2.2Reprocessing of radar altimetry
Compared to measurements over the global oceans, pulse-limited radaraltimetry (PLRA) over ice sheets requires a specific processing to accountfor the effects of topography and the dielectric properties of the surface(Bamber, 1994). To ensure consistency in the analysis of PLRAmeasurements, processed and provided by different institutions, we appliedour own method for retracking and slope correction.
The slope correction is applied to account for the effect of topographywithin the beam-limited footprint(Brenner et al., 1983). Different approachesexist to apply a correction(Bamber, 1994), but this effect is still amain source of error in RA over ice sheets. The “direct method” uses thesurface slope within the beam-limited footprint to obtain a correction forthe measurement in the nadir direction. In contrast, the “relocationmethod” relates the measurement towards the likely true position up slope.While the direct method has the advantage that the measurement location isunchanged, which allows an easier calculation of profile crossovers orrepeat-track parameters, the relocation method has lower intrinsic error(Bamber, 1994). A validation using crossovers with kinematicGNSS-profiles(Schröder et al., 2017) showed that, especially in coastalregions, the direct method leads to significantly larger offsets and standarddeviations, compared to the relocation method.Roemer et al. (2007) developeda refined version of the relocation method, using the full information of adigital elevation model (DEM) to locate the point of closest approach(POCA) within the approximately20 km beam-limited footprint. We applied this method in ourreprocessing chain using the DEM ofHelm et al. (2014). The CryoSat-2measurements, used for this DEM, have very dense coverage, and hence verylittle interpolation is necessary. Compared to the DEM ofBamber et al. (2009), this significantly improves the spatial consistency. Weoptimized the approach ofRoemer et al. (2007) with respect to computationalefficiency for application over the entire ice sheet. Instead of searchingthe POCA with the help of a moving window of 2 km (which representsthe pulse-limited footprint) in the DEM-to-satellite grid, we applied aGaussian filter withσ=1 km to the DEM itself to resemble thecoverage of a pulse-limited footprint. Hence, instead of the closest windowaverage, we can simply search for the closest cell in the smoothed grid,which we use as the coarse POCA location. In order to achieve a subgrid POCAlocation, we fit a biquadratic function to the satellite-to-surface distancewithin a3×3 grid cell environment around the coarse POCA grid celland determine the POCA according to this function.
The retracking of the return signal waveform is another important componentin the processing of RA data over ice sheets(Bamber, 1994). Functionalfit approaches(e.g., Martin et al., 1983;Davis, 1992;Legrésy et al., 2005;Wingham et al., 2006b) are wellestablished and allow the interpretation of the obtained waveform shapeparameters with respect to surface and subsurface characteristics(e.g., Lacroix et al., 2008;Nilsson et al., 2015). However, the alternative approachof threshold retrackers has proven to be more precise in terms ofrepeatability(Davis, 1997;Nilsson et al., 2016;Schröder et al., 2017). A very robustvariant is called ICE-1, using the “offset center of gravity” (OCOG)amplitude(Wingham et al., 1986). Compared to the waveform maximum, theOCOG amplitude is significantly less affected by noise(Bamber, 1994).Davis (1997) compared different retrackers and showed that a threshold-based retracker produces remarkably higher-precision results (especially with a lowthreshold as 10 %), compared to functional-fit-based results. Weimplemented three threshold levels (10 %, 20 % and 50 %) for theOCOG amplitude, which allowed us to analyze the influence of the choice ofthis level, similar toDavis (1997).
In addition to PLRA, we also use the SARIn mode data of CryoSat-2,reprocessed byHelm et al. (2014). The difference with respect to theprocessing by ESA mainly consisted of a refined determination of theinterferometric phase and of the application of a threshold retracker.
2.3Accuracy and precision
The accuracy of RA-derived ice surface elevation measurements has beenassessed previously by a crossover comparison with independent validationdata such as the ICESat laser observations(Brenner et al., 2007), airbornelidar(Nilsson et al., 2016) and ground-based GNSS profiles(Schröder et al., 2017). Besides the offset due to snowpack penetration andinstrumental calibration over flat terrain, these assessments revealed that,with increasingly rough surface topography, the RA measurements showsystematically higher elevations than the validation data. These topography-related offsets can be explained by the fact that for surfaces that undulatewithin the∼20 km beam-limited footprint, the radarmeasurements tend to refer to local topographic maxima (the POCA), while thevalidation data from ground-based GNSS profiles or ICESat-based profilesrepresent the full topography. The standard deviation of differences betweenRA data and validation data contains information about the measurement noisebut is additionally influenced by the significantly different sampling of arough surface as well. While over flat terrain, this standard deviation isbelow 50 cm for most satellite altimeter data sets, it can reach 10 m and more in coastal regions. However, both types of error relate tothe different sampling of topography of the respective observationtechniques. An elevation change, detected from within the same technique, isnot influenced by these effects. Hence, with respect to elevation changes,not the accuracy but the precision (i.e., the repeatability) has to beconsidered.
This precision can be studied using intra-mission crossovers betweenascending and descending profiles. Here, the precision of a singlemeasurement is obtained by as two profilescontribute to this difference. To reduce the influence of significant realsurface elevation changes between the two passes, we only consider crossoverswith a time difference of less than 31 days. In stronger inclined topography,the precision of the slope correction dominates the measurement error(Bamber, 1994). Hence, to provide meaningful results, the surface slopeneeds to be taken into consideration. We calculate the slope from theCryoSat-2 DEM(Helm et al., 2014). The absence of slope-related effects on flatterrain allows for the study of the influence of the retracker (denoted as noisehere). With increasing slope, the additional error due to topographic effectscan be identified.
A comparison of the crossover errors of our reprocessed data and of thestandard products shows significant improvements achieved by ourreprocessing. Figure 2 shows this comparison forEnvisat (similar plots for each data set can be found in Fig. S1), binned into groups of 0.05∘ of specific surface slope. Theresults for a flat topography show that a 10 % threshold provides thehighest precision, which confirms the findings ofDavis (1997). Forhigher slopes, we see that our refined slope correction also contributed to amajor improvement. A constant noise levelσnoise and a quadratic,slope-related termσslope have been fitted to the data according to, wheres is inthe unit of degrees. The results in Table 1 showthat for each of the PLRA data sets of ERS-1, ERS-2 and Envisat, themeasurement noise could be reduced by more than 50 % compared to the ESAproduct which uses the functional fit retracker ICE-2(Legrésy and Rémy, 1997).With respect to the CryoSat-2 standard retracker(Wingham et al., 2006a), theimprovement is even larger. Improvements are also significant for theslope-related component. For the example of Envisat and a slope of1∘, the slope-related component is 1.03 m for the ESA productand only 0.37 m for the reprocessed data. The advancedinterferometric processing of the SARIn data achieved similar improvements.For the two early missions Seasat and Geosat, the crossover error of ourreprocessed profiles is similar to that of the original data set from GSFC.However, the number of crossover points is significantly increased,especially for Geosat (see Fig. S1). This means that our reprocessingobtained reliable data where the GSFC processor rejected the measurements.
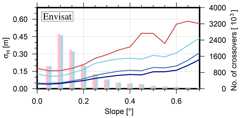
Figure 2Precision of different processing versions of Envisat measurementsfrom near-time (<31 days) crossovers, binned against slope. Red curve: ESAversion with ICE-2 retracker and relocated by mean surface slope. Light,medium and dark blue curves: data reprocessed in this study with 50 %-,20 %- and 10 %-threshold retracker, relocated using the refinedmethod. Vertical bars: number of crossovers for the ESA (red) and our10 % threshold retracked data (blue).
Table 1Noise level and slope-related component (s in degrees) of themeasurement precision, fitted to near-time crossovers (unit:m) of thedata from the respective data center and our reprocessed data (with a10 % threshold retracker applied).
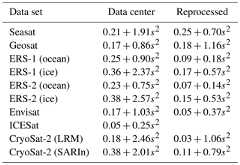
Note that the slope-dependent component is weakly determined for data sets with poor tracking in rugged terrain such as Seasat, Geosat or the ERS ocean mode and for the LRM mode of CryoSat-2.
In addition to measurement noise, reflected in the crossover differences, aconsistent pattern of offsets between ascending and descending tracks hasbeen observed previously(A–D bias; Legrésy et al., 1999;Arthern et al., 2001).Legrésy et al. (1999) interpret this pattern as an effect of the interactionof the linearly polarized radar signal with wind-induced surface structures,whileArthern et al. (2001) attribute the differences to anisotropy within thesnowpack.Helm et al. (2014) showed that a low threshold retrackersignificantly reduces the A–D bias. We observe a similar major reduction(from±1 m in some regions for a functional fit retracker to±15 cm when using a 10 % threshold; see Fig. S2). Theremaining bias is not larger, in its order of magnitude, than the respectivenoise. Moreover, near the ice sheet margins, the determination of meaningfulA–D biases is complicated by the broad statistical distribution of A–Ddifferences and the difficulty of discriminating outliers. We therefore do notapply a systematic A–D bias as a correction but rather include its effect inthe uncertainty estimate of our final result.
3.1Repeat-track parameter fit
We obtain elevation time series following the repeat-track approach, similartoLegrésy et al. (2006) andFlament and Rémy (2012). As the orbits of themissions used here have different repeat-track patterns, instead ofalong-track boxes we perform our fit on a regular grid with 1 kmspacing(as in Helm et al., 2014). For each grid cell we analyze allelevation measurementshi within a radius of 1 km around the gridcell center. This size seems reasonable as for a usual along-track spacing ofabout 350 m for PLRA(Rémy and Parouty, 2009), each track will have up to five measurements within the radius. Due to the size of the pulse-limitedfootprint, a smaller search radius would contain only PLRA measurements withvery redundant topographic information and thus would not be suitable to fita reliable correction for the topography. As specified in Eq. (1), the parameters contain a linear trend (dh∕dt), a planartopography (a0,a1,a2) and a regression coefficient (dBS) for theanomaly of backscattered power (bs) to account forvariations in the penetration depth of the radar signal.
For a single mission, the parameters are adjusted according to the followingmodel:
Here,ti denotes the time of the observation. The reference epocht0 isset to 09/2010.xi andyi are the polar stereographic coordinates ofthe measurement location, reduced by the coordinates of the cell's center.The residual resi describes the misfit between the observation and theestimated parameters.
To account for varying penetration depths due to variations in theelectromagnetic properties of the ice sheet surface, different approachesexist.Wingham et al. (1998),Davis and Ferguson (2004),McMillan et al. (2014) andZwally et al. (2015) apply a linear regression using the backscattered power.Flament and Rémy (2012),Michel et al. (2014) andSimonsen and Sørensen (2017) use twoadditional waveform shape parameters, obtained from functional fitretrackers.Nilsson et al. (2016) showed that a low threshold retrackermitigates the need for a complex waveform shape correction. Hence, we decidedto use a solely backscatter-related correction.
Besides the parameters in Eq. (1),McMillan et al. (2014) andSimonsen and Sørensen (2017) estimate an additional orbit-direction-relatedparameter to account for A–D biases. In Sect. 2.3 we showedthat these biases are significantly reduced due to reprocessing with alow threshold retracker. A further reduction of possible remaining artifactsof A–D biases is achieved by smoothing in the merging step inSect. 3.3.3. The weighted averaging of the results over adiameter of 60 km leads to a balanced ratio of ascending anddescending tracks. Our choices concerning the correction for localtopography, time-variable penetration effects and A–D biases were guided bythe principle of preferring the simplest viable model in order to keep the numberof parameters small compared to the number of observations.
In contrast to this single mission approach, here we perform a combinedprocessing of all data from different missions and even different altimetertechniques. Thus, some of the parameters may vary between the data sets. Toallow for offsets between the missions, the elevation at the cell centera0 is fitted for each mission individually. The same applies to dBS,which might relate to specific characteristics of a mission as well. ForSeasat, covering less than 100 days, this parameter is not estimated, as weassume that during the mission lifetime no significant changes occurred. ForICESat, dBS is not estimated either, as signal penetration is negligiblefor the laser measurements.
Between different observation techniques (i.e., PLRA, SARIn and laseraltimetry), the effective surface slope may also differ. Considering thespecific footprint sizes and shapes, the topography is sampled in acompletely different way as illustrated in Fig. 3. WhilePLRA refers to the closest location anywhere within the∼20 km beam-limited footprint (i.e., the POCA),CryoSat's SARIn measurement can be attributed within the narrow Dopplerstripe in the cross-track direction. For ICESat the∼70 mlaser spot allows a much better sampling of local depressions. Hence, theslope parametersa1 anda2 are estimated for each of the techniquesindependently.
Considering these sensor-specific differences, the model for the leastsquares adjustment in Eq. (1) is extended for multi-missionprocessing to
whereM(i) andT(i) denote to which mission or technique the measurementhi belongs.
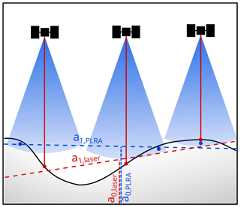
Figure 3Illustration of the technique-dependent topographic sampling. Thelaser (red) measures the surface elevation in the nadir of the instrument,while for radar altimetry (blue) the first return signal originates from thePOCA (marked by the blue point). Hence, planar surface approximations to themeasured heights (dashed lines) as in Eq. (2) are intrinsicallydifferent for the different techniques.
We define a priori weights for the measurementshi based on the precisionof the respective mission and mode from crossover analysis(Table 1) and depending on the surface slope at themeasurement location. This means that in regions with a more distinctivetopography, ICESat measurements (with a comparatively low slope-dependenterror component) will obtain stronger weights, compared to PLRA as Envisat.Over regions of flat topography, such as the interior of East Antarctica, theweights between PLRA and ICESat are comparable.
In order to remove outliers from the data and the results we apply differentoutlier filters. After the multi-mission fit, we screen the standardizedresiduals(Baarda, 1968) to exclude any resi that exceed 5 timesits a posteriori uncertainty. We iteratively repeat the parameter fit untilno more outliers are found. Furthermore, in order to exclude remainingunrealistic results from further processing, we filter our repeat-track cellsand reject any results where we obtain an absolute elevation change rate which is larger than 20 m yr−1 or where the standarddeviation of this rate is higher than 0.5 m yr−1.
3.2Single-mission time series
After fitting all parameters according to the multi-mission model(Eq. 2), we regain elevation time series by recombining theparametersa0 anddh∕dt with monthly averages of theresiduals (). For each monthj and each missionM, the time series are constructed as
This recombination of parameters from Eq. (2) and averages ofresiduals does not include theparameters of topography slope and backscatter regression. Hence, each timeseries ofhj,M relates to the cell center and is corrected fortime-variable penetration effects. Due to the reference elevationa0,M,which may also contain the inter-mission offset, the penetration depth and acomponent of the topography sampling within the cell, this results inindividual time series for each single mission. A schematic illustration ofthe results of this step is given in Fig. 5a. Thetemporal resolution of these time series is defined by using monthly averagesof the residuals. With typical repeat-cycle periods of 35 days or more, these typically represent the anomalies of a singlesatellite pass towards all parameters including the linear rate of elevationchange. The standard deviation of the residuals in these monthly averages isused as uncertainty measure forhj,M (see Sect. S3.1 for furtherdetails).
3.3Combination of the single-mission time series
In order to merge data from different missions into a joint time series,inter-mission offsets have to be determined and eliminated. In the ERSreprocessing project(Brockley et al., 2017), mean offsets between the ERSmissions and Envisat have been determined and applied to the elevation data.However, for ice sheet studies inter-satellite offsets are found to beregionally varying(Zwally et al., 2005;Thomas et al., 2008;Khvorostovsky, 2012). Whenmerging data from different observation techniques (PLRA, SARIn and laser)the calibration gets even more challenging. We chose an approach in differentsteps which is depicted in Figs. 4and5. The following section gives an overview andexplains the different steps to merge the single mission time series. Adetailed description of the parameters used in each step can be found in theSupplement.
3.3.1Merging PLRA time series
In the first step, we merge the PLRA time series. For these missions thetopographic sampling by the instruments is similar and thus the offsets arevalid over larger regions. For overlapping missions (ERS-1, ERS-2, Envisat,CryoSat-2 LRM) the offsets are calculated from simultaneous epochs (blue areain Fig. 5b), as performed byWingham et al. (1998)orPaolo et al. (2016). Smoothed grids of these offsets are generated, summedup if necessary to make all data sets comparable with Envisat (see Fig. S4)and applied to the respective missions. For the ERS missions, we findsignificant differences in the offsets for ice and ocean mode; hence, wedetermine separate offsets for each mode. Comparing our maps with similarmaps of offsets between ERS-2 (ice mode) and Envisat shown byFrappart et al. (2016) reveals that the spatial pattern agrees very well, butwe find significantly smaller amplitudes. We interpret this as a reducedinfluence of volume scattering due to our low retracking threshold. Inaccordance withZwally et al. (2005), we did not find an appropriatefunctional relationship between the offset and the waveform parameters.
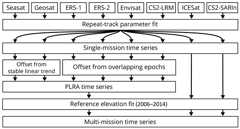
Figure 4Schematic diagram of the processing steps from the combinedrepeat-track parameter fit over single-mission time series towards a combinedmulti-mission time series.
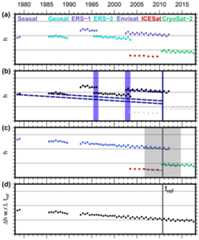
Figure 5Schematic illustration of the combination of the missions.(a) Single-mission time series of PLRA missions (blue and cyan),CryoSat-2 in SARIn mode (green) and the laser altimetry measurements ofICESat (red) with inter-mission offsets.(b) Offsets between thePLRA data are determined from overlapping epochs (blue area) ortrend-corrected elevation differences (according to Eq. 2) wheredh∕dt is sufficiently stable.(c) The specificoffset between PLRA, SARIn and laser data depends on the sampling of thetopography within each single cell. These different techniques are aligned byreducing each elevation time series by the specific elevation at thereference epochtref. Due to possible nonlinear surfaceelevation changes, this reference elevation is obtained from an 8-yearinterval only (gray area).(d) The combined multi-mission timeseries contains SECs with respect totref.
To calibrate Geosat and Seasat, a gap of several years without observationshas to be bridged. As depicted by the dashed blue lines inFig. 5b, we do this using the trend-correctedreference elevationsa0,M from the joint fit in Eq. (2).This, however, can only be done if the rate is sufficiently stable over thewhole period. Therefore, we use two criteria. First, we check the standarddeviation of the fit ofdh∕dt. Thisσdh∕dt indicates the consistency of theobservations towards a linear rate during the observational period. However,anomalies during the temporal gaps between the missions (i.e., 1978–1985 and1989–1992) cannot be detected in this way. Therefore, we further utilize afirn densification model(FDM; Ligtenberg et al., 2011;van Wessem et al., 2018). Thismodel describes the anomalies in elevation due to atmospheric processesagainst the long-term mean. The root mean square (rms) of the FDM time seriesis hence a good measure for the magnitude of the nonlinear variations insurface elevation. Consequently, only cells where cm yr−1 andrmsFDM<20 cm, indicating a highly linear rate, are usedto calibrate the two historic missions. Maps of the offsets with respect toEnvisat are shown in Fig. S5. The FDM criterion is not able to detect changesin ice dynamics. However, as regions where both stability criteria arefulfilled are mainly found on the plateau where flow velocity is below30 m yr−1(Rignot et al., 2017), we expect no significant nonlinearelevation changes due to ice flow. The mean of the offsets over all cellsamounts to−0.86 m for Seasat and−0.73 m for Geosat. Thecorresponding standard deviations of 0.85 and 0.61 m are mainly aresult of the regional pattern of the offsets. The true offsets are likely tohave spatial variations. However, we are not able to distinguish spatialvariations of the offset from residual effects of temporal height variationsin the regions meeting the stability criterion. In the regions not meetingthis criterion, we are not able to estimate the spatial variations of theoffset at all. Therefore, our final estimate of the offset, applied to themeasurements, is a constant, calculated as the average offset over theregions meeting the stability criterion. The spatial variability notaccounted for by the applied offset is included, instead, in the assesseduncertainty. Our bias between Seasat and Envisat ( m)agrees within uncertainties with the ocean-based bias of−0.77 mused byFricker and Padman (2012). However, we prefer the offset determined overthe ice sheet because this kind of offset may depend on the reflecting medium(see Sect. S3.2.2 for a more detailed discussion).
With the help of these offsets, all PLRA missions were corrected towards thechosen reference mission Envisat. Uncertainty estimates of the offsets areapplied to the respective time series to account for the additionaluncertainty. Hence, the PLRA time series are combined (blue inFig. 5c with additional CryoSat-2 LRM mode whereavailable). At epochs when more than one data set exists, we apply weightedaveraging using the uncertainty estimates.
3.3.2Technique-specific surface elevation changes
In contrast to the PLRA data in the previous step, when merging data fromdifferent observation techniques such as CryoSat's SARIn mode, ICESat's laserobservations and PLRA, the different sampling of topography also has to beconsidered. As noted in Sect. 3.1, this might lead tocompletely different surfaces fitted to each type of elevation measurementand the time series need to be calibrated for each cell individually.However, not all cells have valid observations of each data set. Therefore,instead of calibrating the techniques against each other, we reduce each timeseries by their elevation at a common reference epoch and hence obtain timeseries of surface elevation changes (SEC) with respect to this reference epoch insteadof absolute elevation time series. This step eliminates offsets due todifferences in firn penetration or due to the system calibration between thetechniques as well.
We chose September 2010 (09/2010) as thereference epoch. This epoch is covered by the observational periods of PLRAand CryoSat SARIn and also is exactly 1 year after the last observations ofICESat, which reduces the influence of an annual cycle. As discussed inSect. 3.3.1, nonlinear elevation changes will adulteratea0 from Eq. (2), obtained over the full time span. Therefore,we applied another linear fit to a limited time interval of 8 years only(September 2006–September 2014, gray area in Fig. 5c). Wesubtract the variation of the FDM over this period to account for short-termvariations. The limited time interval reduces the influence of changes in icedynamics. We estimate the individual reference elevationsa0,T for eachtechniqueT and a jointdh∕dt. After subtracting thetechnique-specific reference elevationsa0,T from the respective timeseries, they all refer to 09/2010 and can be combined.
3.3.3Merging different techniques
We perform the final combination of the techniques using a weightedspatiotemporal averaging with 10 kmσ Gaussian weights inthe spatial domain (up to a radius of3σ=30 km) and over 3 epochs(i.e., including the two consecutive epochs) in the temporal domain. Hence, weobtain grids of surface elevation change (SEC) with respect to 09/2010 foreach month observed. Due to the smoothing of the weighting function, wereduce our spatial SEC grid resolution to 10 km × 10 km. The respectiveuncertainties are calculated according to the error propagation. To avoidextrapolation and to limit this merging step to the observed area only, wecalculate a value for an epoch in the 10 km × 10 km grid cells only ifwe have data within 20 km around the cell center (which is about thesize of a beam-limited radar footprint). The five examples inFig. 6 demonstrate the spatiotemporal coverage of the resultingSEC grids at different epochs. The corresponding uncertainty estimates, givenin Fig. 6b (further details in the Supplement), reach values of1 m and more. Besides the measurement noise and the uncertainties ofthe offsets, these uncertainty estimates contain a further component from theweighted averaging. In regions with large variations inΔh overrelatively short spatial scales (such as at fast-flowing outlet glaciers),such variations can add a significant contribution toσΔh. Asthe magnitude ofΔh grows with the temporal distance to the referenceepoch, the largest contributions toσΔh can be expected forthe earliest epochs. This also explains why the epoch 09/2008 provides thelowest uncertainty estimate in these examples, even lower than the CryoSat-2-based epoch 09/2017.
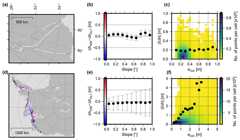
Figure 7Validation with elevation differences observed by kinematic GNSSbetween 2001 and 2015 (a, b, c) and Operation IceBridge between 2002and 2016 (d, e, f). Differences between elevation changes observedby the validation data and altimetry are shown in the maps (a, d,color scale in panels b ande). Median and MAD of thesedifferences, binned by different surface slope, are shown in thecenter (b, e). The right diagrams (c, f) show thecomparison of these differences with the respective uncertainty estimate,obtained from both data sets. The point density is plotted from yellow toblue and the black dots show the root mean square, binned against theestimated uncertainty.
4.1In situ and airborne observations
To validate our results, we used inter-profile crossover differences of19 kinematic GNSS profiles(available for downloadfrom Schröder et al., 2016) and elevation differences from Operation IceBridge(OIB ATM L4; Studinger, 2014). The ground-based GNSS profiles wereobserved between 2001 and 2015 on traverse vehicles of the Russian AntarcticExpedition and most of them covered more than 1000 km. The accuracyof these profiles has been determined inSchröder et al. (2017) to4–9 cm. One profile (K08C) has not been used due to poorlydetermined antenna height offsets. For each crossover difference betweenkinematic profiles from different years, we compare the differences of thecorresponding altimetric SEC epochs in this location (). The same analysis has beenperformed with the elevation changes obtained from differences ofmeasurements of the scanning laser altimeter (Airborne Topographic Mapper,ATM) of OIB. As described byStudinger (2014), the Level 4Δhproduct is obtained by comparing planes fitted to the laser scanner pointclouds. The flights, carried out between 2002 and 2016, were stronglyconcentrated along the outlet glaciers of West Antarctica and the AntarcticPeninsula. Hence, they cover much more rugged terrain, which is morechallenging for satellite altimetry. Over the tributaries of the Amundsen Seaglaciers and along the polar gap of ICESat, some repeated measurements havealso been performed over flat terrain. The accuracy of these airbornemeasurements has been validated, e.g., near summit station in Greenland.Brunt et al. (2017) used ground-based GNSS profiles of snowmobiles for thistask and obtained offsets on the order of only a few centimeters and standarddeviations between 4 and 9 cm.
Figure 7a and d show the results of our validation (moredetailed maps for several regions at Fig. S6). A satellite calibration errorwould lead to systematic biases between the observed elevation differences ifΔhALT is obtained from data of two different missions.However, such biases may also be caused by systematic errors in thevalidation data. Furthermore, in contrast to the calibration data, the RAmeasurements may systematically miss some of the most rapid changes if thoseare located in local depressions(Thomas et al., 2008). With an overall mediandifference of6±10 cm for the GNSS profiles and cm for OIB, however, the observed elevation changes show onlymoderate systematic effects and agree within their error bars. The medianabsolute deviation (MAD) for different specific surface slopes(Fig. 7b and e) reveals the influence of topography inthis validation. The GNSS profiles show only a very small increase of thisvariation with slope. The IceBridge data cover the margins of many WestAntarctic glaciers, where elevation changes differ over relatively shortdistances. Hence, it is not surprising that we see a significantly largerspread ofδΔh at higher slopes here. However, in the lessinclined regions, the MAD of the differences is still at a level of 25 cm,which is significantly larger than in the comparison with the GNSS profiles.This large spread for regions with low slopes originates mainly from thetributaries of Pine Island Glacier, where many campaigns of OIB are focused(see Figs. 7d and S6d for details). While still relativelyflat, the surface elevation in this area is comparatively low, which leads toa stronger influence of precipitation(Fyke et al., 2017). This induces highershort-term variations in surface elevation, which might explain the higherdifferences between the IceBridge results and our 3-month temporally smoothedaltimetry data. In contrast, the differences around the South Pole or inQueen Elizabeth Land (see also Fig. S6c) are significantly smaller. For the2016 campaign of OIB,Brunt et al. (2018) furthermore report a spuriouselevation variation of 10 to 15 cm across the wide-scan ATM swath, whichindicates a bias in the instrumental tilt angle. This could explain thesystematic differences along the 88∘ S circle profiles where thiscampaign is involved.
The observedδΔh can further be used to evaluate theuncertainty estimates. In Fig. 7c and f, the uncertaintyestimates of the four contributing data sets are combined and compared to theobserved differences. The comparison with both validation data sets supportsthe conclusion that the uncertainty estimates are reasonable. ForΔhALT we expecthigher errors in coastal regions due to the increased uncertainty of thetopographic correction in radar altimetry. A similar relation to topographyis expected forΔhOIB due to the plane fit to the ATM pointcloud,but surface roughness and crevassing also play an important role here. Incontrast, the errors of the GNSS-derivedΔhKIN are almostindependent of topography. Instead,ΔhKIN tends to be moreuncertain on the plateau, where the soft snow causes large variations of thesubsidence of the vehicles into the upper firn layers. The relatively lowdifferences inδΔh, even in regions that imply a higheruncertainty, are likely just incidental for the small sample of validationdata along the GNSS profiles.
In conclusion, this validation shows that remaining systematic biases(originating from satellite altimetry or the validation data) are less than adecimeter in the observed regions and that our uncertainty estimate isrealistic. However, only altimetric SEC within the interval 2001–2016 can bevalidated in this way. For the earlier missions, no spatially extensive high-precision in situ data are publicly available.
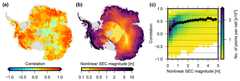
Figure 8(a) Correlation coefficient between the SEC anomalies ofthe altimetry grids and the FDM over 1992–2016 after detrending.(b) Average magnitude of anomalies of the altimetry time series.(c) Correlation coefficient plotted against the nonlinear SEC. Thepoint density is color coded from yellow to blue. The black dots show thebinned mean values.
4.2Firn model
Another data set, which covers almost the identical spatial and temporalrange as the altimetric data, is the IMAU Firn Densification Model(FDM; Ligtenberg et al., 2011), forced at the upper boundary by the accumulationand temperature of the Regional Atmospheric Climate Model, version 2.3p2(van Wessem et al., 2018). The IMAU-FDM has been updated to the period1979–2016, modeling the firn properties and the related surface elevationchanges on a 27 km grid. However, as the FDM contains elevationanomalies only, any long-term elevation trend over 1979–2016, e.g., due tochanges in precipitation on longer timescales(for example those observed insome regions of West Antarctica; Thomas et al., 2015) would not be included in themodel. Furthermore, due to the nature of the model, it cannot giveinformation about ice dynamic thinning and thickening. Hence, to compare the FDMand the SEC from altimetry, we first remove a linear trend from both datasets. This is performed for the period 1992–2016 (depicted inFig. S7). The trends are only calculated from epochs where both data setshave data; i.e., in the polar gap this comparison is limited to 2003–2016 or2010–2016, depending on the first altimetry mission providing data here.After the detrending, the anomalies are used to calculate correlationcoefficients for each cell, depicted in Fig. 8a.Figure 8b shows the average magnitude of the seasonal andinterannual variations (nonlinear SEC), calculated as the rms of theanomalies from the altimetry data. Comparing the two maps shows that thecorrelation is around 0.5 or higher, except in regions where the magnitude ofthe anomalies is small (i.e., where the signal-to-noise ratio of thealtimetric data is low) and where large accelerations in ice velocity areobserved (such as near the grounding zone of Pine Island Glacier). Therelationship between the correlation coefficient and the magnitude of thenonlinear SEC is depicted in Fig. 8c, where we see thatfor the vast majority of cells the correlation is positive. For anomalieswith a nonlinear SEC>0.5 m, the average correlation is between 0.3and 0.6.
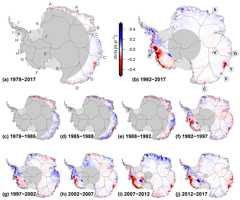
Figure 9Multi-mission surface elevation change from the combined SEC timeseries over different time intervals.(a, b) The long-term surfaceelevation change between 1978 and 2017 and 1992 and 2017 for thecovered area.(c–j) Elevation change over consecutive timeintervals reveals the interannual variability. Thin lines mark the drainagebasin outlines, denoted in panel (a). Bold letters in boxes inpanel (b) denote areas mentioned in the text and inFig. 10.
Anomalies against the simultaneously observed long-term trend (1992–2016) canalso be computed for earlier epochs. Assuming no significant changes in icedynamics here, these anomalies allow for a comparison of Geosat and Seasat withthe FDM. The median difference between the anomalies according to Geosat andthe anomalies according to the FDM amounts to0.12±0.21 m (seeFig. S8). Considering that this difference is very sensitive to extrapolatingthe long-term trends, this is a remarkable agreement. With a median of0.26±0.32 m, the difference between anomalies from Seasat andfrom the FDM is larger, but this comparison is also more vulnerable topotential errors due to the extrapolation. As the FDM starts in 1979 whileSeasat operated in 1978, we compare the Seasat data with the FDM anomaliesfrom the respective months of 1979, which might impose additionaldifferences. Finally, the FDM model has its own inherent errors anduncertainties. Therefore, only part of the differences originate from errorsin the altimetry results.
5.1Surface elevation changes
The average rates of elevation change over different time intervals of ourmulti-mission time series are shown in Fig. 9. To calculatethese rates, we first averaged the data over the first year and the last yearof the interval to reduce the noise, then subtracted the respective averagesfrom each other and finally divided these differences by their timedifference in years. If one of the years does not cover the full annualcycle, we calculate the average only from the months covered in both years(July–October for 1978–2017, April–December for 1992–2017). We calculatethe SEC rate from epoch differences instead of fitting a rate to all epochsbecause the first observations at specific latitudes start in differentyears, the observations havedifferent precisions and the large gap between 1978 and 1985 is not coveredby observations at all. These three points would lead to a bias towards thelater epochs in a fit, so that the rates would not be representative for thetrue average elevation change over the full interval.
The long-term elevation changes over 25 years (Fig. 9b) show thewell known thinning in the Amundsen Sea Embayment and at Totten Glacier, aswell as the thickening of Kamb Ice Stream (see, e.g.,Wingham et al., 2006b;Flament and Rémy, 2012;Helm et al., 2014). In contrast, 60 % of East Antarctica north of81.5∘ S shows surface elevation changes of less than±1 cm yr−1. Several coastal regions of the EAIS, however, showsignificant elevation changes. Totten Glacier (T in Fig. 9b) isthinning at an average rate of72±18 cm yr−1 at the groundingline (cf. Fig. 10b). Several smaller glaciers in Wilkes Landalso show a persistent thinning. We observe SEC rates of cm yr−1 at Denman Glacier (D), cm yr−1 at FrostGlacier (F) and cm yr−1 near Cook Ice Shelf (C).Rignot (2006) showed that the flow velocity of these glaciers, whichare grounded well below sea level, was above the balance velocity for manyyears.Miles et al. (2018) analyzed satellite images since 1973 and found thatthe flow velocity of Cook Ice Shelf glaciers has significantly accelerated since then. In contrast, thewestern sector of the EAIS (Coats Land, Dronning Maud Land and Enderby Land;basins J”–B) shows thickening over the last 25 years at rates of up to adecimeter per year.
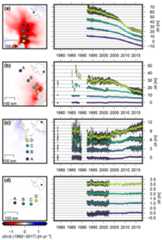
Figure 10Multi-mission SEC time series in four selected regions(a) Pine Island Glacier,(b) Totten Glacier,(c) Shirase Glacier in Dronning Maud Land and(d) LakeVostok (marked by P, T, S and V in Fig. 9b). The time series ofpoints B, C and D are shifted alongΔh for better visibility and theoneσ uncertainty range is displayed in black. The maps on the left showthe elevation change rate between 1992 and 2017 as in Fig. 9b(but in a different color scale).
Comparing the long-term elevation changes over 40 years(Fig. 9a) with those over 25 years shows the limitations of theearly observations, but also the additional information they provide. Therewere relatively few successful observations at the margins. However, forTotten and Denman glaciers, the 40-year rates at a distance of approximately100 km inland from the grounding line are similar to the rates overthe 1992–2017 interval, which indicates a persistent rate of thinning.Another benefit of our merged time series is that they allow for thecalculation of rates over any subinterval, independent of mission periods as demonstratedin Fig. 9c–j. For most of the coastal regions of the AIS,these rates over different intervals reveal that there is significantinterannual variation. Such large-scale fluctuations in elevation change havebeen previously reported byHorwath et al. (2012) andMémin et al. (2015) forthe Envisat period. Our combined multi-mission time series now allow adetailed analysis of such signals on a temporal scale of up to 40 years.
Four examples for elevation change time series in the resulting multi-missionSEC grids are shown in Fig. 10 (coordinates in Table S2). PineIsland Glacier (PIG) is located in the Amundsen Sea Embayment, which isresponsible for the largest mass losses of the Antarctic Ice Sheet(e.g., McMillan et al., 2014). In East Antarctica, the largest thinning ratesare observed at Totten Glacier. The region of Dronning Maud Land and EnderbyLand in East Antarctica has been chosen as an example for interannualvariation. Here,Boening et al. (2012) reported two extreme accumulationevents in 2009 and 2011, which led to significant mass anomalies. We chose aprofile at Shirase Glacier as an example for this region. In contrast to theprevious locations, a very stable surface elevation has been reported forLake Vostok(e.g., Richter et al., 2014). This stability, however, has been acontroversial case recently(Zwally et al., 2015;Scambos and Shuman, 2016;Richter et al., 2016).Therefore, our results in this region shall add further evidence thatpinpointsthe changes there.
For Pine Island Glacier (Fig. 10a), we observe a continuousthinning over the whole observational period since 1992 (Seasat and Geosatmeasurements did not cover this region). Close to the grounding line (point D) the surface elevation has decreased by m since 1992,which means an average SEC rate of m yr−1. The timeseries reveals that this thinning was not constant over time, but acceleratedsignificantly around 2006. The mean rate at D over 1992–2006 of m yr−1 increased to m yr−1 over2007–2010. After 2010, the thinning rates near the grounding line decelerateagain and for the period 2013–2017, the rate at D of m yr−1 is very close to the rate preceding theacceleration. Also, at greater distances from the grounding line (B at80 km, A at 130 km) we observe an acceleration of theprevailing rates around 2006 ( m yr−1 over 1992–2006, m yr−1 over 2006–2017 at A). In contrast to the pointsnear the grounding line, further inland the thinning has not decelerated sofar and is still at a high level. Hence, for the most recent period(2013–2017) the elevation at all points along the 130 km of the mainflow line is decreasing at very similar rates. A similar acceleration of theelevation change rate near the grounding line, followed by slowdown, isobserved byKonrad et al. (2016). The onset of this acceleration coincideswith the detaching of the ice shelf from a pinning point(Rignot et al., 2014).For the time after 2009,Joughin et al. (2016) report relatively littlegrounding line migration, resulting in a leveling off of the ice flowvelocity. This agrees with our observed slowdown of elevation changes.
For Totten Glacier in East Antarctica (Fig. 10b), weobserve a clear negative SEC. This has been previously reported by severalauthors(e.g., Pritchard et al., 2009;Flament and Rémy, 2012;Zwally et al., 2015), but our dataprovide an unprecedented time span and temporal resolution, allowing for theanalysis of the evolution of the elevation changes on a monthly scale over up to40 years. At the grounding line (point D), Totten Glacier thinned by31.8±7.7 m between 1987 and 2017, which results in an averageSEC rate of m yr−1. Seasat could not providesuccessful observations at the grounding line, but the time series forpoint C (around 60 km inland) with a rate of m yr−1 between 1978 and 2017 and for point B(150 km) with a rate of m yr−1 indicate thatthis thinning preceded the epoch of Geosat. At point A in adistance of 280 km, we find no significant elevation change(0.01±0.03 m yr−1 for 1978–2017). The temporal resolution ofthese data allows us to analyze the change over time. While we see asignificant thinning at the grounding line between 1987 and 1994 of16.6±9.8 m, the elevation stabilized between 1994 and 2004 towithin±1.5 m. After 2004, the ice at the grounding linethinned again by15.4±5.5 m until 2017.Li et al. (2016) observea similar variation in ice velocity measurements between 1989 and 2015.Combining their ice discharge estimates with surface mass balance, theyobtain a relatively large mass imbalance for Totten Glacier in 1989,decreasing in the following years to a state close to equilibrium around2000. After 2000, they observe an acceleration of ice flow, again consistentwith our thinning rates. The authors attribute this high variability tovariations in ocean temperature. In another study,Li et al. (2015) observe agrounding line retreat at Totten Glacier of 1 to 3 km between 1996and 2013 using SAR interferometry. They conclude that this indicates athinning by 12 m, which is again consistent with our results overthis period (12.0±8.8 m).
At Shirase Glacier in Dronning Maud Land (DML, Fig. 10c), weobserve a relatively stable surface with a slightly negative change ratebetween 1978 and the early 2000s. The subintervals until 2002 in theelevation change maps of Fig. 9c–g confirm that this agreeswith the large-scale trend in this region. After 2002, however, the rate changed significantly. Ourtime series show an increasing surface elevation, which is most pronouncedduring the time of the two significant accumulation events in 2009 and 2011in this region(Boening et al., 2012;Lenaerts et al., 2013). At point C, the elevationchanged by1.0±1.5 m between 2008 and 2012. Even at point A,more than 200 km inland and at an altitude of 2500 m, theelevation increased by0.55±0.50 m during this time. At point D,an abrupt elevation increase is also observed in 2003, which corresponds toanother SMB anomaly(cf. Fig. 2a in Lenaerts et al., 2013). The map inFig. 9h shows that the coastal regions of Enderby Land (basinsA'–B) already experienced elevation gains before 2007. In contrast to the2009 and 2011 events, which affected a very large region(Fig. 9i), this earlier accumulation event is significantly morelocalized at the coast.
In contrast to the regions discussed so far, the elevation change on theplateau of East Antarctica is very small. The time series for four differentpoints at Lake Vostok (Fig. 10d) show rates withinuncertainties and very close to zero (point A:5±9 mm yr−1, B: mm yr−1, C: mm yr−1, D: mm yr−1 between 1992 and 2017). The larger variations inthe ERS time series are a result of the lower resolution of the waveform inthe ice mode of the ERS satellites. These rates contradict the findings ofZwally et al. (2015). They report a surface elevation increase of20 mm yr−1 over Lake Vostok, which would result in an elevationincrease of 0.5 m over the period 1992–2017. Our results areconfirmed by ground-based static GNSS observations(Richter et al., 2008, 0.3±4.9 mm yr−1), kinematic GNSS profilesmeasured around Vostok Station using snow mobiles(Richter et al., 2014, 1±5 mm yr−1) and by GNSS profiles usingtraverse vehicles over the entire Lake Vostok region(Schröder et al., 2017, mm yr−1).
5.2Ice sheet mass time series
The surface elevation time series are converted into ice mass changes inorder to determine their effect on global sea level. In the first step, theSECs are corrected for uplift rates related to glacial isostatic adjustment(GIA) using coefficients from the IJ05_R2 model(Ivins et al., 2013). This GIAmodel predicts an uplift of 5 mm yr−1 near the Antarctic Peninsula andrates between−0.5 and+2 mm yr−1 in East Antarctica. Furthermore, wemultiplied the SEC by a scaling factorα=1.0205 to account forelastic solid earth rebound effects(Groh et al., 2012). The resulting icesheet thickness changes are multiplied by each cell's area and a densityaccording to a firn and ice mask(McMillan et al., 2014,2016), depicted inFig. S10, to obtain a mass change. In regions where ice dynamic processes areassumed to be dominating (e.g., in the Amundsen Sea Embayment, Kamb Ice Stream orTotten Glacier), we use a density of 917 kg m−3.Elsewhere, we apply the density of near-surface firn as modeled byLigtenberg et al. (2011), using annual averages of accumulation, 10 mwind speed and surface temperature. We have chosen this straightforwardmethod here, instead of using the modeled impact of the temporal variationsof accumulation, melting and firn compaction on the firn layer(see,e.g., Zwally et al., 2015;Kallenberg et al., 2017) in the volume-to-mass conversion. Thisallows us to keep our altimetry time series independent of the modeledvariations in SMB, which is a prerequisite for the interpretation of thecomparison of both data sets.
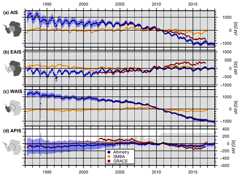
Figure 11Mass change of the Antarctic Ice Sheet north of81.5∘ S (a) and the three subregions (b: EAIS,c: WAIS andd: APIS) from our combined altimetric timeseries (blue), GRACE (red) and SMBA (orange). The error bars show theuncertainty estimateσΣ of the altimetry data according toSect. S6.2. The gray color in the background displays the fraction of thearea covered by altimetry (up to the top of the panel means 100 %).
We integrate our measurements over larger regions to calculate the cumulativemass anomalies for individual drainage basins and major Antarctic sectors(AIS; WAIS; EAIS; APIS). Our basin delineations are fromRignot et al. (2011),which have been updated for the second Ice Sheet Mass BalanceInter-comparison Exercise(IMBIE-2; Shepherd et al., 2018). Cells that weremasked out due to the predominance of rocks or that are considered unobservedafter our gridding (due to the polar gap or a lack of valid observations) arenot included in these sums. Uncertainty estimates are obtained by propagatingthe uncertainties of the SEC, the GIA and the firn density to the basin sumsfor each month (see Sect. S6.2 for details). Toaccount for the lack of information due to unobserved cells, we also add atotal estimate for the effect of these cells, based on trends from GRACE, tothe error budget.
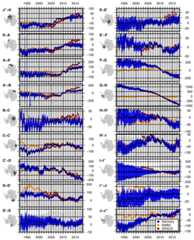
Figure 12Mass change (ΔM [Gt]) of the individual drainage basinsnorth of 81.5∘ S from our combined altimetric time series (blue),GRACE (red) and SMBA (orange). The error bars show the uncertainty estimateσΣ of the altimetry data according to Sect. S6.2. The gray colorin the background displays the fraction of the area covered by altimetry (thetop of each panel means 100 %).
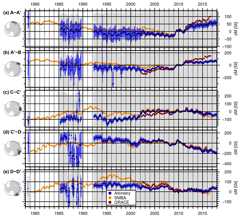
Figure 13Mass change of subregions north of 72∘ S for several EastAntarctic drainage basins from our combined altimetric time series (blue),GRACE (red) and SMBA (orange). The error bars show the uncertainty estimateσΣ of the altimetry data according to Sect. S6.2. The gray colorin the background displays the fraction of the area covered by altimetry (thetop of each panel means 100 %).
Figure 11a–d show time series for the entire AIS northof 81.5∘ S (i.e., covered by satellite altimetry since 1992), andthe subregions EAIS, WAIS and the APIS. Similar time series for the singledrainage basins over 1992–2017 are shown in Fig. 12. Forthe coastal areas of the EAIS the full time interval since 1978 is shown inFig. 13. These 4-decade time series use data north of72∘ S only and, hence, provide a nearly consistent observationalcoverage over the whole period. To support the interpretation and evaluatethe temporal evolution, we compared the respective time series toGIA-corrected cumulated mass anomalies from satellite gravimetry(GRACE; Groh and Horwath, 2016), which are products of the ESA Climate ChangeInitiative (CCI) Antarctic Ice Sheet project and are available for downloadathttps://data1.geo.tu-dresden.de/ais_gmb (last access: 1 February2019) andhttp://cci.esa.int/data (last access: 1 February 2019). Toreduce the effect of noise in the GRACE monthly solutions and to make thedata more comparable to our altimetry results, we applied a 3-month movingaverage to the GRACE time series. We also compare our data to time series ofcumulated surface mass balance anomaly (SMBA) from RACMO2.3p2(van Wessem et al., 2018). To obtain these anomalies, the gridded SMB rates havebeen reduced by a mean rate and integrated over time. Similar to the IMAUfirn model, these SMBAs contain seasonal and interannual variations due tosurface processes but do not include long-term changes over the full modeledperiod (1979–2016). The different time series show the good agreement of thetechniques in resolving interannual variations. For example for the basin ofTotten Glacier (C'–D in Fig. 12), all techniques observe anegative mass anomaly in early 2008, followed by a significant mass gain in2009 as previously reported byVelicogna et al. (2014) andLi et al. (2016).Between the epochs 03/2008 and 10/2009, we obtain a mass difference of116.6±27.0 Gt from altimetry, 109.4 Gt from SMBA and113.4 Gt from GRACE. The high agreement with SMBA indicates that thismass gain at Totten Glacier is caused by snow accumulation. In most of thebasins, we observe a similar high agreement in the short-term variations. Agood example for a total mass change signal which is constituted fromcomponents of SMBA and ice dynamics is the Getz and Abbot region (F–G) inWest Antarctica. While all techniques observe a significant mass loss between2009 and 2011, the SMBA does not contain the decadal trend, as observed byaltimetry and GRACE. In some regions, there are also significantdiscrepancies between the data sets of satellite altimetry and GRACE.Inadequate sampling by radar altimetry (such as in the northern tip of theAntarctic Peninsula (I–I”) where steep regional topography and small outletglacier size limits the recovery), leakage in the GRACE estimate betweendifferent sectors and uncertainties in the individual measurements and in thegeophysical corrections might cause these differences. In George V Land(D–D'), the agreement during the GRACE period is reasonable, while the massgain, indicated by SMBA in the early 1990s, is not revealed by the altimetrytime series.
Over the last 25 years our data indicate a clearly negative mass balance of Gt for the AIS (Fig. 11a), whichcorresponds to an increase in mean sea level of5.7±1.0 mm. Thischange is mainly a result of the mass loss in the WAIS over the last decade.In contrast, the EAIS has been very stable over our observational record(120±121 Gt between 1992 and 2017). The time series of the APIScontains large uncertainties due to many unobserved cells. Mass change ratesfor selected regions, obtained from the differences over a specific timeinterval, and their uncertainties are given in Table 2. Wecalculated separate trends for the area north of 72∘ S, which iscovered by all satellites, the area north of 81.5∘ S, which has beencovered since the launch of ERS-1,and for the total area, which has been covered since the launch of CryoSat-2,except for its 500 km polar gap. A total of 96.4 % of the cellsclassified as ice sheet north of 81.5∘ S are successfully covered byobservations of ERS-1. Cells without successful observation occur mostly atthe APIS, where only 61 % is covered with data.
From the overall mass loss of Gt for the AIS(<81.5∘ S over 1992–2017) we obtain an average long-term rate of Gt yr−1 (or a corresponding mean sea level changerate of0.24±0.04 mm yr−1). After 2010, this rate accelerated to Gt yr−1 or0.38±0.07 mm yr−1 of mean sealevel.
Table 2Mass change rates for different regions of the Antarctic Ice Sheetand different time intervals. The sizes of the total and observed area referto all cells classified as ice sheet in the respective region (and ifstated are limited by the given latitude).
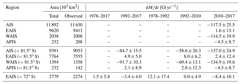
For the APIS (<72∘ S), the very sparse observations ofSeasat and Geosat did not allow for the calculation of a reliable trend.
6.1Surface elevation changes
Combining all the single missions consistently, our SEC time series allow for theanalysis of the long-term changes over the full time period of satellitealtimetry observations. For 79 % of the area of the AIS, this means atime span of 25 years. Over 25 % of the ice sheet, largely in the coastalregions of East Antarctica, the time series can be extended back 40 years.Such long-term trends are significantly less affected by short-termvariations in snowfall than a trend from a single mission. Furthermore, theperiod of observation of a single mission is short compared to climaticoscillations as reported by, e.g.,Mémin et al. (2015). Our extended time serieshelps to separate elevation change due to climate variations from potentiallyaccelerating volume losses. Seasat and Geosat also provide importantinformation here, despite their larger uncertainties. Due to the stabilitycriteria in the calibration, we do not expect significant new insights on theEast Antarctic Plateau (even as regional variation still may be discernibleas we used an ice-sheet-wide average in calibration). However, in the coastalregions of East Antarctica, with SECs of up to several meters with respect to 2010 (seeFig. 6), the older data can also contribute significantinformation to the study of elevation changes in a long-term context of 40 years(cf. the rates in Fig. 9 and their uncertainties in Fig. S9).Unfortunately, in coastal DML west of the ice divide A', the data of Seasatand Geosat are very noisy due to the mountain ranges just north of72∘ S in these regions. They lead to many signal losses across this part of the ice sheet. The same applies to the measurementsat the APIS.
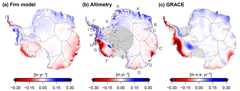
Figure 14Mean rates for the time interval 2002–2016 of elevation changesfrom IMAU-FDM (a), from the multi-mission SEC grids (b) andof the mass changes from GRACE (c).
The benefits of a seamless combination of the time series are demonstrated inFig. 9. The time intervals for the elevation changes areindependent of the observational period of a single mission. This isnecessary to analyze processes which occurred close to the transition betweendifferent missions. A good example of the advantage of such long time seriesis the elevation changes caused by the accumulation events in DML.Figure 10c clearly shows the changes in elevation, caused bythe strong snowfall events in 2009 and 2011. The mission lifetime of ICESatended during epoch 10/2009, CryoSat-2 provided the first measurements in epoch 07/2010. OnlyEnvisat covered both events, but here the orbit was shifted in epoch 10/2010,resulting in different repeat-track cells covered before and after the orbitshift. We merged all these missions as described inSect. 3.3, which allows us to analyze the full time series.Comparing the elevation changes from altimetry with those in the FDM servesas a cross-validation of both data sets. For example, at point A inFig.10c our SEC time series observes a change of0.55±0.50 m between 2008 and 2012, while the FDM models a verysimilar elevation gain of 0.48 m for this period.Figure 8 shows the degree of agreement over the entire AIS.
As these elevation change rates alone do not contain any information on theirorigin, additional data are needed for improved process understanding.Figure 14 shows SEC rates for the interval 2002–2016(calculated as in Sect. 5.1 over March–September, respectively) from altimetry and the IMAU-FDM and corresponding rates of icemass changes from GRACE. These maps show that the elevation gains in DML andEnderby Land agree very well with the firn model, which implies thatincreased snow accumulation during this period is responsible for thethickening. For Princess Elizabeth Land (C–C'), the negative rates agree aswell, implying that the thinning here can be related to lower than normalsnow accumulation. In contrast, the strong thinning along the Amundsen SeaEmbayment (G–H) or the thickening of Kamb Ice Stream (E'–F) is not presentin the FDM results but does show up in the GRACE data. Due to the higherdensities of the involved material, ice dynamic processes are even morepronounced in the map of mass changes compared to the maps of elevationchanges.
The inland propagation of dynamic thinning of the glaciers of the AmundsenSea Embayment over the last few decades has been described byKonrad et al. (2016). A recent onset of significant mass losses has also beenreported for the adjacent glaciers along the Bellingshausen Sea(H–I; Wouters et al., 2015) and on the Getz and Abbot region(F–G; Chuter et al., 2017). Figure 9i reveals that the largestlosses along the coast of the WAIS occurred between 2007 and 2012. The period2012–2017 (Fig. 9i) shows that only a part of these large ratesis persistent. While the ice discharge of the Getz and Abbot region increased by 6 % between2008 and 2015(Gardner et al., 2018), the deceleration of the elevation changeafter 2012 indicates that interannual variations in SMB also have to beconsidered here(see also Chuter et al., 2017). The FDM-derived rate inFig. 14a confirms the role of the surface massbalance in this region.
6.2Ice sheet mass time series
The integrated time series of basin-wide mass changes allow for the analysisof the temporal evolution at a monthly resolution(Fig. 12). As described in the previous section, for mostof the basins of the WAIS, they show an increase of mass loss after themid-2000s. The acceleration of thinning at the Getz and Abbot region (F–G) started already in2004, but experienced a further significant acceleration after 2007. In theAmundsen Sea Embayment, a small positive mass anomaly in late 2005 relates toa similar event in the SMBA time series, but after that, also here theoverall mass losses accelerated. The Bellingshausen Sea basin (H–H') wasrelatively stable until 2009, but started to lose significant amounts of massafter that time, as reported byWouters et al. (2015). Since 2016, however, weobserve that the basins at the Bellingshausen Sea and the western part of thepeninsula regained mass. The comparison with SMBA reveals that this can beexplained by a positive snowfall anomaly in this area in 2016. The shape andorientation of the peninsula makes GRACE observations challenging withrespect to leakage and GRACE error effects(Horwath and Dietrich, 2009).Nevertheless, the results of the satellite gravity mission confirm this massanomaly.
A similar comparison of the ice-sheet-wide mass time series between altimetryand GRACE in Fig. 11 reveals that for the entire WAIS,both data sets agree very well, while for the APIS and the EAIS, we observesignificant differences on a decadal scale of the trends. The percentage ofobserved area of the APIS (gray area in the background ofFig. 11d) indicates that before 2010, a significant partof the area remained unobserved. Here, conventional RA measurements veryoften failed due to the rugged terrain. Even for ICESat, the largeacross-track distances and the dependence on cloud-free conditions makemeasurements very sparse at the peninsula. With the weather independent,dense and small footprint measurements of CryoSat-2 in SARIn mode, up to80 % of the area is covered by observations. Compared to GRACE, however,we observe a significantly weaker mass loss signal.Thomas et al. (2008)pointed out that RA fails to sample the large elevation changes in narrowvalleys of outlet glaciers. This leads to an overall underestimation of the signal byaltimetric observations. Furthermore, the complex terrain, especially in theAPIS, also causes problems in the parameter fit. Even if enough validmeasurements are available (as from, for example, ICESat or CryoSat-2), thefit of a planar surface over a diameter of 2 km in ourrepeat-altimetry processing can hardly adequately represent the realtopography here. Our approach is designed to provide valid results over themajority of the AIS. Under the challenging conditions of the APIS,modifications such as a smaller diameter or more complex parameterization ofthe surface would surely help to improve the results. Furthermore, we did notcalculate a SEC for cells that are further away than a beam-limited radarfootprint from valid measurements. In order to interpolate or evenextrapolate the results to unobserved cells, advanced gridding methods suchas kriging, especially with the help of additional data sets(Hurkmans et al., 2012), would be advisable.
This effect may also explain the differences of our results, compared to theresults of the combination of different techniques byShepherd et al. (2018).Their 1992–2017 rate of Gt yr−1 agrees within error barswith our results, but our rate of Gt yr−1 isconsiderably smaller. Part of this disagreement might be attributed todifferences in the estimates for the Antarctic Peninsula where retrievingreliable radar altimetry estimates is nontrivial. However, the extendedmaterial inShepherd et al. (2018) shows that there are still somediscrepancies between the different techniques to determine the AIS massbalance. For the time interval 2003–2010(Extended Data Table 4in Shepherd et al., 2018) the input–output method obtains a rate of Gt yr−1 for the AIS, while the mass balance rates fromsatellite gravimetry ( Gt yr−1) and from altimetry( Gt yr−1) agree much better with our result for the AIS(<81.5∘ S) between 2003 and 2010 of Gt yr−1.
Besides the peninsula, our comparison of mass changes from altimetry and fromGRACE at the EAIS (Fig. 11b) reveals some significantdifferences between the time series. For the time interval 2002 to 2016 (seeSect. S6.3), the mean rate at the EAIS from altimetry (9.6±6.9 Gt yr−1) is mainly dominated by the accumulation events in 2009and 2011. In contrast, the GRACE data imply an average mass gain of42.1 Gt yr−1 over this time interval. Especially after 2011, thedifferences become very prominent in the time series. The mass changes forthe individual basins (Fig. 12) reveal that this differencein the signals can be attributed to DML and Enderby Land. This might be asign for dynamic thickening. Here, all elevation changes have been convertedto mass using the density of surface firn. If a part of the positiveelevation changes in this region were indeed caused by ice dynamics, thiswould lead to an underestimation of mass gains from altimetry. The results ofthe Bayesian combined approach ofMartín-Español et al. (2017) also suggest asmall dynamic thickening in this region.Rignot et al. (2008) observed nosignificant mass changes in this region between 1992 and 2006 using theinput–output method.Gardner et al. (2018) compared present-day ice flowvelocities to measurements from 2008. They obtain a slightly reduced icedischarge in DML (which would support the hypothesis of a dynamicthickening), while they observe a small increase in discharge for EnderbyLand. Part of the discrepancy with the GRACE results could be also due touncertainties in the geophysical corrections applied to the GRACE data, suchas the effects of glacial isostatic adjustment. More work, similar to the IceSheet Mass Balance Inter-comparison Exercises(Shepherd et al., 2018), or thecombination of different types of observations as inMartín-Español et al. (2016), could help identify the reasons leading to thedisagreement.
In this paper we presented an approach to combine different satellitealtimetry missions, observation modes and techniques. The reprocessing of theconventional pulse-limited radar altimetry ensures that two fundamental stepsin processing of radar ice altimetry, the waveform retracking and the slopecorrection, are performed consistently. Furthermore, we showed that themethods used here improved the overall precision by 50 % over thestandard data sets available from ESA and NASA. The validation with in situand airborne measurements and the comparison with the IMAU-FDM shows thatinter-mission offsets have been successfully corrected and that theuncertainty estimates for our resulting monthly multi-mission SEC grids arerealistic.
We analyzed the resulting time series and found that they provide detailedinsight in the evolution of the surface elevation of the Antarctic Ice Sheet.From the combined SEC time series we calculated the long-term surfaceelevation change over the last 25 years. Observations from the Seasat andGeosat missions extend the time series in the coastal regions of EastAntarctica back to 1978. The unique data show that large parts of the EastAntarctic Plateau are very close to equilibrium, while changes over shortertime intervals identify interannual variations, which cannot be identified inlong-term trends and are mostly associated with snowfall anomalies.
The monthly mass time series show that the AIS (excluding the polar gapwithin 81.5∘ S) lost an average amount of mass of Gt yr−1 between 1992 and 2017 (equivalent to0.24±0.04 mm yr−1 of mean sea level change). These lossesaccelerated in several regions and, hence, for 2010–2017 we obtain Gt yr−1 (or0.38±0.07 mm yr−1) for thesame area. The comparison of the altimetry-derived mass changes, integratedover different basins and regions of the ice sheet, with SMBA and GRACE showshigh consistency of the different techniques. A correlation coefficientbetween the mass anomalies from altimetry and from GRACE of 0.96 (for thetime interval 2002–2016; see Table S4) indicates the excellent agreement ofthe observed interannual variations. The correlation with the SMBA (0.60 for1992–2016) is comparatively lower but still indicates a high agreement. Inthe APIS, differences between the mass time series of the differenttechniques arise mainly due to the poor spatial sampling of the altimetrydata, while for the EAIS, the remaining discrepancies to mass time seriesfrom GRACE might be explained by the density mask used or uncertainties inthe GRACE processing. These remaining issues and open questions should beaddressed in future work in order to further reduce the uncertainty of theestimates of the mass balance of the AIS. The recently launched laseraltimeter ICESat-2 promises a new milestone in ice sheet altimetry. Webelieve that our multi-mission combination approach can provide an importanttool for including the extremely high resolution of this mission into thelong-time observations of satellite altimetry spanning the past few decades.
Our resulting monthly 10 km × 10 km grids of SECwith respect to epoch 09/2010, accompanied by corresponding uncertainty estimates, are available fordownload athttps://doi.pangaea.de/10.1594/PANGAEA.897390(Schröder et al., 2019).
The supplement related to this article is available online at: https://doi.org/10.5194/tc-13-427-2019-supplement.
LS designed the study and developed the PLRAreprocessing, the repeat-altimetry processing and the time series generation.VH supplied the reprocessed CryoSat-2 SARIn data. SRML and MRvdB provided theRACMO and IMAU-FDM models. All authors discussed the results and contributedto the writing and editing of the manuscript.
Michiel van den Broeke is a member of the editorial boardof the journal. All other authors declare that they have no conflict ofinterest.
This work is supported by the Deutsche Bundesstiftung Umwelt (DBU, GermanFederal Environmental Foundation)) and the German Ministry of Economics and Technology (grant 50EE1331 to Veit Helm). We thank the European Space Agency, theNational Snow and Ice Data Center and the NASA Goddard Space Flight Centerfor providing the altimetry data products. We would especially like to thankJairo Santana for his support in accessing the GSFC data. We are very gratefulfor the comments from the anonymous referees, Andrew Shepherd and the editorEtienne Berthier, which significantly helped to improve and clarify the manuscript.We acknowledge support by the Open Access Publication Funds of the SLUB/TUDresden.
Edited by: Etienne Berthier
Reviewed by: three anonymous referees
Adusumilli, S., Fricker, H. A., Siegfried, M. R., Padman, L., Paolo, F. S., andLigtenberg, S. R. M.: Variable Basal Melt Rates of Antarctic Peninsula IceShelves, 1994–2016, Geophys. Res. Lett., 45, 4086–4095,https://doi.org/10.1002/2017gl076652, 2018. a
Arthern, R., Wingham, D., and Ridout, A.: Controls on ERS altimetermeasurements over ice sheets: Footprint-scale topography, backscatterfluctuations, and the dependence of microwave penetration depth on satelliteorientation, J. Geophys. Res.-Atmos., 106,33471–33484,https://doi.org/10.1029/2001JD000498, 2001. a,b
Baarda, W.: A testing procedure for use in geodetic networks, NetherlandsGeodetic Commission, Delft, the Netherlands, 1968. a
Bamber, J.: Ice Sheet Altimeter Processing Scheme, Int. J. Remote Sens.,14, 925–938,https://doi.org/10.1080/01431169408954125, 1994. a,b,c,d,e,f
Bamber, J. L., Gomez-Dans, J. L., and Griggs, J. A.: A new 1 km digitalelevation model of the Antarctic derived from combined satellite radar andlaser data – Part 1: Data and methods, The Cryosphere, 3, 101–111,https://doi.org/10.5194/tc-3-101-2009, 2009. a
Boening, C., Lebsock, M., Landerer, F., and Stephens, G.: Snowfall-driven masschange on the East Antarctic ice sheet, Geophys. Res. Lett., 39, L21501,https://doi.org/10.1029/2012GL053316, 2012. a,b
Brenner, A., Bindschadler, R., Zwally, H., and Thomas, R.: Slope-inducederrors in radar altimetry over continental ice sheets, J. Geophys. Res., 88,1617–1623,https://doi.org/10.1029/JC088iC03p01617, 1983. a
Brenner, A., DiMarzio, J., and Zwally, H.: Precision and Accuracy of SatelliteRadar and Laser Altimeter Data Over the Continental Ice Sheets, IEEE T.Geosci. Remote, 45, 321–331,https://doi.org/10.1109/TGRS.2006.887172, 2007. a
Brockley, D., Baker, S., Femenias, P., Martinez, B., Massmann, F.-H., Otten,M., Paul, F., Picard, B., Prandi, P., Roca, M., Rudenko, S., Scharroo, R.,and Visser, P.: REAPER: Reprocessing 12 Years of ERS-1 and ERS-2 Altimetersand Microwave Radiometer Data, IEEE T. Geosci. Remote, 55,5506–5514,https://doi.org/10.1109/TGRS.2017.2709343, 2017. a,b
Brunt, K. M., Hawley, R. L., Lutz, E. R., Studinger, M., Sonntag, J. G.,Hofton, M. A., Andrews, L. C., and Neumann, T. A.: Assessment of NASAairborne laser altimetry data using ground-based GPS data near SummitStation, Greenland, The Cryosphere, 11, 681–692,https://doi.org/10.5194/tc-11-681-2017, 2017. a
Brunt, K. M., Neumann, T. A., and Larsen, C. F.: Assessment of altimetryusing ground-based GPS data from the 88S Traverse, Antarctica, in support ofICESat-2, The Cryosphere Discuss.,https://doi.org/10.5194/tc-2018-160, inreview, 2018. a
Chuter, S., Martín-Español, A., Wouters, B., and Bamber, J.: Massbalance reassessment of glaciers draining into the Abbot and Getz Ice Shelvesof West Antarctica: Getz and Abbot Mass Balance Reassessment, Geophys. Res.Lett., 44, 7328–7337,https://doi.org/10.1002/2017GL073087, 2017. a,b
Davis, C.: A Combined Surface/volume Scattering Retracking Algorithm for IceSheet Satellite Altimetry, in: Geoscience and Remote Sensing Symposium,1992, IGARSS '92. International, Houston, TX, USA, 26–29 May 1992, vol. 2,969–971,https://doi.org/10.1109/IGARSS.1992.578311, 1992. a
Davis, C.: A robust threshold retracking algorithm for measuring ice-sheetsurface elevation change from satellite radar altimeters, IEEE T. Geosci.Remote, 35, 974–979,https://doi.org/10.1109/36.602540, 1997. a,b,c,d
Davis, C. and Ferguson, A.: Elevation change of the Antarctic ice sheet,1995–2000, from ERS-2 satellite radar altimetry, IEEE T. Geosci. Remote,42, 2437–2445,https://doi.org/10.1109/TGRS.2004.836789, 2004. a
Flament, T. and Rémy, F.: Dynamic thinning of Antarctic glaciers fromalong-track repeat radar altimetry, J. Glaciol., 58, 830–840,https://doi.org/10.3189/2012JoG11J118, 2012. a,b,c,d,e
Frappart, F., Legrésy, B., Niño, F., Blarel, F., Fuller, N., Fleury,S., Birol, F., and Calmant, S.: An ERS-2 altimetry reprocessing compatiblewith ENVISAT for long-term land and ice sheets studies, Remote Sens.Environ., 184, 558–581,https://doi.org/10.1016/j.rse.2016.07.037, 2016. a,b
Fricker, H. and Padman, L.: Thirty years of elevation change on AntarcticPeninsula ice shelves from multimission satellite radar altimetry, J.Geophys. Res., 117, C02026,https://doi.org/10.1029/2011JC007126, 2012. a,b
Fyke, J., Lenaerts, J. T. M., and Wang, H.: Basin-scale heterogeneity inAntarctic precipitation and its impact on surface mass variability, TheCryosphere, 11, 2595–2609,https://doi.org/10.5194/tc-11-2595-2017, 2017. a
Gardner, A. S., Moholdt, G., Scambos, T., Fahnstock, M., Ligtenberg, S., vanden Broeke, M., and Nilsson, J.: Increased West Antarctic and unchanged EastAntarctic ice discharge over the last 7 years, The Cryosphere, 12, 521–547,https://doi.org/10.5194/tc-12-521-2018, 2018. a,b
Groh, A. and Horwath, M.: The method of tailored sensitivity kernels forGRACE mass change estimates, General Assembly EGU, Vienna, Austria,17–22 April 2016, Geophys. Res. Abstr., 18, EGU2016–12065, 2016. a
Groh, A., Ewert, H., Scheinert, M., Fritsche, M., Rülke, A., Richter, A.,Rosenau, R., and Dietrich, R.: An Investigation of Glacial IsostaticAdjustment over the Amundsen Sea sector, West Antarctica, Global Planet.Change, 98–99, 45–53,https://doi.org/10.1016/j.gloplacha.2012.08.001, 2012. a
Helm, V., Humbert, A., and Miller, H.: Elevation and elevation change ofGreenland and Antarctica derived from CryoSat-2, The Cryosphere, 8,1539–1559,https://doi.org/10.5194/tc-8-1539-2014, 2014. a,b,c,d,e,f
Hogg, A., Shepherd, A., Cornford, S., Briggs, K., Gourmelen, N., Graham, J.,Joughin, I., Mouginot, J., Nagler, T., Payne, A., Rignot, E., and Wuite, J.:Increased ice flow in Western Palmer Land linked to ocean melting, Geophys.Res. Lett., 44, 4159–4167,https://doi.org/10.1002/2016GL072110, 2017. a
Horwath, M. and Dietrich, R.: Signal and error in mass change inferences fromGRACE: the case of Antarctica, Geophys. J. Int., 177, 849–864,https://doi.org/10.1111/j.1365-246X.2009.04139.x, 2009. a
Horwath, M., Legrésy, B., Rémy, F., Blarel, F., and Lemoine, J.-M.:Consistent patterns of Antarctic ice sheet interannual variations fromENVISAT radar altimetry and GRACE satellite gravimetry, Geophys. J. Int.,189, 863–876,https://doi.org/10.1111/j.1365-246X.2012.05401.x, 2012. a,b
Hurkmans, R., Bamber, J., Sørensen, L., Joughin, I., Davis, C., and Krabill,W.: Spatiotemporal interpolation of elevation changes derived from satellitealtimetry for Jakobshavn Isbræ, Greenland, J. Geophys. Res., 117, F03001,https://doi.org/10.1029/2011JF002072, 2012. a
Ivins, E., James, T., Wahr, J., Schrama, E., Landerer, F., and Simon, K.:Antarctic contribution to sea level rise observed by GRACE with improved GIAcorrection, J. Geophys. Res.-Sol. Ea., 118, 3126–3141,https://doi.org/10.1002/jgrb.50208, 2013. a
Joughin, I., Shean, D., Smith, B., and Dutrieux, P.: Grounding linevariability and subglacial lake drainage on Pine Island Glacier, Antarctica,Geophys. Res. Lett., 43, 9093–9102,https://doi.org/10.1002/2016GL070259, 2016. a
Kallenberg, B., Tregoning, P., Hoffmann, J. F., Hawkins, R., Purcell, A., andAllgeyer, S.: A new approach to estimate ice dynamic rates using satelliteobservations in East Antarctica, The Cryosphere, 11, 1235–1245,https://doi.org/10.5194/tc-11-1235-2017, 2017. a
Khvorostovsky, K.: Merging and Analysis of Elevation Time Series OverGreenland Ice Sheet From Satellite Radar Altimetry, IEEE T. Geosci.Remote, 50, 23–36,https://doi.org/10.1109/TGRS.2011.2160071, 2012. a,b
Konrad, H., Gilbert, L., Cornford, S., Payne, A., Hogg, A., Muir, A., andShepherd, A.: Uneven onset and pace of ice-dynamical imbalance in theAmundsen Sea Embayment, West Antarctica, Geophys. Res. Lett., 44, 910–918,https://doi.org/10.1002/2016GL070733, 2016. a,b
Lacroix, P., Dechambre, M., Legrésy, B., Blarel, F., and Rémy, F.: Onthe use of the dual-frequency ENVISAT altimeter to determine snowpackproperties of the Antarctic ice sheet, Remote Sens. Environ., 112,1712–1729,https://doi.org/10.1016/j.rse.2007.08.022, 2008. a
Legrésy, B. and Rémy, F.: Altimetric observations of surfacecharacteristics of the Antarctic ice sheet, J. Glaciol., 43, 265–276,https://doi.org/10.3189/S002214300000321X, 1997. a
Legrésy, B., Rémy, F., and Schaeffer, P.: Different ERS altimetermeasurements between ascending and descending tracks caused by wind inducedfeatures over ice sheets, Geophys. Res. Lett., 26, 2231–2234,https://doi.org/10.1029/1999GL900531, 1999. a,b
Legrésy, B., Papa, F., Rémy, F., Vinay, G., van den Bosch, M., andZanife, O.-Z.: ENVISAT radar altimeter measurements over continentalsurfaces and ice caps using the ICE-2 retracking algorithm, Remote Sens.Environ., 85, 150–163,https://doi.org/10.1016/j.rse.2004.11.018, 2005. a
Legrésy, B., Rémy, F., and Blarel, F.: Along track repeat altimetryfor ice sheets and continental surface studies, in: Proc. Symposium on15 years of Progress in Radar Altimetry, Venice, Italy, 13–18 March 2006,European Space Agency Publication Division, Noordwijk, the Netherlands,eSA-SP No. 614, paper No. 181, 2006. a
Lenaerts, J., van Meijgaard, E., van den Broeke, M., Ligtenberg, S., Horwath,M., and Isaksson, E.: Recent snowfall anomalies in Dronning Maud Land, EastAntarctica, in a historical and future climate perspective, Geophys. Res.Lett., 40, 2684–2688,https://doi.org/10.1002/grl.50559, 2013. a,b,c,d
Li, X., Rignot, E., Morlighem, M., Mouginot, J., and Scheuchl, B.: Groundingline retreat of Totten Glacier, East Antarctica, 1996 to 2013, Geophys. Res.Lett., 42, 8049–8056,https://doi.org/10.1002/2015GL065701, 2015. a
Li, X., Rignot, E., Mouginot, J., and Scheuchl, B.: Ice flow dynamics and massloss of Totten Glacier, East Antarctica, from 1989 to 2015, Geophys. Res.Lett., 43, 6366–6373,https://doi.org/10.1002/2016GL069173, 2016. a,b
Li, Y. and Davis, C.: Decadal Mass Balance of the Greenland and Antarctic IceSheets from High Resolution Elevation Change Analysis of ERS-2 and EnvisatRadar Altimetry Measurements, in: IEEE International Geoscience & RemoteSensing Symposium, IGARSS 2008, 8–11 July 2008, Boston, Massachusetts, USA,Proceedings, IEEE, 339–342,https://doi.org/10.1109/IGARSS.2008.4779727, 2008. a
Ligtenberg, S. R. M., Helsen, M. M., and van den Broeke, M. R.: An improvedsemi-empirical model for the densification of Antarctic firn, The Cryosphere,5, 809–819,https://doi.org/10.5194/tc-5-809-2011, 2011. a,b,c
Martin, T., Zwally, H., Brenner, A., and Bindschadler, R.: Analysis andretracking of continental ice sheet radar altimeter waveforms, J. Geophys.Res., 88, 1608,https://doi.org/10.1029/JC088iC03p01608, 1983. a
Martín-Español, A., Zammit-Mangion, A., Clarke, P., Flament, T., Helm,V., King, M., Luthcke, S., Petrie, E., Rémy, F., Schön, N., Wouters,B., and Bamber, J.: Spatial and temporal Antarctic Ice Sheet mass trends,glacio-isostatic adjustment and surface processes from a joint inversion ofsatellite altimeter, gravity and GPS data, J. Geophys. Res.-Earth,121, 182–200,https://doi.org/10.1002/2015JF003550, 2016. a
Martín-Español, A., Bamber, J., and Zammit-Mangion, A.: Constrainingthe mass balance of East Antarctica, Geophys. Res. Lett., 44, 4168–4175,https://doi.org/10.1002/2017GL072937, 2017. a
McMillan, M., Shepherd, A., Sundal, A., Briggs, K., Muir, A., Ridout, A., Hogg,A., and Wingham, D.: Increased ice losses from Antarctica detected byCryoSat-2, Geophys. Res. Lett., 41, 3899–3905,https://doi.org/10.1002/2014GL060111,2014. a,b,c,d
McMillan, M., Leeson, A., Shepherd, A., Briggs, K., Armitage, T., Hogg, A.,Kuipers Munneke, P., van den Broeke, M., Noël, B., van de Berg, W.,Ligtenberg, S., Horwath, M., Groh, A., Muir, A., and Gilbert, L.: Ahigh-resolution record of Greenland mass balance, Geophys. Res. Lett., 43,7002–7010,https://doi.org/10.1002/2016GL069666, 2016. a
Mémin, A., Flament, T., Alizier, B., Watson, C., and Rémy, F.:Interannual variation of the Antarctic Ice Sheet from a combined analysis ofsatellite gravimetry and altimetry data, Earth Planet. Sc. Lett., 422,150–156,https://doi.org/10.1016/j.epsl.2015.03.045, 2015. a,b
Michel, A., Flament, T., and Rémy, F.: Study of the Penetration Bias ofENVISAT Altimeter Observations over Antarctica in Comparison toICESat Observations, Remote Sensing, 6, 9412–9434,https://doi.org/10.3390/rs6109412,2014. a
Miles, B. W. J., Stokes, C. R., and Jamieson, S. S. R.: Velocity increases atCook Glacier, East Antarctica, linked to ice shelf loss and a subglacialflood event, The Cryosphere, 12, 3123–3136,https://doi.org/10.5194/tc-12-3123-2018, 2018. a
Mouginot, J., Rignot, E., and Scheuchl, B.: Sustained increase in icedischarge from the Amundsen Sea Embayment, West Antarctica, from 1973 to2013, Geophys. Res. Lett., 41, 1576–1584,https://doi.org/10.1002/2013GL059069, 2014. a
Nilsson, J., Vallelonga, P., Simonsen, S., Sørensen, L., Forsberg, R.,Dahl-Jensen, D., Hirabayashi, M., Goto-Azuma, K., Hvidberg, C., Kjaer, H.,and Satow, K.: Greenland 2012 melt event effects on CryoSat-2 radaraltimetry, Geophys. Res. Lett., 42, 3919–3926,https://doi.org/10.1002/2015GL063296,2015. a
Nilsson, J., Gardner, A., Sandberg Sørensen, L., and Forsberg, R.:Improved retrieval of land ice topography from CryoSat-2 data and its impactfor volume-change estimation of the Greenland Ice Sheet, The Cryosphere, 10,2953–2969,https://doi.org/10.5194/tc-10-2953-2016, 2016. a,b,c
Paolo, F., Fricker, H., and Padman, L.: Constructing improved decadal recordsof Antarctic ice shelf height change from multiple satellite radaraltimeters, Remote Sens. Environ., 177, 192–205,https://doi.org/10.1016/j.rse.2016.01.026, 2016. a,b,c
Pritchard, H., Arthern, R., Vaughan, D., and Edwards, L.: Extensive dynamicthinning on the margins of the Greenland and Antarctic ice sheets, Nature,461, 971–975,https://doi.org/10.1038/nature08471, 2009. a
Rémy, F. and Parouty, S.: Antarctic Ice Sheet and Radar Altimetry: AReview, Remote Sensing, 1, 1212–1239,https://doi.org/10.3390/rs1041212, 2009. a,b
Richter, A., Popov, S., Dietrich, R., Lukin, V., Fritsche, M., Lipenkov, V.,Matveev, A., Wendt, J., Yuskevich, A., and Masolov, V.: Observationalevidence on the stability of the hydro-glaciological regime of subglacialLake Vostok, Geophys. Res. Lett., 35, L11502,https://doi.org/10.1029/2008GL033397,2008. a
Richter, A., Popov, S., Fritsche, M., Lukin, V., Matveev, A., Ekaykin, A.,Lipenkov, V., Fedorov, D., Eberlein, L., Schröder, L., Ewert, H.,Horwath, M., and Dietrich, R.: Height changes over subglacial Lake Vostok,East Antarctica: Insights from GNSS observations, J. Geophys. Res.-Earth,119, 2460–2480,https://doi.org/10.1002/2014JF003228, 2014. a,b
Richter, A., Horwath, M., and Dietrich, R.: Comment on Zwally and others(2015)-Mass gains of the Antarctic ice sheet exceed losses, J. Glaciol., 62,604–606,https://doi.org/10.1017/jog.2016.60, 2016. a
Rignot, E.: Changes in ice dynamics and mass balance of the Antarctic icesheet, Philos. T. Roy. Soc. A, 364, 1637–1655,https://doi.org/10.1098/rsta.2006.1793, 2006. a
Rignot, E., Bamber, J., van den Broeke, M., Davis, C., Li, Y., van de Berg, W.,and van Meijgaard, E.: Recent Antarctic ice mass loss from radarinterferometry and regional climate modelling, Nat. Geosci., 1, 106–110,https://doi.org/10.1038/ngeo102, 2008. a
Rignot, E., Mouginot, J., and Scheuchl, B.: Ice Flow of the Antarctic IceSheet, Science, 333, 1427–1430,https://doi.org/10.1126/science.1208336, 2011. a
Rignot, E., Mouginot, J., Morlighem, M., Seroussi, H., and Scheuchl, B.:Widespread, rapid grounding line retreat of Pine Island, Thwaites, Smith,and Kohler glaciers, West Antarctica, from 1992 to 2011, Geophys. Res.Lett., 41, 3502–3509,https://doi.org/10.1002/2014GL060140, 2014. a
Rignot, E., Mouginot, J., and Scheuchl, B.: MEaSUREs InSAR-Based AntarcticaIce Velocity Map, Version 2, Boulder, Colorado USA, NASA National Snow andIce Data Center Distributed Active Archive Center,https://doi.org/10.5067/D7GK8F5J8M8R, 2017. a
Roemer, S., Legrésy, B., Horwath, M., and Dietrich, R.: Refined analysisof radar altimetry data applied to the region of the subglacial LakeVostok/Antarctica, Remote Sens. Environ., 106, 269–284,https://doi.org/10.1016/j.rse.2006.02.026, 2007. a,b
Scambos, T. and Shuman, C.: Comment on “Mass gains of theAntarctic ice sheet exceed losses” by H. J. Zwally andothers, J. Glaciol., 62, 599–603,https://doi.org/10.1017/jog.2016.59, 2016. a
Schröder, L., Richter, A., Fedorov, D., Eberlein, L., Brovkov, E., Popov,S. V., Knöfel, C., Horwath, M., Dietrich, R., Matveev, A. Y., Scheinert,M., Lukin, Valeriy. V. : Kinematic GNSS profiles in central East Antarctica,PANGAEA,https://doi.org/10.1594/PANGAEA.869761, 2016. a
Schröder, L., Richter, A., Fedorov, D. V., Eberlein, L., Brovkov, E. V.,Popov, S. V., Knöfel, C., Horwath, M., Dietrich, R., Matveev, A. Y.,Scheinert, M., and Lukin, V. V.: Validation of satellite altimetry bykinematic GNSS in central East Antarctica, The Cryosphere, 11, 1111–1130,https://doi.org/10.5194/tc-11-1111-2017, 2017. a,b,c,d,e
Schröder, L., Horwath, M., Dietrich, R., Helm, V., van den Broeke, M. R.,Ligtenberg, S. R. M.: Gridded surface elevation changes from multi-missionsatellite altimetry 1978–2017, PANGAEA,https://doi.org/10.1594/PANGAEA.897390, 2019. a,b
Scott, R., Baker, S., Birkett, C., Cudlip, W., Laxon, S., Mantripp, D.,Mansley, J., Morley, J., Rapley, C., Ridley, J., Strawbridge, F., andWingham, D.: A comparison of the performance of the ice and ocean trackingmodes of the ERS-1 radar altimeter over non-ocean surfaces, Geophys. Res.Lett., 21, 553–556,https://doi.org/10.1029/94GL00178, 1994. a
Shepherd, A., Ivins, E., A, G., Barletta, V., Bentley, M., Bettadpur, S.,Briggs, K., Bromwich, D., Forsberg, R., Galin, N., Horwath, M., Jacobs, S.,Joughin, I., King, M., Lenaerts, J., Li, J., Ligtenberg, S., Luckman, A.,Luthcke, S., McMillan, M., Meister, R., Milne, G., Mouginot, J., Muir, A.,Nicolas, J., Paden, J., Payne, A., Pritchard, H., Rignot, E., Rott, H.,Sorensen, L., Scambos, T., Scheuchl, B., Schrama, E., Smith, B., Sundal, A.,van Angelen, J., van de Berg, W., van den Broeke, M., Vaughan, D., Velicogna,I., Wahr, J., Whitehouse, P., Wingham, D., Yi, D., Young, D., and Zwally, H.:A Reconciled Estimate of Ice-Sheet Mass Balance, Science, 338, 1183–1189,https://doi.org/10.1126/science.1228102, 2012. a,b,c
Shepherd, A., Ivins, E., Rignot, E., Smith, B., van den Broeke, M., Velicogna,I., Whitehouse, P., Briggs, K., Joughin, I., Krinner, G., Nowicki, S., Payne,T., Scambos, T., Schlegel, N., A, G., Agosta, C., Ahlstrøm, A., Babonis,G., Barletta, V., Blazquez, A., Bonin, J., Csatho, B., Cullather, R.,Felikson, D., Fettweis, X., Forsberg, R., Gallee, H., Gardner, A., Gilbert,L., Groh, A., Gunter, B., Hanna, E., Harig, C., Helm, V., Horvath, A.,Horwath, M., Khan, S., Kjeldsen, K., Konrad, H., Langen, P., Lecavalier, B.,Loomis, B., Luthcke, S., McMillan, M., Melini, D., Mernild, S., Mohajerani,Y., Moore, P., Mouginot, J., Moyano, G., Muir, A., Nagler, T., Nield, G.,Nilsson, J., Noel, B., Otosaka, I., Pattle, M., Peltier, W., Pie, N.,Rietbroek, R., Rott, H., Sandberg-Sørensen, L., Sasgen, I., Save, H.,Scheuchl, B., Schrama, E., Schröder, L., Seo, K.-W., Simonsen, S.,Slater, T., Spada, G., Sutterley, T., Talpe, M., Tarasov, L., van de Berg,W., van der Wal, W., van Wessem, M., Vishwakarma, B., Wiese, D., and Wouters,B.: Mass balance of the Antarctic Ice Sheet from 1992 to 2017, Nature, 558,219–222,https://doi.org/10.1038/s41586-018-0179-y, 2018. a,b,c,d,e,f
Simonsen, S. and Sørensen, L.: Implications of changing scatteringproperties on Greenland ice sheet volume change from Cryosat-2 altimetry,Remote Sens. Environ., 190, 207–216,https://doi.org/10.1016/j.rse.2016.12.012, 2017. a,b
Studinger, M.: IceBridge ATM L4 Surface Elevation Rate of Change, Version 1.Updated 2017, Boulder, Colorado USA. NASA National Snow and Ice DataCenter Distributed Active Archive Center,https://doi.org/10.5067/BCW6CI3TXOCY, 2014. a,b
Thomas, E., Hosking, J., Tuckwell, R., Warren, R., and Ludlow, E.: Twentiethcentury increase in snowfall in coastal West Antarctica, Geophys. Res.Lett., 42, 9387–9393,https://doi.org/10.1002/2015GL065750, 2015. a
Thomas, R., Davis, C., Frederick, E., Krabill, W., Li, Y., Manizade, S., andMartin, C.: A comparison of Greenland ice-sheet volume changes derived fromaltimetry measurements, J. Glaciol., 54, 203–212,https://doi.org/10.3189/002214308784886225, 2008. a,b,c
van Wessem, J. M., van de Berg, W. J., Noël, B. P. Y., van Meijgaard, E.,Amory, C., Birnbaum, G., Jakobs, C. L., Krüger, K., Lenaerts, J. T. M.,Lhermitte, S., Ligtenberg, S. R. M., Medley, B., Reijmer, C. H., van Tricht,K., Trusel, L. D., van Ulft, L. H., Wouters, B., Wuite, J., and van denBroeke, M. R.: Modelling the climate and surface mass balance of polar icesheets using RACMO2 – Part 2: Antarctica (1979–2016), The Cryosphere, 12,1479–1498,https://doi.org/10.5194/tc-12-1479-2018, 2018. a,b,c
Velicogna, I., Sutterley, T., and van den Broeke, M.: Regional acceleration inice mass loss from Greenland and Antarctica using GRACE time-variable gravitydata, Geophys. Res. Lett., 41, 8130–8137,https://doi.org/10.1002/2014GL061052, 2014. a
Wingham, D., Rapley, C., and Griffiths, H.: New techniques in satellitealtimeter tracking systems, in: ESA Proceedings of the 1986 InternationalGeoscience and Remote Sensing Symposium (IGARSS'86) on RemoteSensing: Today's Solutions for Tomorrow'sInformation Needs, Zürich, Switzerland, 8–11 September 1986, vol. 3, 1339–1344, 1986. a
Wingham, D., Ridout, A., Scharroo, R., Arthern, R., and Shum, C.: AntarcticElevation Change from 1992 to 1996, Science, 282, 456–458,https://doi.org/10.1126/science.282.5388.456, 1998. a,b
Wingham, D., Francis, C., Baker, S., Bouzinac, C., Brockley, D., Cullen, R.,Chateau-Thierry, P. d., Laxon, S., Mallow, U., Mavrocordatos, C., Phalippou,L., Ratier, G., Rey, L., Rostan, F., Viau, P., and Wallis, D.: CryoSat: Amission to determine the fluctuations in Earth's land andmarine ice fields, Adv. Space Res., 37, 841–871,https://doi.org/10.1016/j.asr.2005.07.027, 2006a. a
Wingham, D., Shepherd, A., Muir, A., and Marshall, G.: Mass balance of theAntarctic ice sheet, Philos. T. Roy. Soc. A, 364, 1627–1635,https://doi.org/10.1098/rsta.2006.1792, 2006b. a,b,c,d
Wouters, B., Bamber, J., van den Broeke, M., Lenaerts, J., and Sasgen, I.:Limits in detecting acceleration of ice sheet mass loss due to climatevariability, Nat. Geosci., 6, 613–616,https://doi.org/10.1038/ngeo1874, 2013. a
Wouters, B., Martín-Español, A., Helm, V., Flament, T., van Wessem, J.,Ligtenberg, S., van den Broeke, M., and Bamber, J.: Dynamic thinning ofglaciers on the Southern Antarctic Peninsula, Science, 348, 899–903,https://doi.org/10.1126/science.aaa5727, 2015. a,b
Zwally, H., Giovinetto, M., Li, J., Cornejo, H., Beckley, M., Brenner, A.,Saba, J., and Yi, D.: Mass changes of the Greenland and Antarctic ice sheetsand shelves and contributions to sea-level rise: 1992-2002, J. Glaciol., 51,509–527,https://doi.org/10.3189/172756505781829007, 2005. a,b,c
Zwally, H., Li, J., Robbins, J., Saba, J., Yi, D., and Brenner, A.: Mass gainsof the Antarctic ice sheet exceed losses, J. Glaciol., 61, 1019–1936,https://doi.org/10.3189/2015JoG15J071, 2015. a,b,c,d,e